Abstract PO2-07-05: Deep learning model for automated quantification of HER2 expression in invasive breast cancers from immunohistochemical whole slide images
Cancer Research(2024)
摘要
Abstract Introduction Human epidermal growth factor receptor 2 (HER2) protein overexpression and/or HER2 gene amplification is found in about 20% of invasive breast cancers. Considering the results of the DESTINY-Breast trials confirming the remarkable efficacy of anti-HER2 antibody drug-conjugate (T-Dxd) in both HER2-overexpressed and HER2-low tumors, it is necessary to identify not only HER2 (immunohistochemistry (IHC) score 3+) overexpressing tumors, but also HER2-low tumors. The latter category, defined as IHC1+ or IHC2+ but non-amplified, has proven to be challenging even for experienced pathologists, with high inter-observer variability. Here, we validate the performance of a deep learning (DL) model at: 1) predicting the IHC score from IHC histological features and 2) identifying HER2-low tumors with high sensitivity. Methods 675 HER2 stained IHC slides from primary breast cancer patients were selected based on pathology reports across three different cohorts (KCL_GSTT_1 n=369; Cypath_Breast n=214; KCL_GSTT_2 n=92). The slides were digitized and reviewed by expert breast pathologists. Specifically, Cypath_Breast and KCL_GSTT_2 were each annotated by one expert pathologist, while KCL_GSTT_1 was annotated by 5 expert pathologists with the ground truth defined as a majority vote between the 5 annotators. Cypath_Breast was assigned as the “discovery” set; while KCL_GSTT_1 and KCL_GSTT_2 were used as two independent validation cohorts. The model was specifically trained to extract features from IHC tiles to ensure they would capture both the staining intensity and the staining location on the cells (membrane, cytoplasmic or nuclei). The one-vs-one (OVO) and one-vs-rest (OVR) AUC as well as sensitivity and specificity to HER2-low and positive tumors were used as metrics to assess the performance of our model. An expert pathologist reviewed the most predictive regions of HER2 expression. Results After review by expert pathologists, 59 slides from KCL_GSTT_1 were removed either because of folded tissue, blurriness or because there was too little tissue. The IHC scores (0/1+/2+/3+) for the 3 cohorts were distributed as follows; Cypath_Breast: 42/120/36/16; KCL_GSTT_1: 120/90/52/48; KCL_GSTT_2: 54/22/15/1. For KCL_GSTT_1, the average agreement between the 5 expert pathologists amounted to a Cohen’s Kappa score of 0.63. Following training on Cypath_Breast, the performance of the model are presented in An ablation study proved that a feature extractor trained on IHC tiles outperformed an in-house extractor solely trained on H&E tiles (OVO AUC 0.95 vs 0.93 on KCL_GSTT_1; DeLong p< 0.001). Specifically, the model performed best at distinguishing HER2-negative (HER2 IHC 0) from HER2-low and HER2-positive tumors with a sensitivity (0 vs 1+/2+/3+) of 0.95 [0.93 - 0.98] and a specificity (0 vs 1+/2+/3+) of 0.78 [0.72 - 0.84] on KCL_GSTT_1. We observed the same tendency on KCL_GSTT_2 with a sensitivity (0 vs 1+/2+/3+) of 0.97 [0.91 - 1.00] and a specificity of 0.91 [0.83 - 0.98]. More importantly, the DL model reached a Cohen’s Kappa score of 0.72 [0.67 - 0.77] with the ground truth on KCL_GSTT_1, surpassing the average agreement Kappa score between expert pathologists (0.63). The most predictive regions showed cytoplasmic staining in tumor regions. Conclusion Our model provides a path towards a fully automated workflow for identifying up to 95% of breast cancer patients who could potentially benefit from anti-HER2 targeted therapies. Additionally, we show the utility of AI-based tools to minimize discrepancies among pathologists and assist in the diagnostic patient pathway. Table 1: Results of external validation on KCL_GSTT_1 and KCL_GSTT_2 <grpahic> Cohorts OVO AUC OVR AUC IHC 0 OVR AUC IHC 1+ OVR AUC IHC 2+ OVR AUC IHC 3+ KCL_GSTT_1 0.95 0.95 0.80 0.91 0.99 [0.93 - 0.96] [0.94 - 0.95] [0.79 - 0.82] [0.90 - 0.92] [0.99 - 1.00] KCL_GSTT_2 0.92 0.97 0.78 0.84 N/A [0.90 - 0.93] [0.96 - 0.98] [0.76 - 0.81] [0.80 - 0.88] Citation Format: Pierre-Antoine Bannier, Loïc Herpin, Rémy Dubois, Lydwine Van Praet, Charles Maussion, Ellen Amonoo, Anca Mera, Jasmine Timbres, Cheryl Gillett, Elinor Sawyer, Patrycja Gazinska, Piotr Ziolkowski, Roberto Salgado, Sheeba Irshad. Deep learning model for automated quantification of HER2 expression in invasive breast cancers from immunohistochemical whole slide images [abstract]. In: Proceedings of the 2023 San Antonio Breast Cancer Symposium; 2023 Dec 5-9; San Antonio, TX. Philadelphia (PA): AACR; Cancer Res 2024;84(9 Suppl):Abstract nr PO2-07-05.
