The Power of Question Translation Training in Multilingual Reasoning: Broadened Scope and Deepened Insights
CoRR(2024)
摘要
Bridging the significant gap between large language model's English and
non-English performance presents a great challenge. While some previous studies
attempt to mitigate this gap with translated training data, the recently
proposed question alignment approach leverages the model's English expertise to
improve multilingual performance with minimum usage of expensive, error-prone
translation. In this paper, we explore how broadly this method can be applied
by examining its effects in reasoning with executable code and reasoning with
common sense. We also explore how to apply this approach efficiently to
extremely large language models using proxy-tuning. Experiment results on
multilingual reasoning benchmarks mGSM, mSVAMP and xCSQA demonstrate that the
question alignment approach can be used to boost multilingual performance
across diverse reasoning scenarios, model families, and sizes. For instance,
when applied to the LLaMA2 models, our method brings an average accuracy
improvements of 12.2
mechanism of its success, we analyze representation space, chain-of-thought and
translation data scales, which reveals how question translation training
strengthens language alignment within LLMs and shapes their working patterns.
更多查看译文
AI 理解论文
溯源树
样例
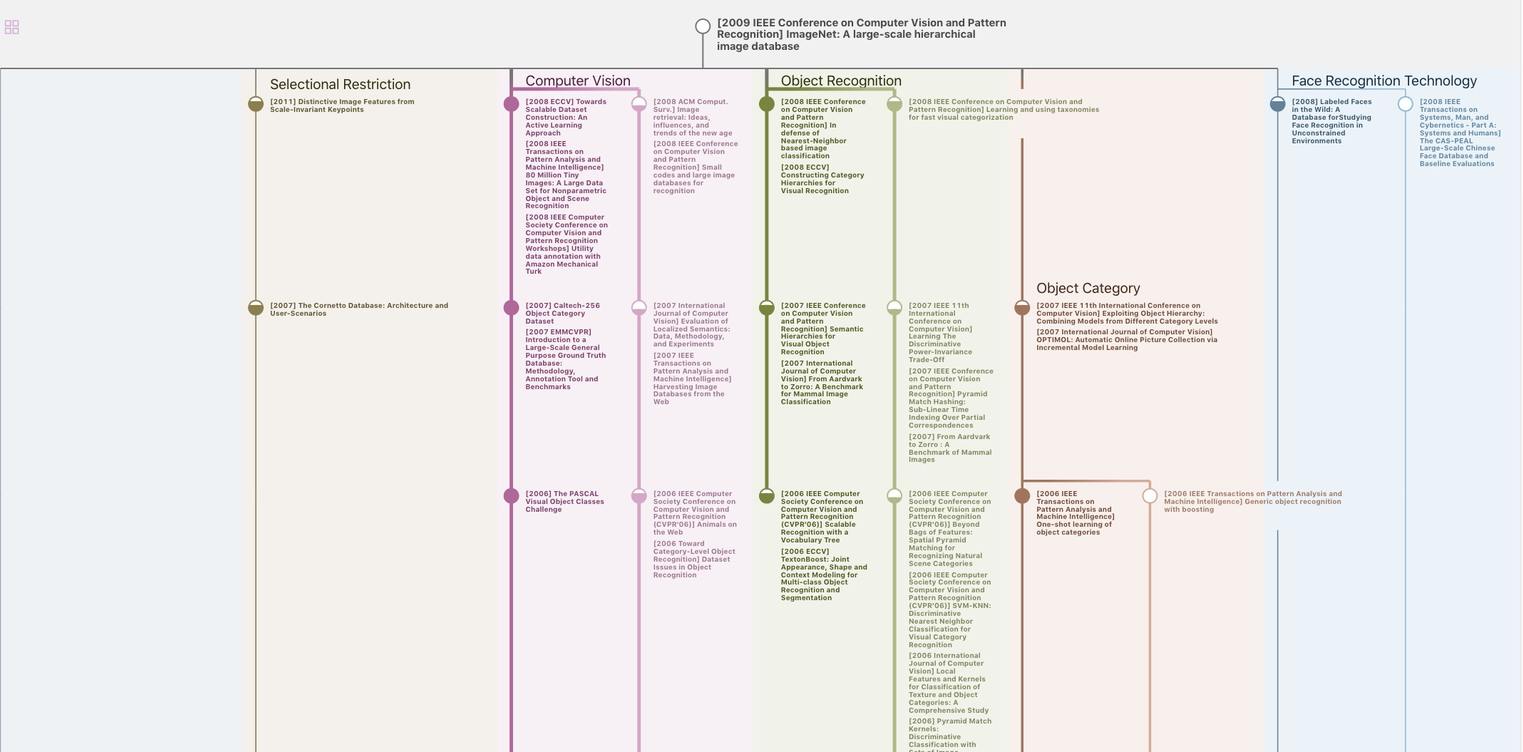
生成溯源树,研究论文发展脉络
Chat Paper
正在生成论文摘要