Smooth-Guided Implicit Data Augmentation for Domain Generalization
IEEE TRANSACTIONS ON NEURAL NETWORKS AND LEARNING SYSTEMS(2024)
Abstract
The training process of a domain generalization (DG) model involves utilizing one or more interrelated source domains to attain optimal performance on an unseen target domain. Existing DG methods often use auxiliary networks or require high computational costs to improve the model's generalization ability by incorporating a diverse set of source domains. In contrast, this work proposes a method called Smooth-Guided Implicit Data Augmentation (SGIDA) that operates in the feature space to capture the diversity of source domains. To amplify the model's generalization capacity, a distance metric learning (DML) loss function is incorporated. Additionally, rather than depending on deep features, the suggested approach employs logits produced from cross entropy (CE) losses with infinite augmentations. A theoretical analysis shows that logits are effective in estimating distances defined on original features, and the proposed approach is thoroughly analyzed to provide a better understanding of why logits are beneficial for DG. Moreover, to increase the diversity of the source domain, a sampling-based method called smooth is introduced to obtain semantic directions from interclass relations. The effectiveness of the proposed approach is demonstrated through extensive experiments on widely used DG, object detection, and remote sensing datasets, where it achieves significant improvements over existing state-of-the-art methods across various backbone networks.
MoreTranslated text
Key words
Distance metric learning (DML),domain generalization (DG),implicit data augmentation,smooth
AI Read Science
Must-Reading Tree
Example
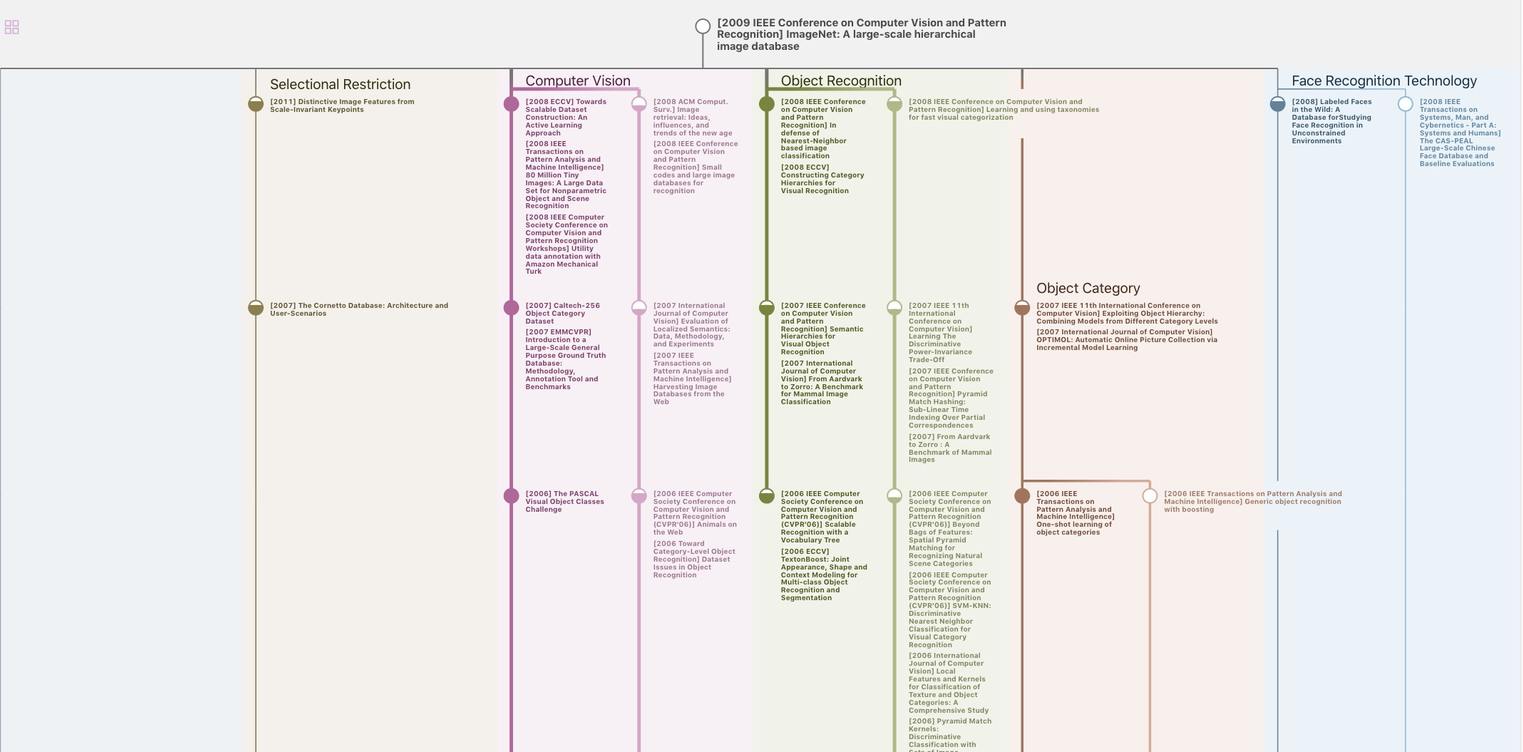
Generate MRT to find the research sequence of this paper
Chat Paper
Summary is being generated by the instructions you defined