Machine-Learning-Assisted Descriptors Identification for Indoor Formaldehyde Oxidation Catalysts.
Environmental science & technology(2024)
摘要
The development of highly efficient catalysts for formaldehyde (HCHO) oxidation is of significant interest for the improvement of indoor air quality. Up to 400 works relating to the catalytic oxidation of HCHO have been published to date; however, their analysis for collective inference through conventional literature search is still a challenging task. A machine learning (ML) framework was presented to predict catalyst performance from experimental descriptors based on an HCHO oxidation catalysts database. MnOx, CeO2, Co3O4, TiO2, FeOx, ZrO2, Al2O3, SiO2, and carbon-based catalysts with different promoters were compiled from the literature. Notably, 20 descriptors including reaction catalyst composition, reaction conditions, and catalyst physical properties were collected for data mining (2263 data points). Furthermore, the eXtreme Gradient Boosting algorithm was employed, which successfully predicted the conversion efficiency of HCHO with an R-square value of 0.81. Shapley additive analysis suggested Pt/MnO2 and Ag/Ce-Co3O4 exhibited excellent catalytic performance of HCHO oxidation based on the analysis of the entire database. Validated by experimental tests and theoretical simulations, the key descriptor identified by ML, i.e., the first promoter, was further described as metal-support interactions. This study highlights ML as a useful tool for database establishment and the catalyst rational design strategy based on the importance of analysis between experimental descriptors and the performance of complex catalytic systems.
更多查看译文
AI 理解论文
溯源树
样例
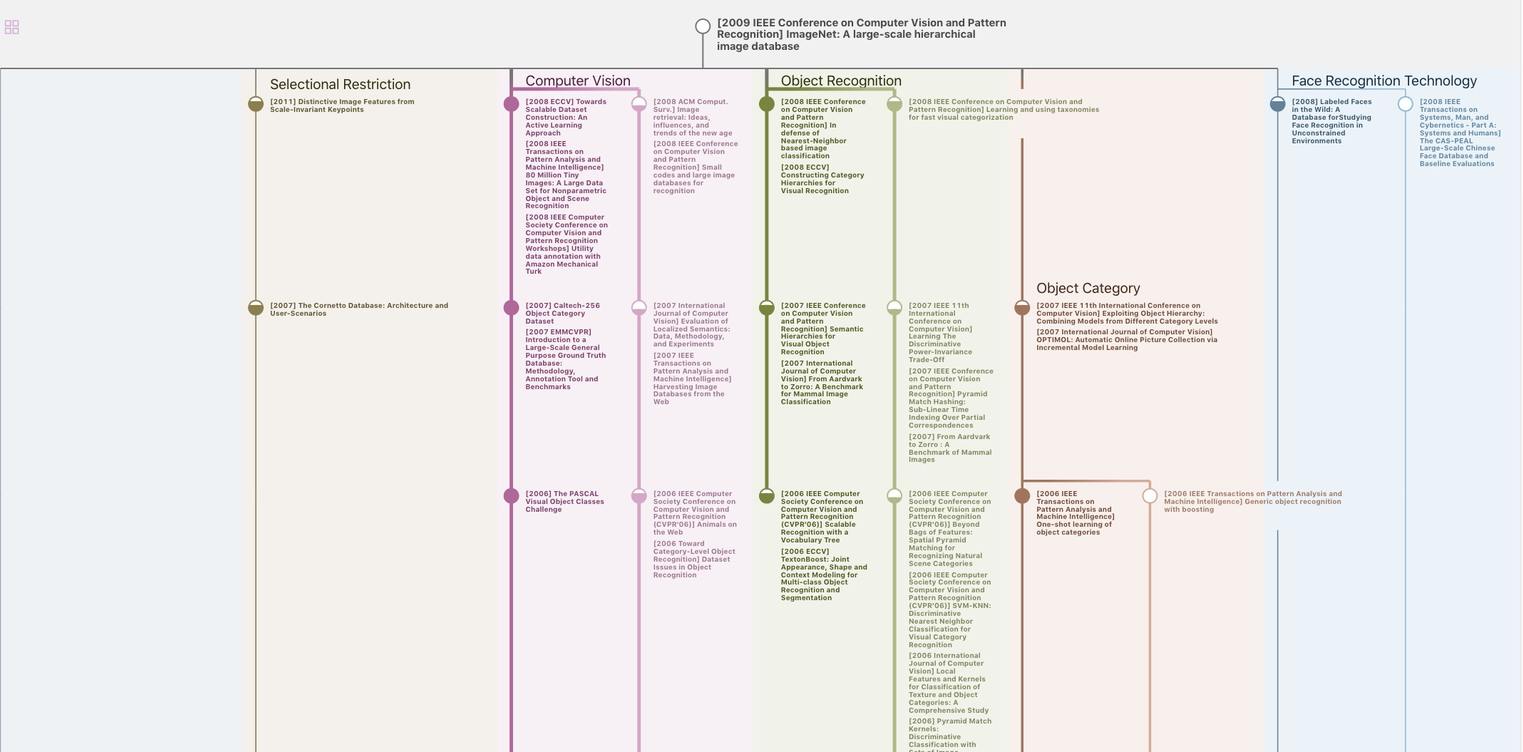
生成溯源树,研究论文发展脉络
Chat Paper
正在生成论文摘要