Predicting Daily Suspended Sediment Load in Rivers Using Hybrid and Deep Learning Models-Case Study: Kharestan Watershed
Iranian Journal of Science and Technology, Transactions of Civil Engineering(2024)
摘要
Suspended sediment load (SSL) is one of the important parts of the river sediment load which its prediction is not easy due to the its nonlinear and complex nature. Knowing the amount of SSL is an important factor in the design of river engineering projects, water resources and environmental management. Hence, it is necessary to measure or predict its amount accurately. The goal of the current study is to evaluate the efficacy of hybrid model integrating multi-layer perceptron with Particle Swarm Optimization algorithm (MLP-PSO) for predicting daily SSL and its comparison with long short-term memory (LSTM) deep learning model and MLP at Jamal Beyg stations in Shour River, Fars province, Iran. To attain this goal, the daily SSL and streamflow data (Qt) of Jamal Beyg station were collected during a period of 30 years. The streamflow at time t (Qt) and streamflow with the delays 1–5 days were considered as primary input variables and SSL as output variable. Partial mutual information (PMI) algorithm was used for recognizing efficient input variables on daily SSL among the primary input variables. Then, the values of daily SSL were predicted by MLP-PSO, MLP and LSTM models and their accuracy evaluated based on RMSE, R and NSE criteria. The results of PMI algorithm showed that Qt and Qt-1 variables are efficient input variables on SSL. Also, the evaluation results of models revealed that MLP-PSO hybrid model with lower values of RMSE = 26.952 ton/day and high values of NSE = 0.716 and R = 0.870 provides the best performance compared to MLP and LSTM models for predicting daily SSL at Jamal Beyg station.
更多查看译文
关键词
MLP,PSO,LSTM,SSL,PMI
AI 理解论文
溯源树
样例
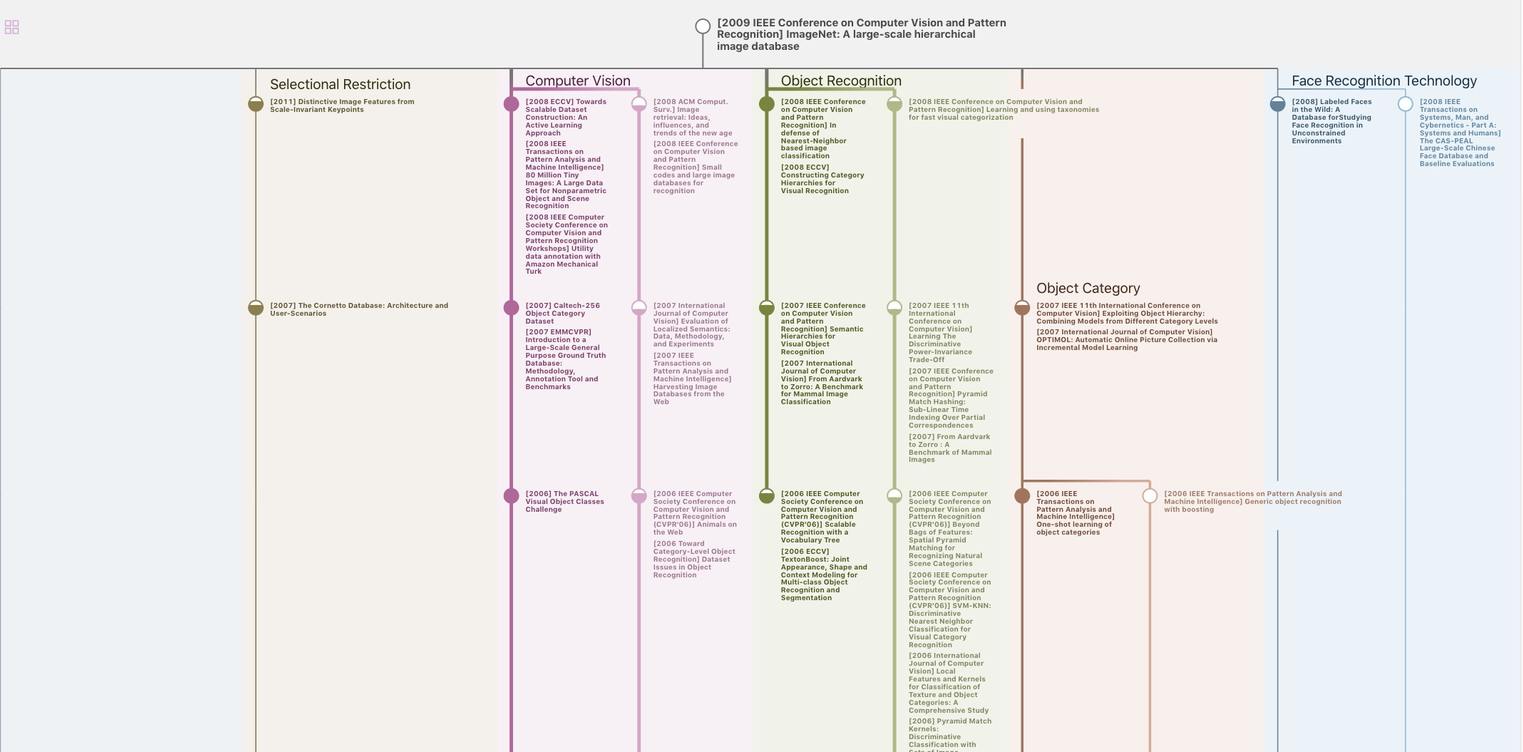
生成溯源树,研究论文发展脉络
Chat Paper
正在生成论文摘要