High impedance fault classification in microgrids using a transformer-based model with time series harmonic synchrophasors under data quality issues
Neural Computing and Applications(2024)
摘要
Recent advances in distribution networks, driven by the integration of renewable energy sources, have spurred the emergence of microgrids, elevating concerns regarded reliability and stability. In this context, precise monitoring of events, particularly those elusive to detection like high-impedance faults (HIFs), becomes imperative. The development of phasor measurement units (PMUs) with their harmonic synchronized measurements has enhanced the monitoring task and fostering the application of synchrophasors even on microgrids. This work introduces a novel method for event classification in microgrids, utilizing combined low-rate PMU data and harmonic synchrophasor time series. Central to our approach is the usage of a state-of-the-art transformer neural network, based on the attention mechanism, to effectively discern HIFs from other faulty and non-fault events. Notably, this methodology accounts for prevalent PMU data quality issues, including noise, missing data, and synchronism errors. Results from real-world HIF data demonstrate a robust performance, with an accuracy rate of approximately 98
更多查看译文
关键词
Microgrids,Harmonic synchrophasors,Transformer,High impedance faults
AI 理解论文
溯源树
样例
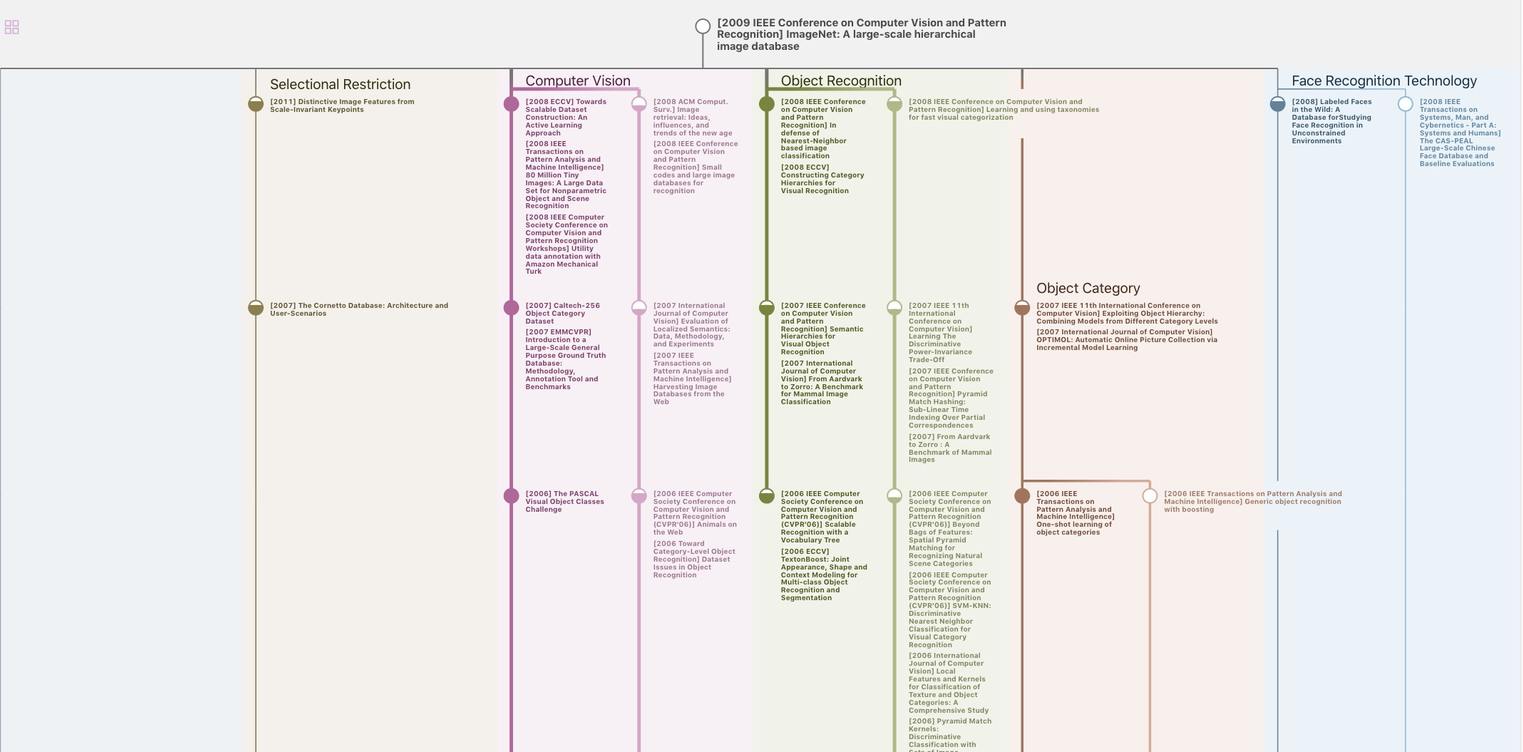
生成溯源树,研究论文发展脉络
Chat Paper
正在生成论文摘要