更多查看译文
AI 理解论文
溯源树
样例
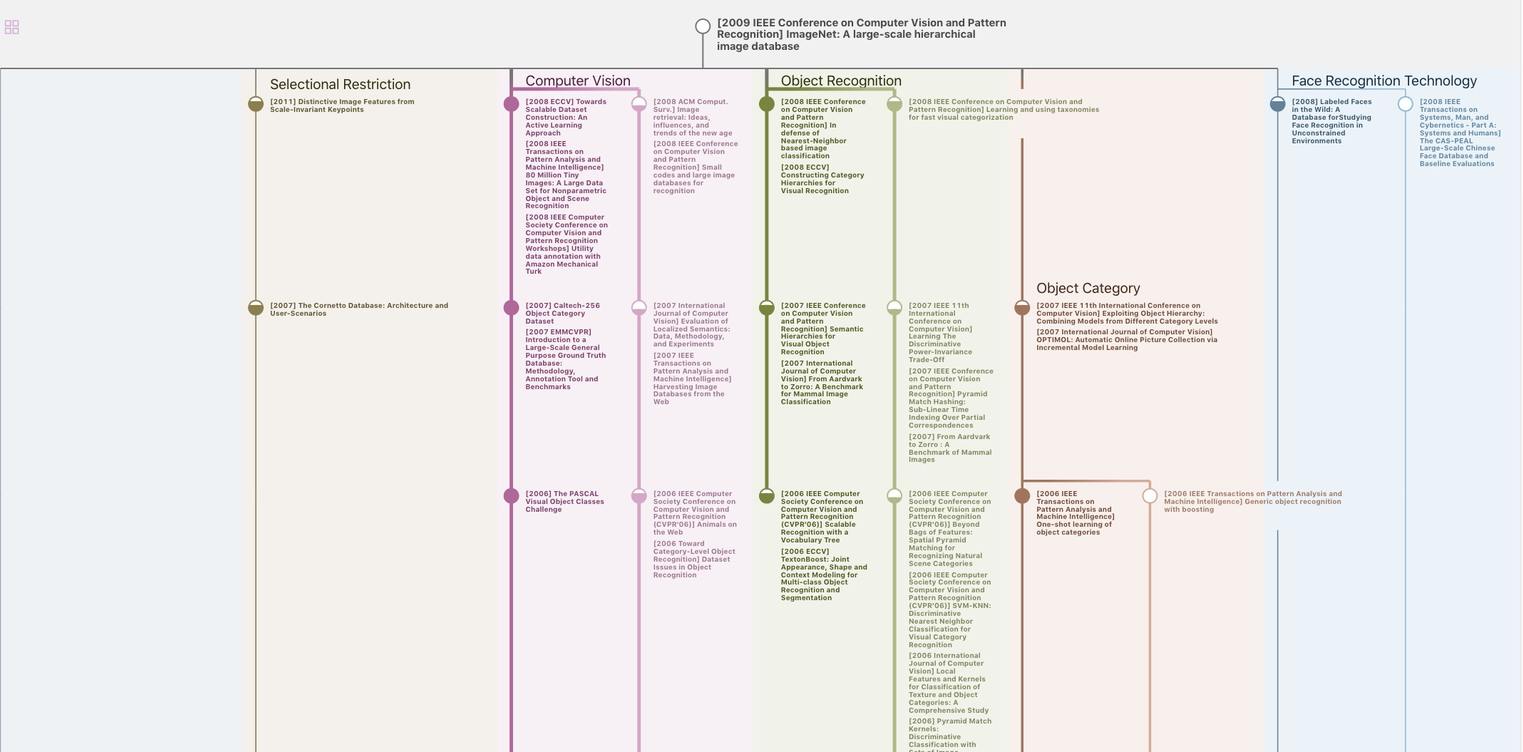
生成溯源树,研究论文发展脉络
Chat Paper
正在生成论文摘要