Abstract PO4-07-03: Machine Learning Breast Cancer Risk Prediction Using Sequential Past Mammograms - a Pilot Study
Cancer Research(2024)
AI Read Science
Must-Reading Tree
Example
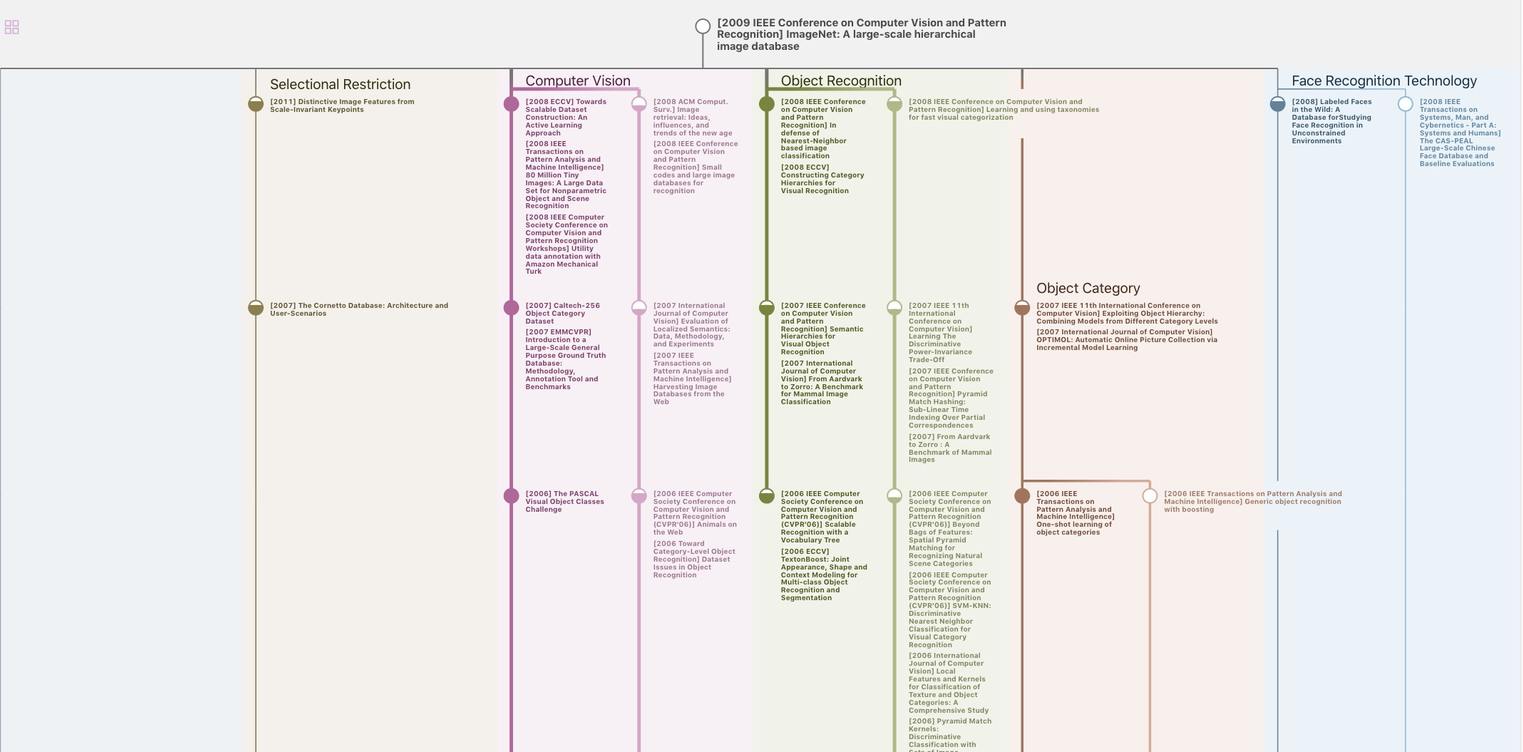
Generate MRT to find the research sequence of this paper
Chat Paper
Summary is being generated by the instructions you defined