Detection of cervical cancer using deep transfer learning
Dutse Journal of Pure and Applied Sciences(2024)
摘要
Cervical cancer, the fourth most prevalent disease among females, is one of the major threats to the health of women worldwide. The early detection of cervical cancer is crucial for efficient disease management and medical care since it increases the likelihood of treating and curing the disease. Medically cervical cancer is usually diagnosed using pap test or colposcopic examination. This tests are time consuming and lack of enough, but with technological advancement and application of artificial intelligence to health care, machine learning algorithms has be giving faster and more accurate prediction of illness. This study made use of the readily available SIPAKMed dataset which includes the collection of a large image dataset of 4049 images. Due to the fact that detection of this type of cancer using medical images gives a faster and more accurate detection compared to using structured dataset comprising of various attributes related to cervical cancer. Pretrained EfficientNet-B7 was used as the detection model. The dataset went through series of preprocessing techniques like filtering using low and high pass filter, normalization using Min-Max Scalar. Label and image data were then converted to an array. The developed system was evaluated and gave an average accuracy and precision of 87% and 87% respectively, it was then compared with Graph Neural Network and Random Forest but they were both outperformed. As a result of this, EfficientNet-B7 is a very good deep learning algorithm for detecting cervical cancer using medical images. Other architectures of convolutional neural networks should be experimented and their performance should be compared with the developed system in future work, while larger dataset should be utilized as well.
更多查看译文
AI 理解论文
溯源树
样例
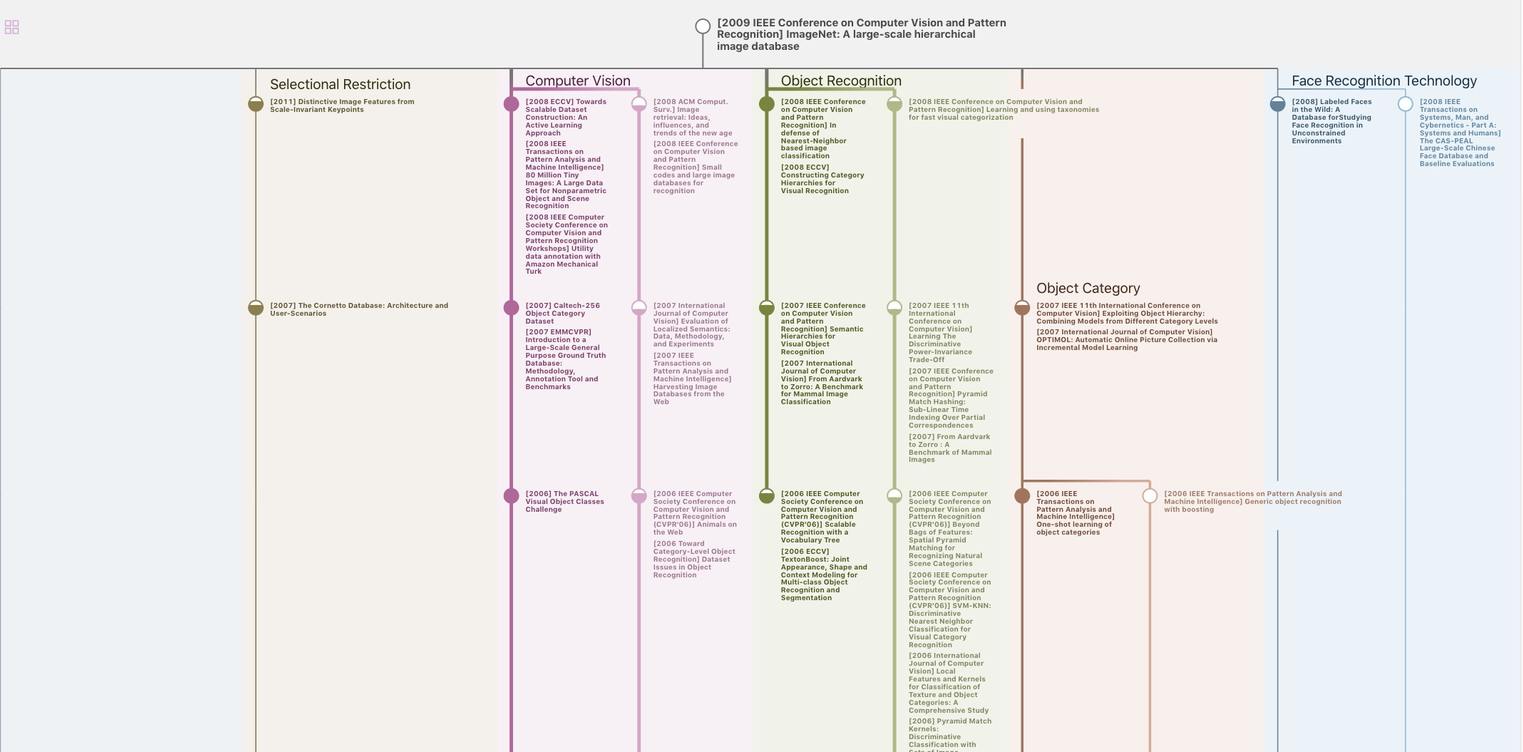
生成溯源树,研究论文发展脉络
Chat Paper
正在生成论文摘要