Rockafellian Relaxation for PDE-Constrained Optimization with Distributional Uncertainty
arxiv(2024)
摘要
Stochastic optimization problems are generally known to be ill-conditioned to
the form of the underlying uncertainty. A framework is introduced for optimal
control problems with partial differential equations as constraints that is
robust to inaccuracies in the precise form of the problem uncertainty. The
framework is based on problem relaxation and involves optimizing a bivariate,
"Rockafellian" objective functional that features both a standard control
variable and an additional perturbation variable that handles the
distributional ambiguity. In the presence of distributional corruption, the
Rockafellian objective functionals are shown in the appropriate settings to
Γ-converge to uncorrupted objective functionals in the limit of
vanishing corruption. Numerical examples illustrate the framework's utility for
outlier detection and removal and for variance reduction.
更多查看译文
AI 理解论文
溯源树
样例
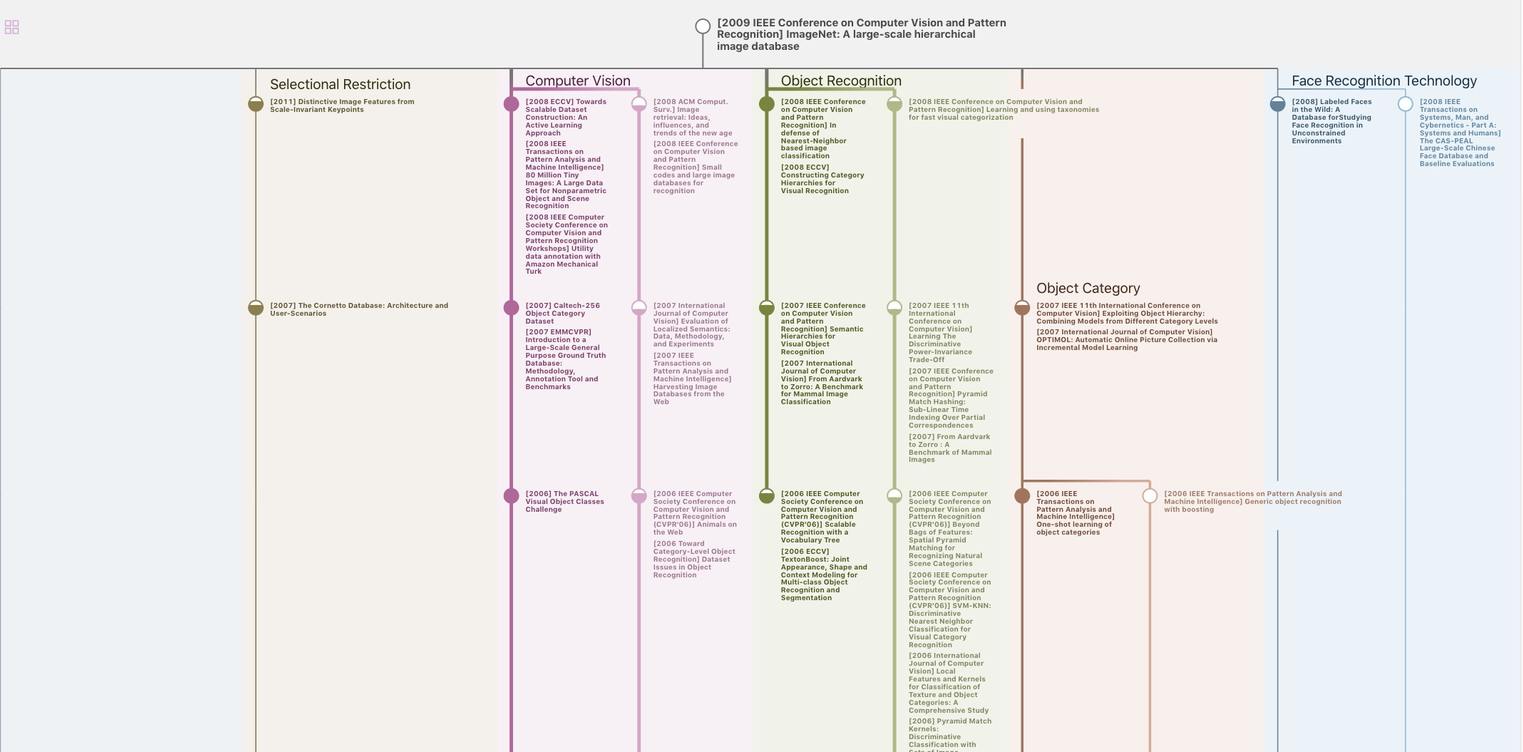
生成溯源树,研究论文发展脉络
Chat Paper
正在生成论文摘要