A Variance-Based Sensitivity Analysis Approach for Identifying Interactive Exposures
Statistics in Biosciences(2024)
摘要
Chemical mixtures can significantly affect human health, but understanding the interactions among various chemical exposures and identifying influential ones in relation to some health outcomes are difficult. Bayesian kernel machine regression (BKMR) is a widely used model for capturing nonlinear dynamics and interactions between multiple exposures and health outcomes. However, tools for quantifying the interactions captured by this flexible model are scarce. Utilizing the inherent connection between BKMR and Gaussian process regressions, we adopt the classic variance-based sensitivity analysis tools from the uncertainty quantification community and propose a variable clustering approach to quantify interactions, discover high-order interaction terms, and rank variable importance. The performance of this method is demonstrated in a range of simulation scenarios and applied to a real dataset to examine the interactive effects of multiple per- and polyfluoroalkyl substances exposures, dietary patterns, and gestational diabetes mellitus status on thyroid function in women during their late pregnancy.
更多查看译文
关键词
Gaussian process regression,Sensitivity analysis,Interaction,Chemical mixture
AI 理解论文
溯源树
样例
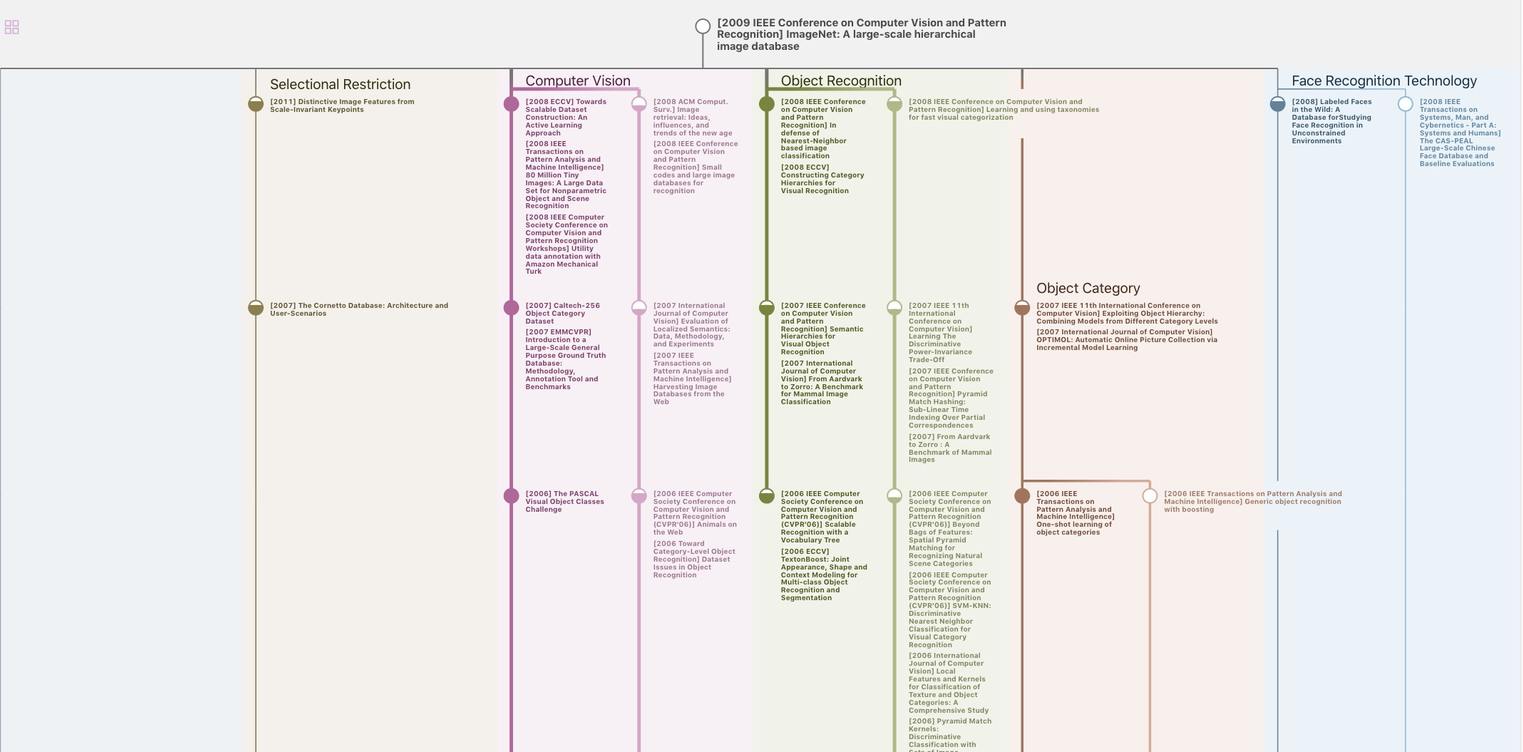
生成溯源树,研究论文发展脉络
Chat Paper
正在生成论文摘要