Exploring mechanisms of Neural Robustness: probing the bridge between geometry and spectrum
arxiv(2024)
摘要
Backpropagation-optimized artificial neural networks, while precise, lack
robustness, leading to unforeseen behaviors that affect their safety.
Biological neural systems do solve some of these issues already. Thus,
understanding the biological mechanisms of robustness is an important step
towards building trustworthy and safe systems. Unlike artificial models,
biological neurons adjust connectivity based on neighboring cell activity.
Robustness in neural representations is hypothesized to correlate with the
smoothness of the encoding manifold. Recent work suggests power law covariance
spectra, which were observed studying the primary visual cortex of mice, to be
indicative of a balanced trade-off between accuracy and robustness in
representations. Here, we show that unsupervised local learning models with
winner takes all dynamics learn such power law representations, providing
upcoming studies a mechanistic model with that characteristic. Our research
aims to understand the interplay between geometry, spectral properties,
robustness, and expressivity in neural representations. Hence, we study the
link between representation smoothness and spectrum by using weight, Jacobian
and spectral regularization while assessing performance and adversarial
robustness. Our work serves as a foundation for future research into the
mechanisms underlying power law spectra and optimally smooth encodings in both
biological and artificial systems. The insights gained may elucidate the
mechanisms that realize robust neural networks in mammalian brains and inform
the development of more stable and reliable artificial systems.
更多查看译文
AI 理解论文
溯源树
样例
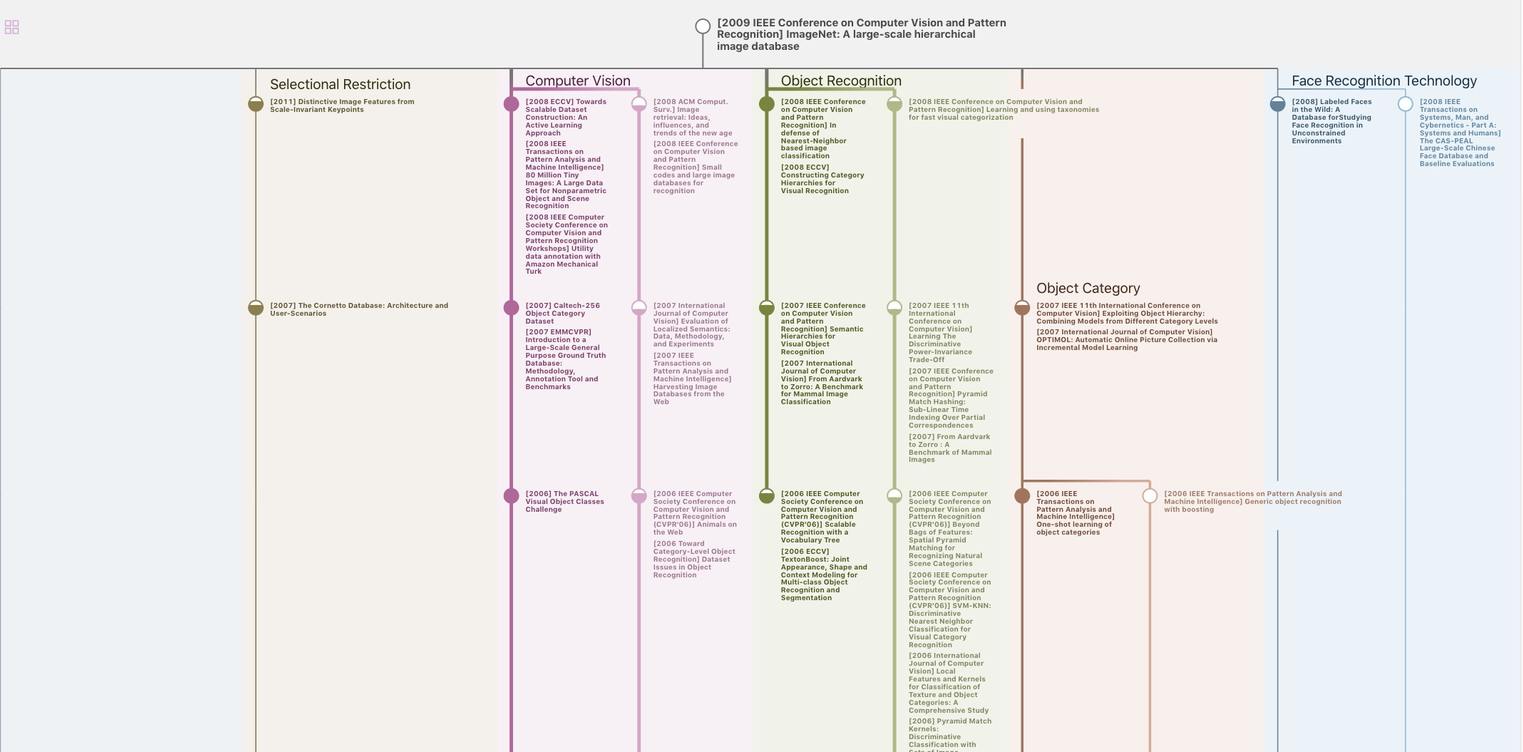
生成溯源树,研究论文发展脉络
Chat Paper
正在生成论文摘要