Dual Behavior Regularized Offline Deterministic Actor–Critic
IEEE Transactions on Systems, Man, and Cybernetics: Systems(2024)
摘要
To mitigate the extrapolation error arising from offline reinforcement learning (RL) paradigm, existing methods typically make learned
$Q$
-functions over-conservative or enforce global policy constraints. In this article, we propose a dual behavior regularized offline deterministic Actor–Critic (DBRAC) by simultaneously performing behavior regularization on the coupling-iterative policy evaluation (PE) and policy improvement (PI) in the policy iteration process. In the PE phase, the difference between the
$Q$
-function and behavior value is first taken as the anti-exploration behavior value regularization term to drive the
$Q$
-function toward its true
$Q$
-value, which significantly reduces the conservatism of learned
$Q$
-function. In the PI phase, the estimated action variances of behavior policy in different states are then utilized for designing the weight and threshold of mild-local behavior cloning regularization term, which standardizes the local improvement potential of learned policy. Experiments on the well-known datasets for deep data-driven RL (D4RL) demonstrate that the DBRAC can quickly learn more competitive task-solving policies in various offline situations with different data qualities, significantly outperforming state-of-the-art offline RL baselines.
更多查看译文
关键词
Anti-exploration behavior value,dual behavior regularization (DBR),mild-local behavior cloning (BC),offline deterministic Actor–Critic,reinforcement learning (RL)
AI 理解论文
溯源树
样例
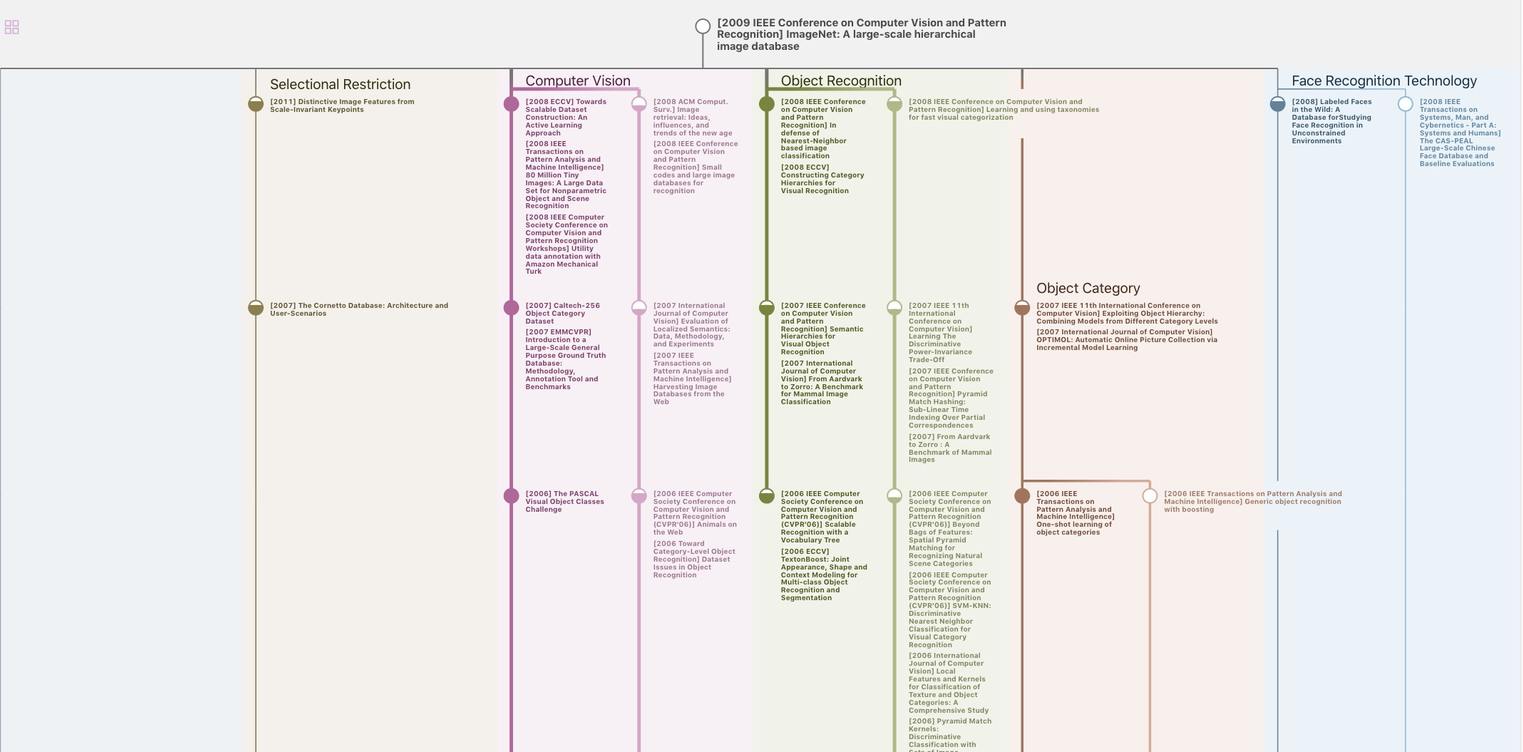
生成溯源树,研究论文发展脉络
Chat Paper
正在生成论文摘要