Greater Benefits of Deep Learning-Based Computer-Aided Detection Systems for Finding Small Signals in 3D Volumetric Medical Images
Journal of Medical Imaging(2024)
Key words
visual search,3D volumetric images,computer-aided detection,convolutional neural networks,foveated vision
AI Read Science
Must-Reading Tree
Example
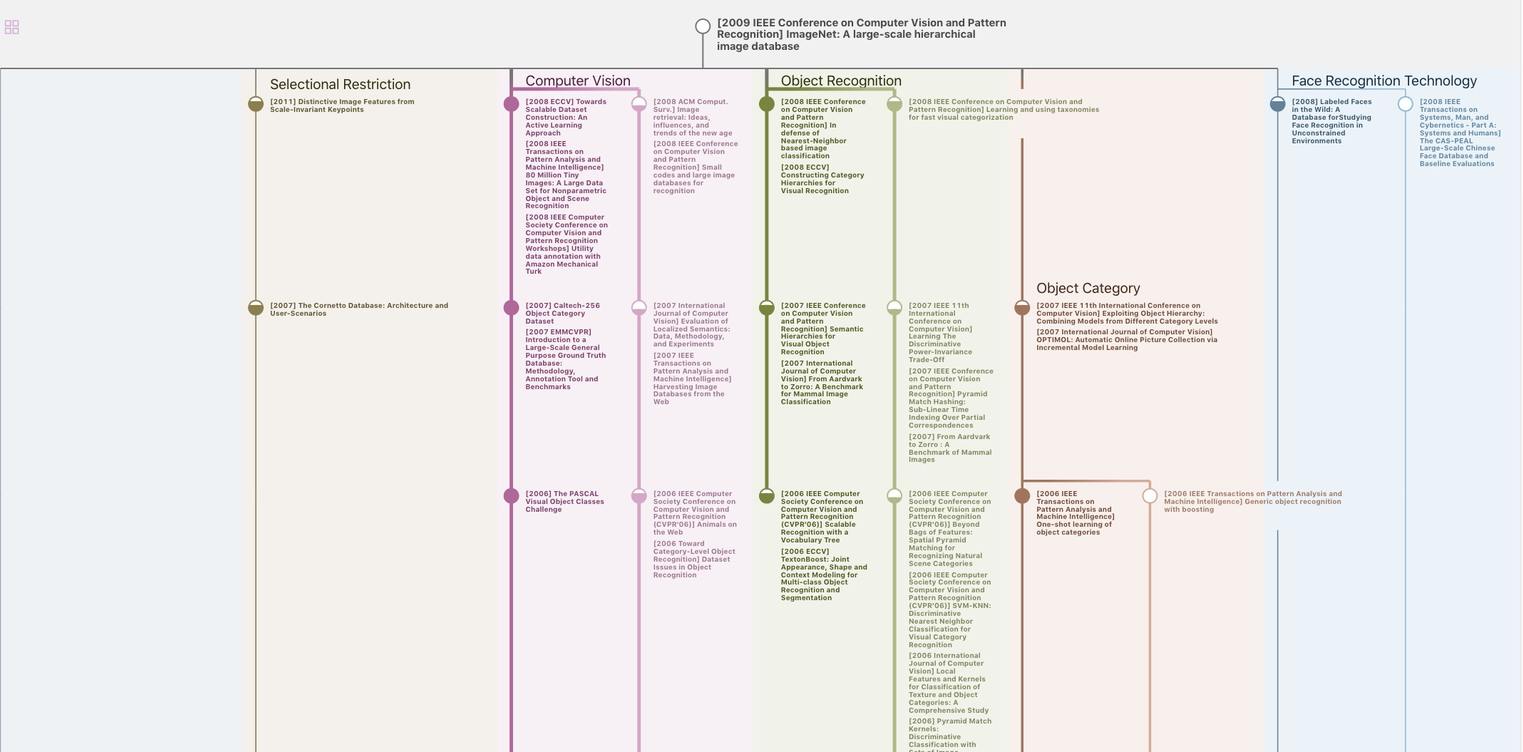
Generate MRT to find the research sequence of this paper
Chat Paper
Summary is being generated by the instructions you defined