MARLow: A Joint Multiplanar Autoregressive and Low-Rank Approach for Image Completion
COMPUTER VISION - ECCV 2016, PT VII(2016)
摘要
In this paper, we propose a novel multiplanar autoregressive (AR) model to exploit the correlation in cross-dimensional planes of a similar patch group collected in an image, which has long been neglected by previous AR models. On that basis, we then present a joint multiplanar AR and low-rank based approach (MARLow) for image completion from random sampling, which exploits the nonlocal self-similarity within natural images more effectively. Specifically, the multiplanar AR model constraints the local stationarity in different cross-sections of the patch group, while the low-rank minimization captures the intrinsic coherence of nonlocal patches. The proposed approach can be readily extended to multichannel images (e.g. color images), by simultaneously considering the correlation in different channels. Experimental results demonstrate that the proposed approach significantly outperforms state-of-the-art methods, even if the pixel missing rate is as high as 90%.
更多查看译文
关键词
Image completion,Multiplanar autoregressive model,Low-rank minimization
AI 理解论文
溯源树
样例
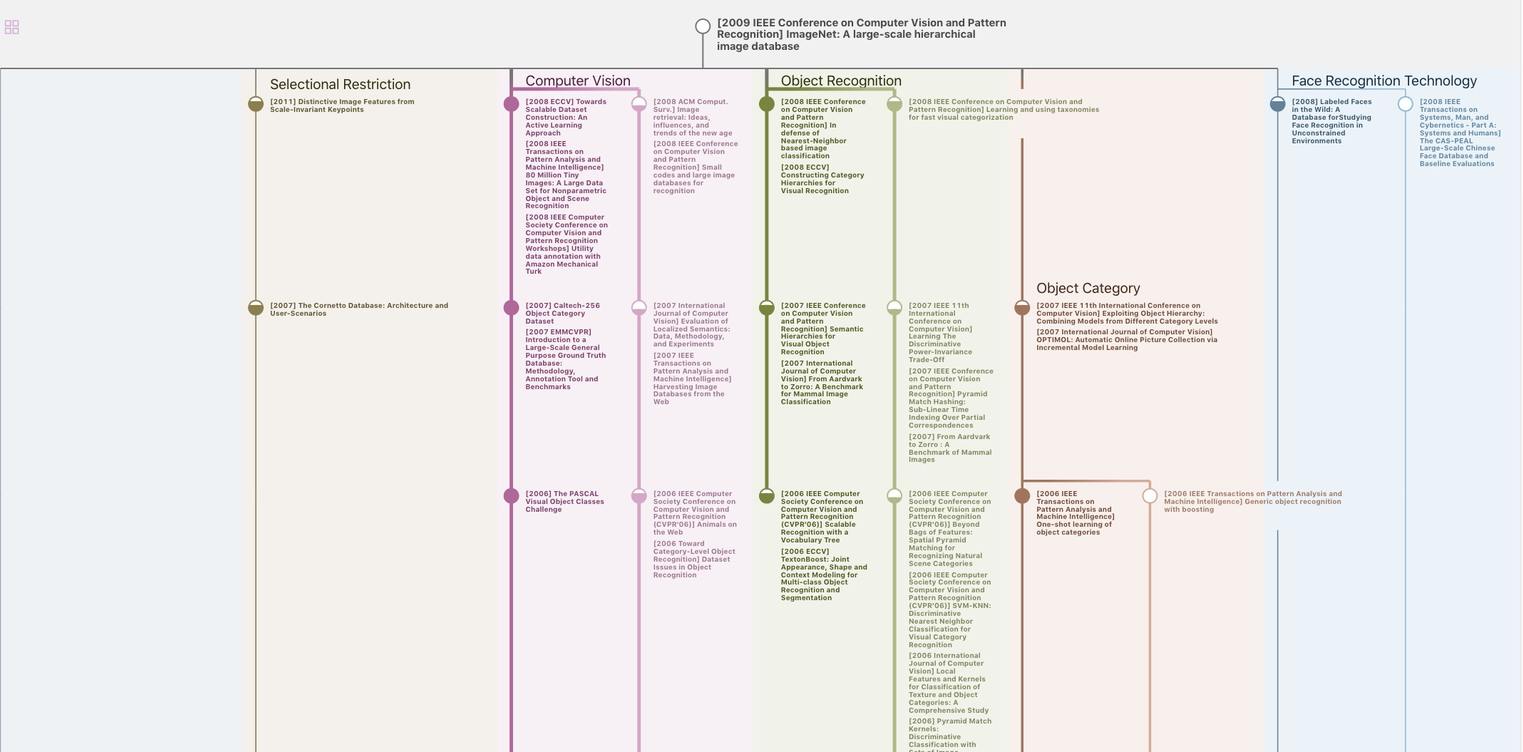
生成溯源树,研究论文发展脉络
Chat Paper
正在生成论文摘要