Can't say cant? Measuring and Reasoning of Dark Jargons in Large Language Models
CoRR(2024)
摘要
Ensuring the resilience of Large Language Models (LLMs) against malicious
exploitation is paramount, with recent focus on mitigating offensive responses.
Yet, the understanding of cant or dark jargon remains unexplored. This paper
introduces a domain-specific Cant dataset and CantCounter evaluation framework,
employing Fine-Tuning, Co-Tuning, Data-Diffusion, and Data-Analysis stages.
Experiments reveal LLMs, including ChatGPT, are susceptible to cant bypassing
filters, with varying recognition accuracy influenced by question types,
setups, and prompt clues. Updated models exhibit higher acceptance rates for
cant queries. Moreover, LLM reactions differ across domains, e.g., reluctance
to engage in racism versus LGBT topics. These findings underscore LLMs'
understanding of cant and reflect training data characteristics and vendor
approaches to sensitive topics. Additionally, we assess LLMs' ability to
demonstrate reasoning capabilities. Access to our datasets and code is
available at https://github.com/cistineup/CantCounter.
更多查看译文
AI 理解论文
溯源树
样例
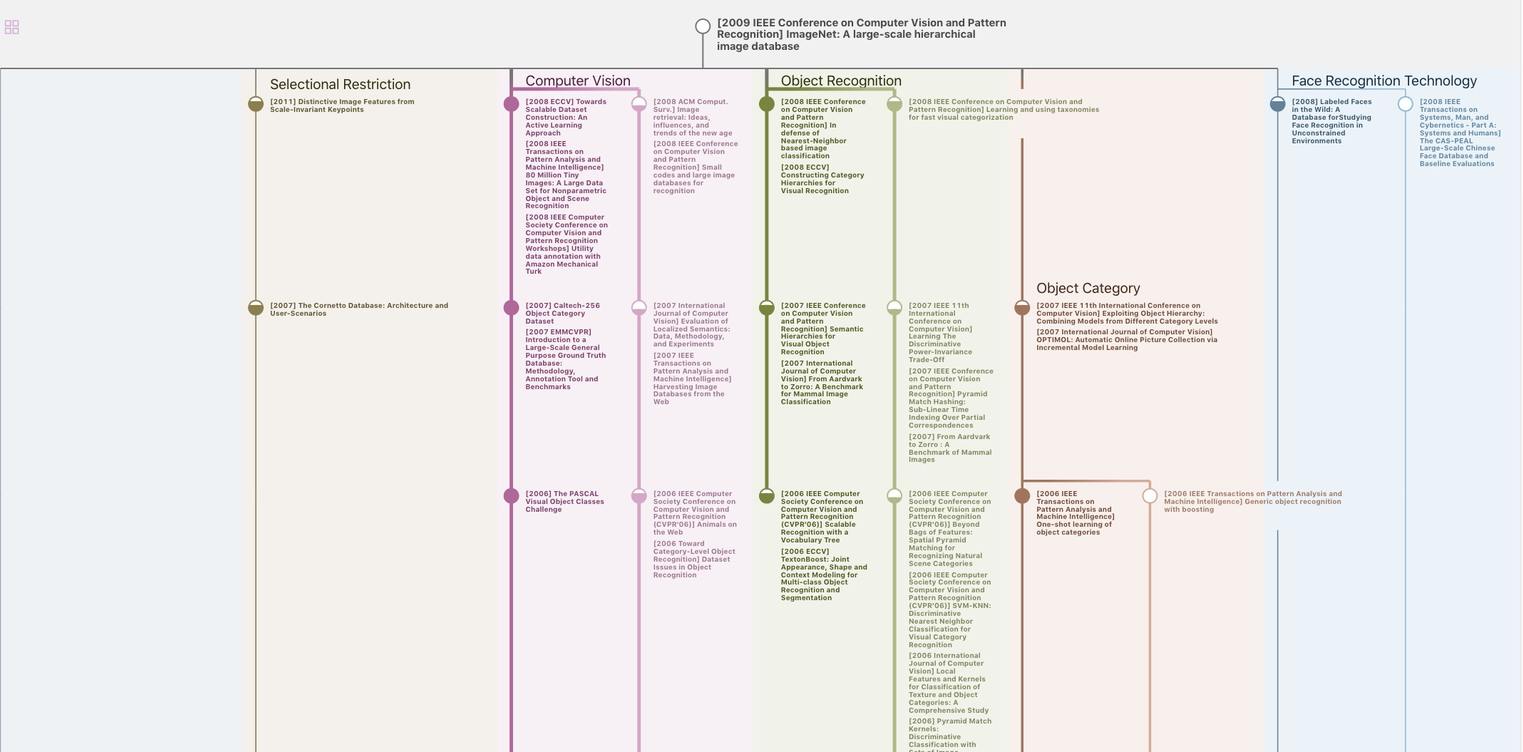
生成溯源树,研究论文发展脉络
Chat Paper
正在生成论文摘要