CURE: A deep learning framework pre-trained on large-scale patient data for treatment effect estimation
Patterns(2024)
摘要
Treatment effect estimation (TEE) aims to identify the causal effects of treatments on important outcomes. Current machine-learning-based methods, mainly trained on labeled data for specific treatments or outcomes, can be sub-optimal with limited labeled data. In this article, we propose a new pre-training and fine-tuning framework, CURE (causal treatment effect estimation), for TEE from observational data. CURE is pre-trained on large-scale unlabeled patient data to learn representative contextual patient representations and fine-tuned on labeled patient data for TEE. We present a new sequence encoding approach for longitudinal patient data embedding both structure and time. Evaluated on four downstream TEE tasks, CURE outperforms the state-of-the-art methods, marking a 7% increase in area under the precision-recall curve and an 8% rise in the influence-function-based precision of estimating heterogeneous effects. Validation with four randomized clinical trials confirms its efficacy in producing trial conclusions, highlighting CURE’s capacity to supplement traditional clinical trials.
更多查看译文
关键词
treatment effect estimation,pre-training and fine-tuning,real-world patient data
AI 理解论文
溯源树
样例
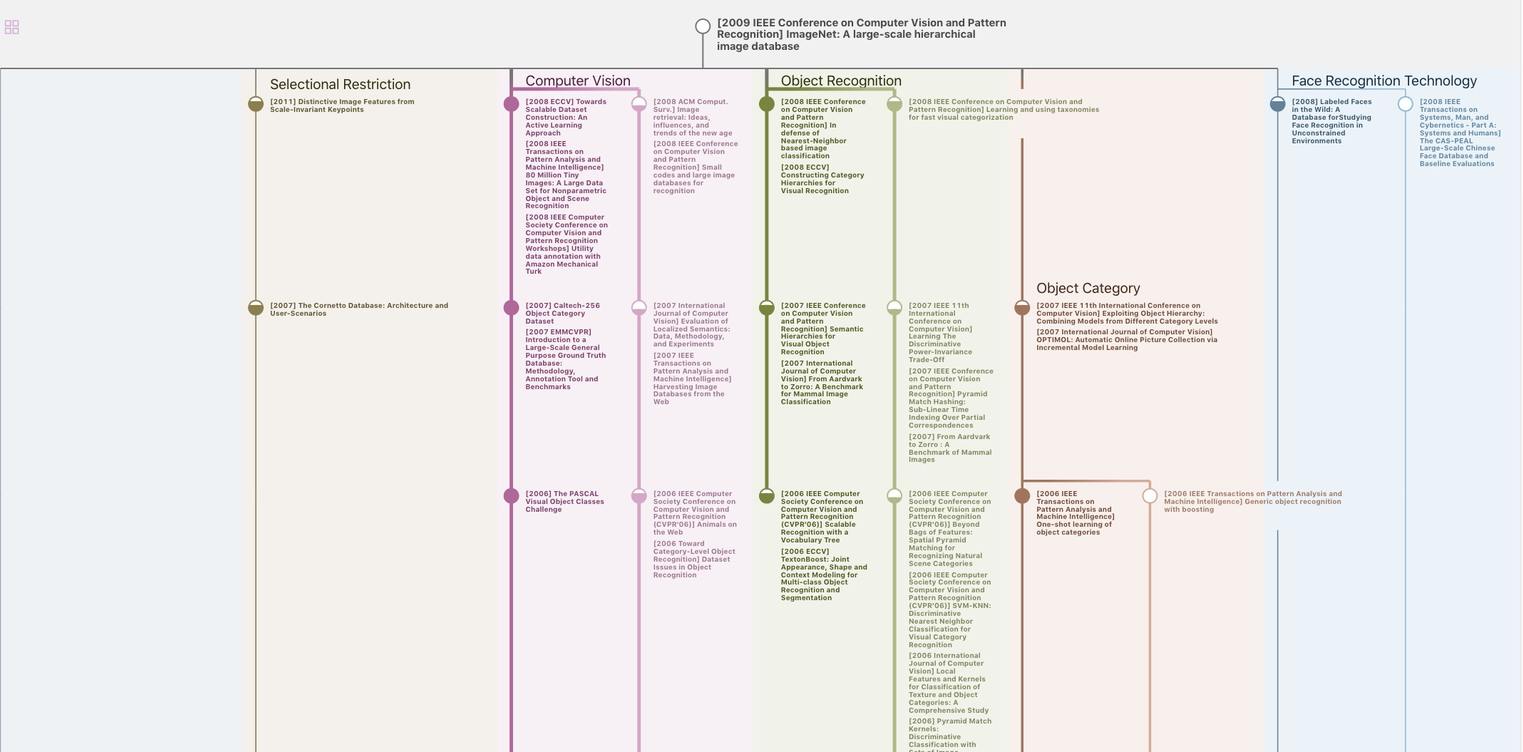
生成溯源树,研究论文发展脉络
Chat Paper
正在生成论文摘要