Modelling and numerical methods for identifying low-level adulteration in ground beef using near-infrared hyperspectral imaging (nir-hsi)
Talanta(2024)
Abstract
Owing to the inherent characteristics of ground beef, adulteration presents a substantial risk for suppliers and consumers alike. This study developed a robust and novel method for identifying replacement fraud in ground beef with beef liver, beef heart, and pork using Near Infrared-Hyperspectral Imaging (NIR-HSI) coupled with chemometric and other statistical methods. More specifically, NIR-HSI provided an efficient and accurate means of identifying each type of adulteration using the classification model Genetic Algorithm (GA) - Backpropagation Artificial Neural Network (BPANN), showing perfect sensitivity and specificity (a value of 1.00) for the calibration and the validation sets for all types of adulteration. As an alternative to chemometric analysis, Hyperspectral Imaging-Root Mean Square (HSI-RMS) value, based on the RMScut-off calculation, was determined to discriminate types of adulterations without the need of resource-intensive modelling. This HSI-RMS approach provides a simple-to-use method that avoids the complexity of HSI data processing and aims to directly understand the similarity between different spectra of one sample in the pixel level. Different types of adulteration show noticeable differences reflected in the HSI-RMS value (varying from 55-1439), which demonstrate the potential of HSI-RMS concept as a novel and valuable alternative for assessing the HSI data and facilitating the identification of adulterants.
MoreTranslated text
Key words
Hyperspectral imaging,Adulteration,Chemometrics,Statistics,Ground beef
AI Read Science
Must-Reading Tree
Example
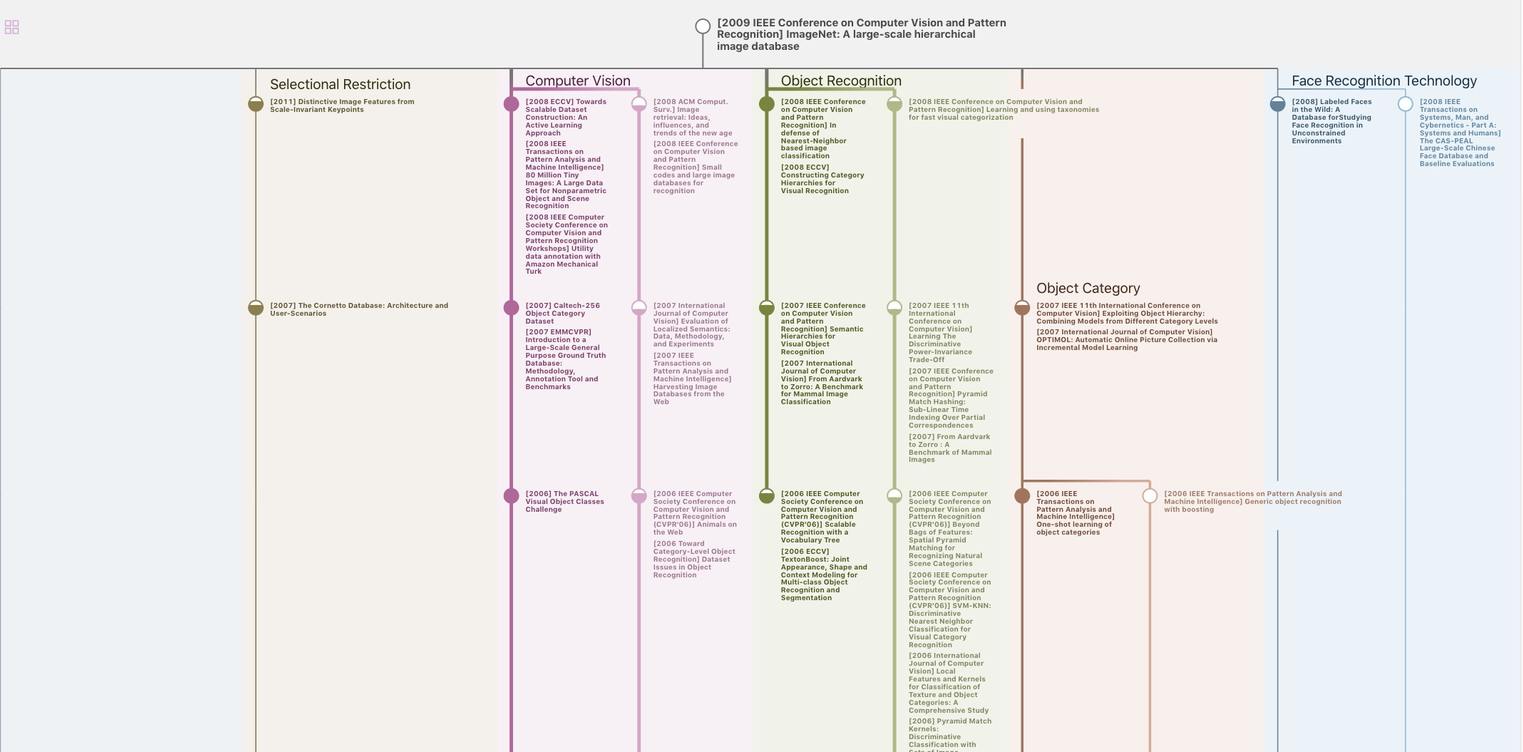
Generate MRT to find the research sequence of this paper
Chat Paper
Summary is being generated by the instructions you defined