Towards Better Unguided Depth Completion via Cross-Modality Knowledge Distillation in the Frequency Domain
IEEE Transactions on Intelligent Vehicles(2024)
摘要
The inherent sparsity of LiDAR data often leads to extremely sparse depth maps, which poses a challenge for the development of LiDAR-based egocentric vehicles, such as self-driving cars and mobile robots. To overcome this limitation, guided depth completion methods use calibrated camera images to create precise, dense depth predictions from sparse LiDAR data. However, the extreme reliance on camera image limits its generalization of guided depth completion, especially its robustness to weather and light. In this paper, we aim to utilize camera images only during training phase to improve unguided depth completion, and discard camera in the inference phase. We comprehensively analyze the pivotal role of camera images in the depth completion task and emphasize the significance of the frequency distribution within the local windows, quantitatively demonstrating its substantial contribution. Subsequently, we introduce cross-modality knowledge distillation to align LiDAR features with camera features in the frequency domain, yielding corresponding guidance features. We devise a guidance and selection module to mitigate unavoidable inaccuracies in knowledge distillation, while it can enhance depth features and adeptly selects more precise encoded values from both the guidance branch and the unguided input. To further refine the completion result, we propose a progressive depth completion module incorporating two sub-networks connected by an attention for refinement module. This module produces weighted features from the decoder of the first stage to enhance the features in the encoder of the second stage. We denominate our method as Better Unguided Network (BUNet) and evaluate its efficacy on the KITTI depth completion benchmark and NYUv2 dataset, demonstrating its superiority over methods that exclude camera images during the inference phase.
更多查看译文
关键词
Depth completion,autonomous driving,cross-modality knowledge distillation,frequency domain
AI 理解论文
溯源树
样例
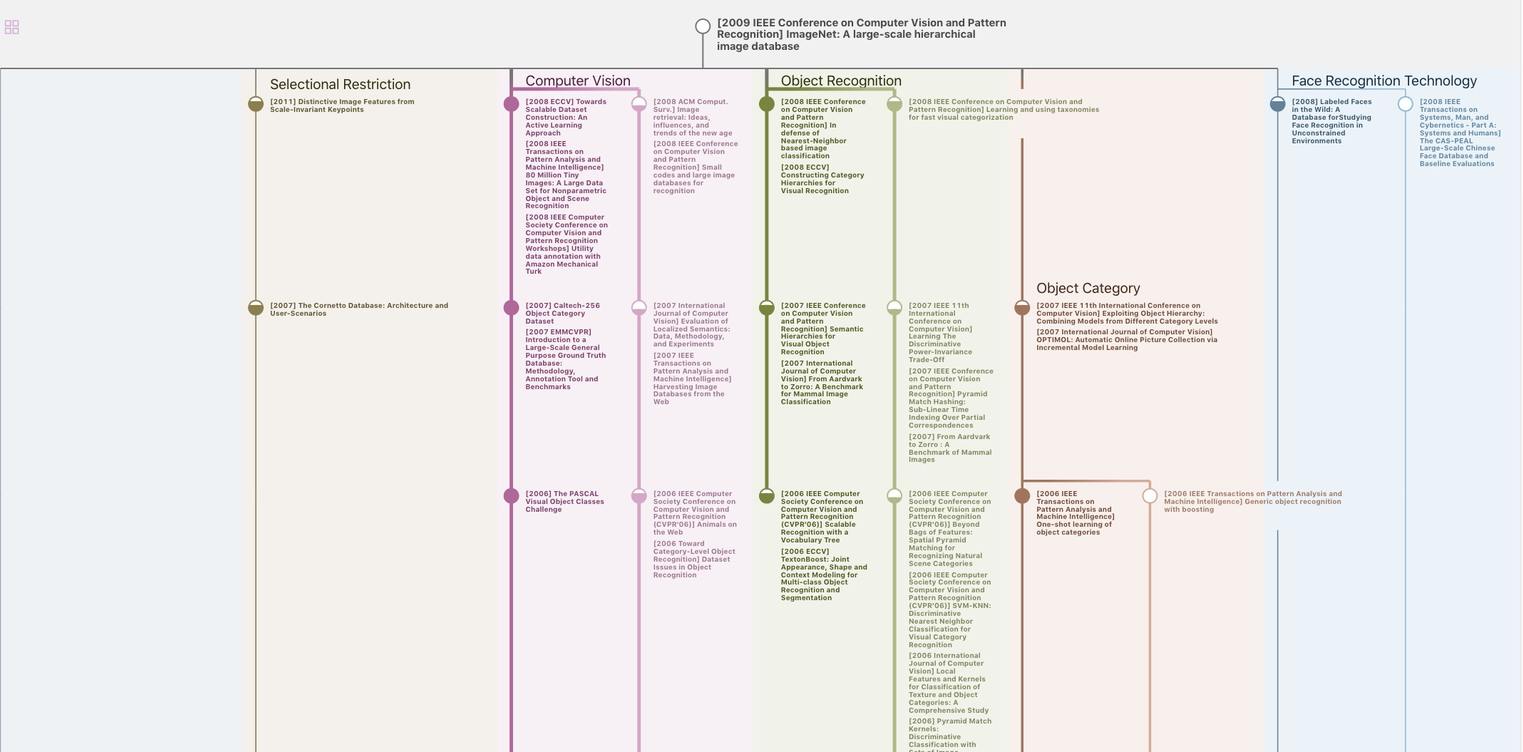
生成溯源树,研究论文发展脉络
Chat Paper
正在生成论文摘要