Non-clairvoyant Scheduling with Partial Predictions
ICML 2024(2024)
Abstract
The non-clairvoyant scheduling problem has gained new interest within learning-augmented algorithms, where the decision-maker is equipped with predictions without any quality guarantees. In practical settings, access to predictions may be reduced to specific instances, due to cost or data limitations. Our investigation focuses on scenarios where predictions for only $B$ job sizes out of $n$ are available to the algorithm. We first establish near-optimal lower bounds and algorithms in the case of perfect predictions. Subsequently, we present a learning-augmented algorithm satisfying the robustness, consistency, and smoothness criteria, and revealing a novel tradeoff between consistency and smoothness inherent in the scenario with a restricted number of predictions.
MoreTranslated text
AI Read Science
Must-Reading Tree
Example
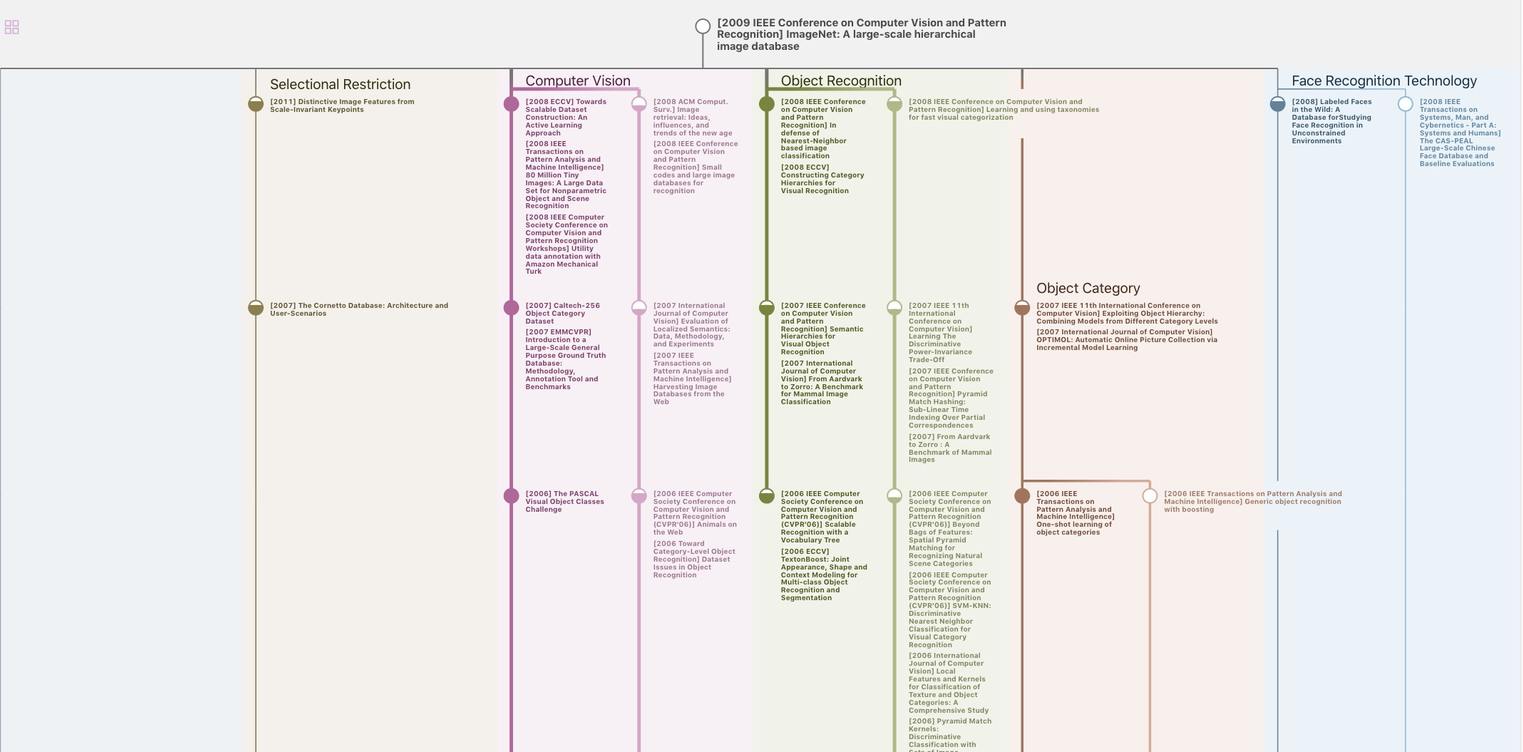
Generate MRT to find the research sequence of this paper
Chat Paper
Summary is being generated by the instructions you defined