An autoregressive integrated moving average and long short-term memory (ARIM-LSTM) hybrid model for multi-source epidemic data prediction
PeerJ Computer Science(2024)
摘要
The COVID-19 pandemic has far-reaching impacts on the global economy and public health. To prevent the recurrence of pandemic outbreaks, the development of short-term prediction models is of paramount importance. We propose an ARIMA-LSTM (autoregressive integrated moving average and long short-term memory) model for predicting future cases and utilize multi-source data to enhance prediction performance. Firstly, we employ the ARIMA-LSTM model to forecast the developmental trends of multi-source data separately. Subsequently, we introduce a Bayes-Attention mechanism to integrate the prediction outcomes from auxiliary data sources into the case data. Finally, experiments are conducted based on real datasets. The results demonstrate a close correlation between predicted and actual case numbers, with superior prediction performance of this model compared to baseline and other state-of-the-art methods.
更多查看译文
关键词
Epidemic trend forecasting,Multi-source data fusion,ARIMA model,LSTM model,Bayes-Attention mechanism
AI 理解论文
溯源树
样例
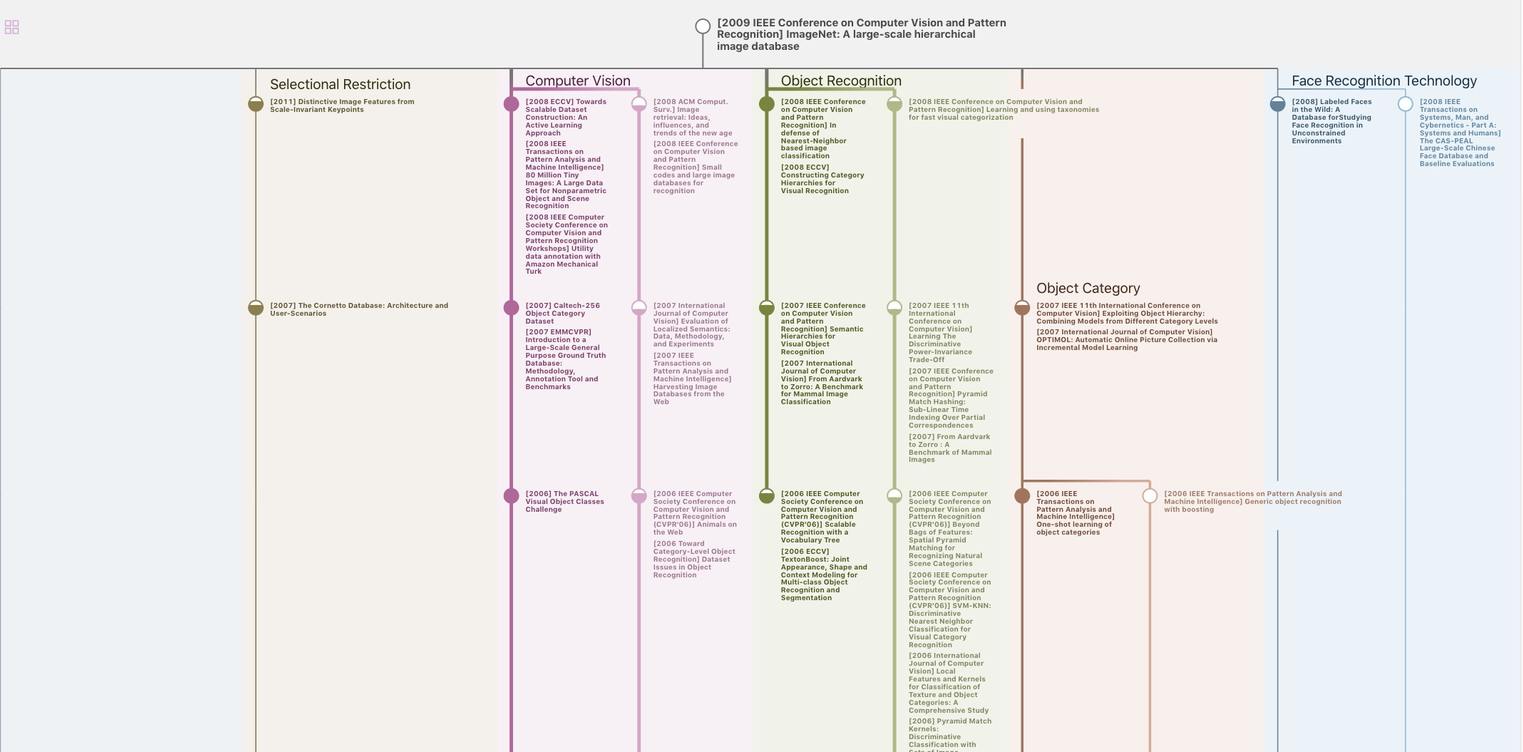
生成溯源树,研究论文发展脉络
Chat Paper
正在生成论文摘要