MASTER: Machine Learning-based Cold Start Latency Prediction Framework in Serverless Edge Computing Environments for Industry 4.0
IEEE Journal of Selected Areas in Sensors(2024)
摘要
The integration of serverless edge computing and the Industrial Internet of Things (IIoT), like Industry 4.0 applications, is seen as a promising development that can make industrial processes more efficient and faster. These two technologies can be integrated to optimize production by enabling faster adaptation in critical industries with variable environmental conditions. However, challenges that have a negative impact on latency, such as cold start due to the serverless paradigm, are one of the challenging problems in this adaptation process. Cold start latency has recently received much attention in academia, but most proposed solutions lead to wasted resources. To address this issue, we propose a new machine learning-based resource management framework called MASTER which utilizes an Extreme Gradient Boosting (XGBoost) model to predict the cold start latency for Industry 4.0 applications for performance optimization. Furthermore, we created a new cold start dataset using an IIoT scenario (i.e. predictive maintenance) to validate the proposed MASTER framework in serverless edge computing environments. We have evaluated the performance of the MASTER framework using a real-world serverless platform, Google Cloud Platform for single-step prediction (SSP) and multiple-step prediction (MSP) operations and compared it with existing frameworks that used Deep Deterministic Policy Gradient (DDPG) and Long Short-Term Memory (LSTM) models. The experimental results show that the XGBoost-based resource management framework is the most successful model in predicting cold start with Mean Absolute Percentage Error (MAPE) values of 0.23 in SSP and 0.12 in MSP. It has been also identified that the Linear Regression model (utilized in the MASTER framework) has the least computational time (0.03 seconds) as compared to other deep learning and machine learning models considered in this work. Finally, we compare the energy consumption and
$CO_{2}$
emissions of all models to emphasize resource awareness.
更多查看译文
关键词
Serverless Computing,Edge Computing,Industry 4.0,Predictive Maintenance,Cold Start Latency
AI 理解论文
溯源树
样例
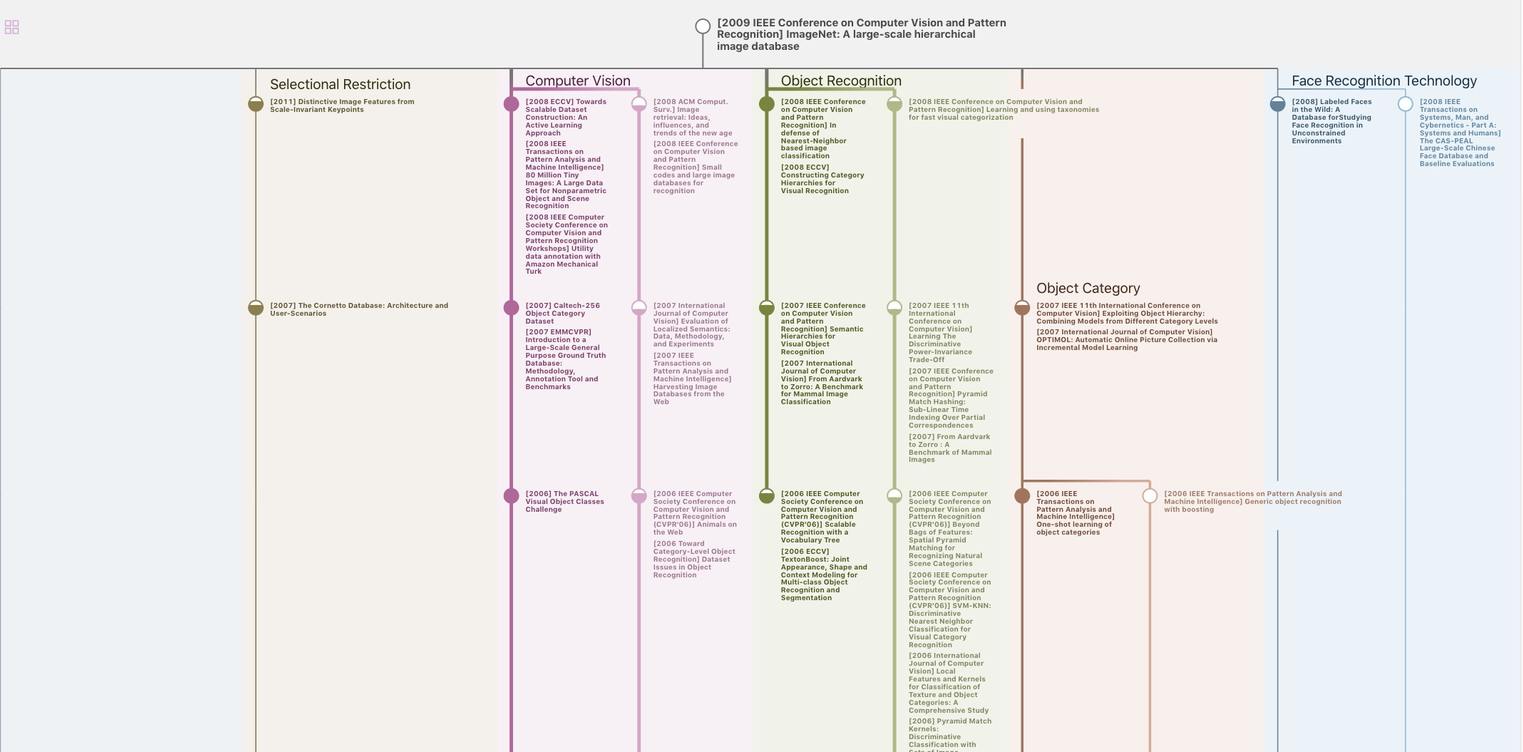
生成溯源树,研究论文发展脉络
Chat Paper
正在生成论文摘要