Textural features for BLB disease damage assessment in paddy fields using drone data and machine learning: Enhancing disease detection accuracy
Smart Agricultural Technology(2024)
摘要
Detecting Bacterial Leaf Blight (BLB) in paddy fields is a critical challenge in Indonesia, where the disease poses a significant threat to rice production by reducing the photosynthetic ability and ultimately compromising plant productivity. This study explored the effectiveness of using drone-acquired data for textural analysis in paddy fields in West Java, with the aim of improving BLB detection by integrating textural and thermal characteristics. Utilizing advanced machine learning techniques, we combined drone data to assess different levels of damage caused by BLB. The normalized difference texture index, derived from the Haralick textural features, was employed as a key predictor. Our findings demonstrate that the inclusion of textural features markedly enhances disease detection accuracy compared with traditional methods based solely on spectral indices. Specifically, the random forest algorithm, which integrates texture and vegetation indices, achieved an impressive classification accuracy of 0.984. This innovative approach offers a robust, non-invasive solution for detecting BLB, significantly contributing to the protection of crop yields and addressing global food security challenges. This study underscores the potential of advanced remote sensing technologies and machine learning to revolutionize agricultural disease management.
更多查看译文
关键词
Crop diseases,UAV,Machine learning,Precision agriculture,Rice,Texture analysis,Zero hunger
AI 理解论文
溯源树
样例
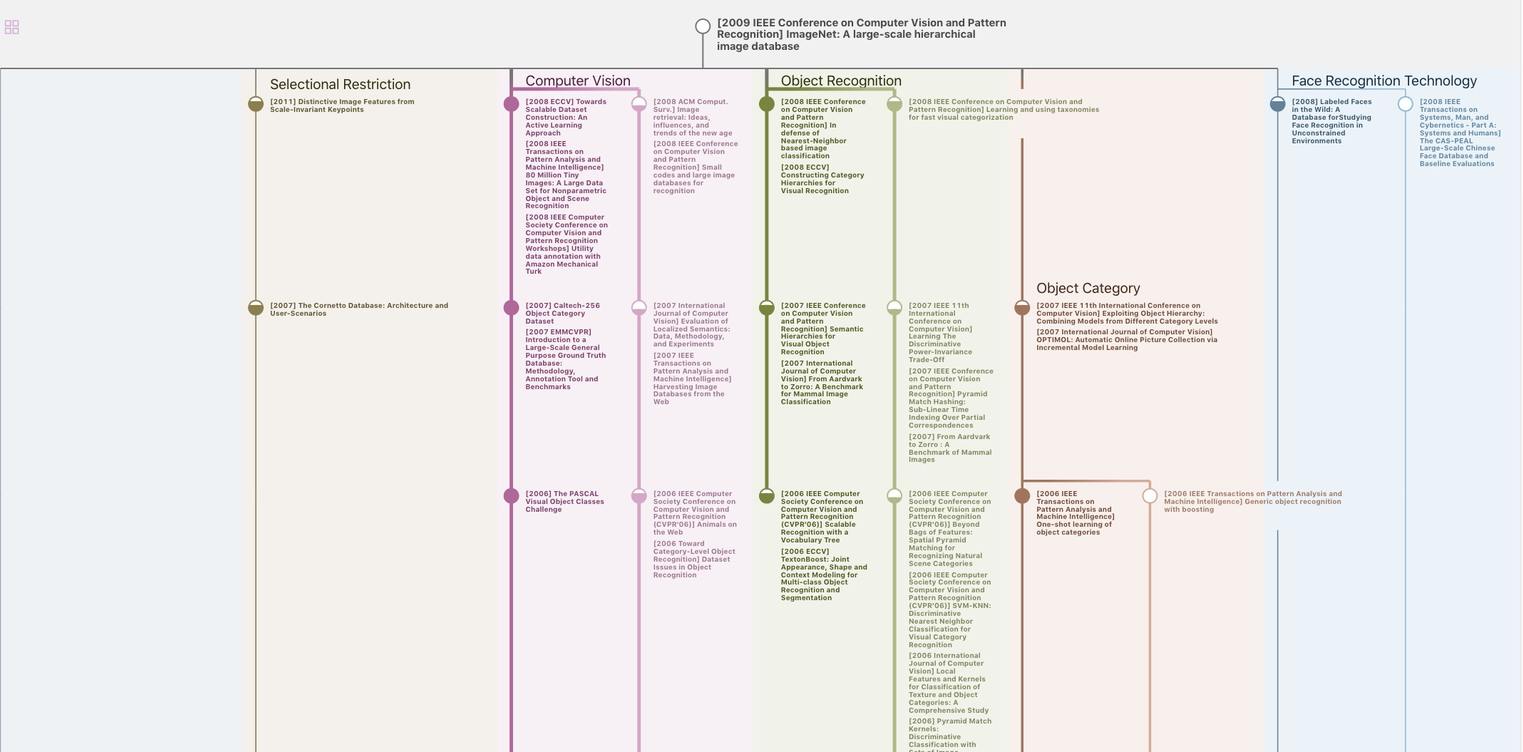
生成溯源树,研究论文发展脉络
Chat Paper
正在生成论文摘要