SnakeNet: An adaptive network for small object and complex background for insulator surface defect detection
Computers and Electrical Engineering(2024)
摘要
Aiming at the current problems of small-target defect detection difficulty, weak resistance to complex background interference, and low detection precision in insulator defect detection, an insulator defect detection model, SnakeNet, is proposed based on the YOLOv8 detection framework. Firstly, the Dynamic Snake Convolutional Neck (DSConv-Neck) is proposed to extract rich fine-grained features and improve the detection ability of small-target defects. Then, the background interference is suppressed by the C2f Efficient Multi-Scale Attention (C2MA) module to enhance the anti-background resistance; finally, the GhostConv VanillaNet (GCVNet) module is proposed to minimize the number of model parameters while ensuring the detection precision. Many experiments are carried out on two public datasets, the China power line insulator dataset (CPLID) and the Insulator Defect Image Dataset (IDID). The results show that the optimal detection precision and recall of SnakeNet are 98.7% and 98.8%, respectively, and the number of model parameters and computation volume are only 2.9M and 6.7G. Compared with the original YOLOv8, the detection precision and recall are improved by 2.4% and 3.5%, respectively, and the number of model parameters and computation volume are reduced by 0.3M and 1.3M, respectively. Compared with other algorithms, such as SSD and RT-DETR, SnakeNet performs well in detecting small targets and adapting to complex backgrounds with lower computational costs.
更多查看译文
关键词
Deep learning,Defect detection,Power line insulators,SnakeNet,YOLOv8
AI 理解论文
溯源树
样例
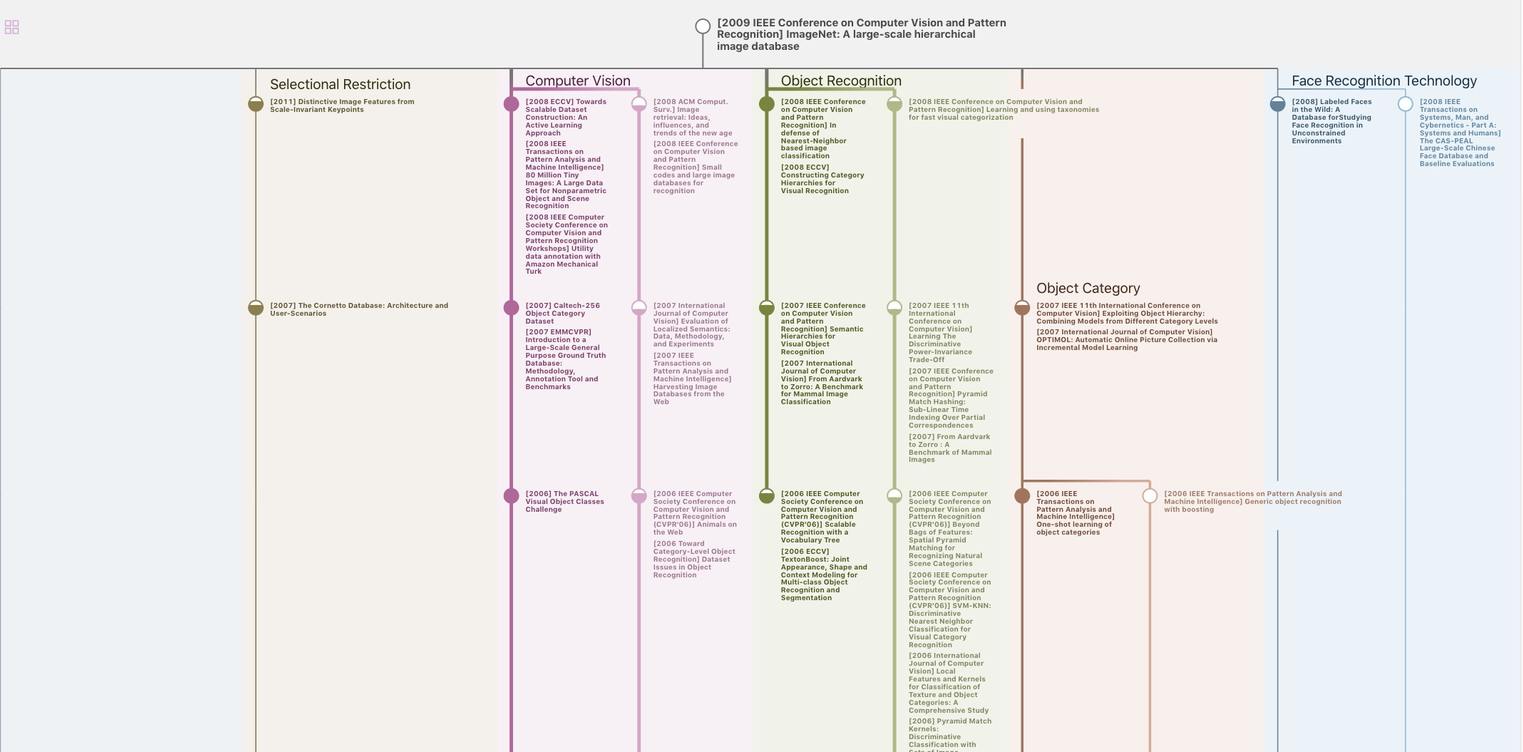
生成溯源树,研究论文发展脉络
Chat Paper
正在生成论文摘要