PP-Loss: An imbalanced regression loss based on plotting position for improved precipitation nowcasting
Theoretical and Applied Climatology(2024)
摘要
Precipitation nowcasting refers to the accurate and timely forecasting of rainfall in a certain area for the near future, which plays a key role in severe convective weather warnings, water resources, and flood risk management. Rainfall data sample imbalance has always been an important issue that needs to be addressed in precipitation forecasting. It has been shown that an appropriate constraint can be introduced into the loss function of deep learning models to reduce the negative impact of imbalanced data on model training. In this work, we propose a sample weighting approach for imbalanced precipitation datasets called Plotting Position Weight (PP-weight), and a cost-sensitive learning approach based on weighting schemes, called Plotting Position Loss (PP-Loss). The developed PP-Loss is used to adjust the impact of each data point on the loss through the PP-weight calculated based on the ranking of the position of the data values. We explore the manipulation of imbalanced regression loss function by the convolutional long short-term memory (ConvLSTM) model on the ERA5-Land precipitation dataset. Experimental results suggest that the ConvLSTM model using PP-Loss is comparable to that using state-of-the-art loss functions (mean square error, Focal-R, Gumbel Loss, and label distribution smoothing), obtaining an R2 of 0.886 and a root mean square error of 2.220 mm when using 3-h precipitation data as a learning sample to predict precipitation for the next hour. Therefore, the proposed approach may be expected to contribute to applying imbalanced regression methods in deep learning precipitation forecasting research.
更多查看译文
AI 理解论文
溯源树
样例
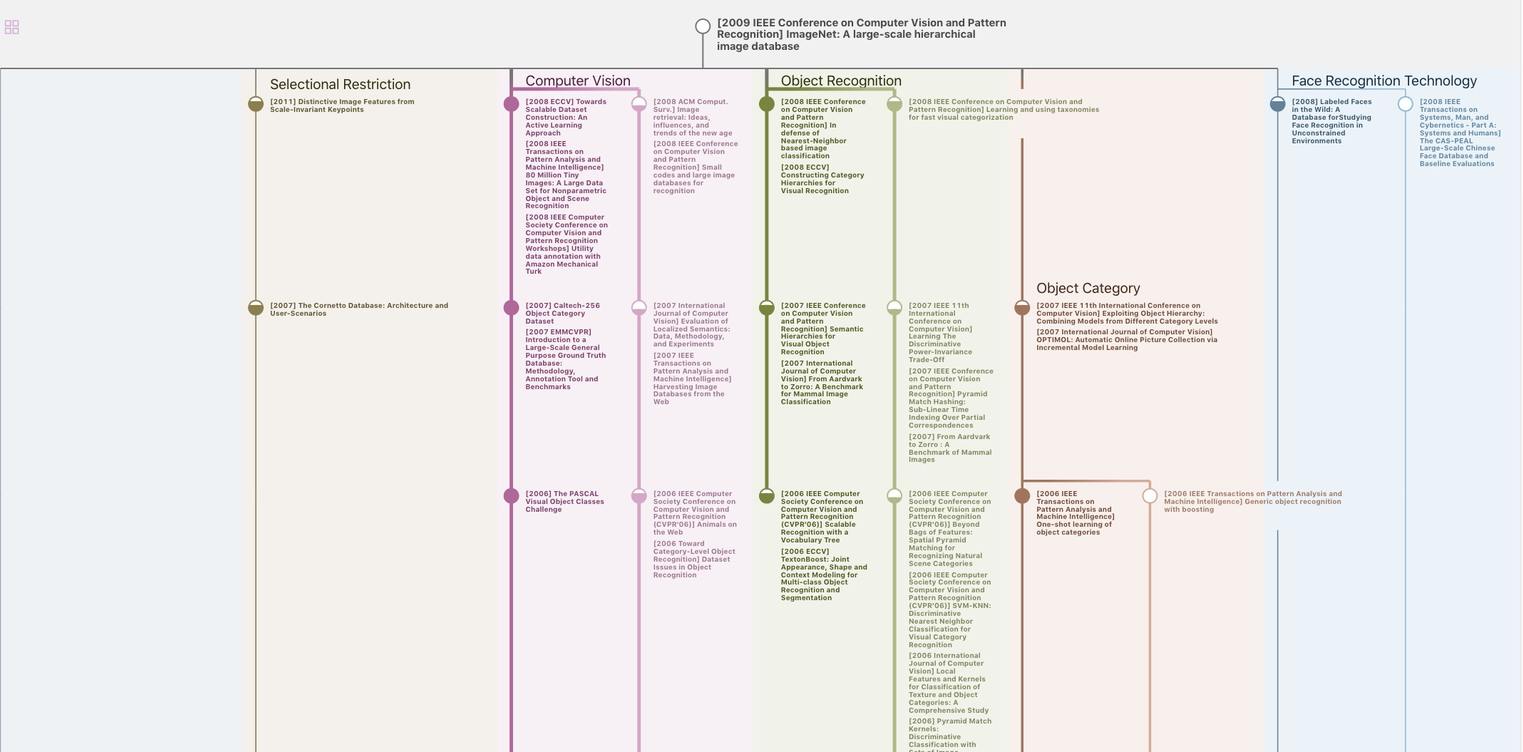
生成溯源树,研究论文发展脉络
Chat Paper
正在生成论文摘要