[Identification of Protein-Coding Gene Markers in Breast Invasive Carcinoma Based on Machine Learning].
Zhongguo yi xue ke xue yuan xue bao. Acta Academiae Medicinae Sinicae(2024)
摘要
Objective To screen out the biomarkers linked to prognosis of breast invasive carcinoma based on the analysis of transcriptome data by random forest (RF),extreme gradient boosting (XGBoost),light gradient boosting machine (LightGBM),and categorical boosting (CatBoost). Methods We obtained the expression data of breast invasive carcinoma from The Cancer Genome Atlas and employed DESeq2,t-test,and Cox univariate analysis to identify the differentially expressed protein-coding genes associated with survival prognosis in human breast invasive carcinoma samples.Furthermore,RF,XGBoost,LightGBM,and CatBoost models were established to mine the protein-coding gene markers related to the prognosis of breast invasive cancer and the model performance was compared.The expression data of breast cancer from the Gene Expression Omnibus was used for validation. Results A total of 151 differentially expressed protein-coding genes related to survival prognosis were screened out.The machine learning model established with C3orf80,UGP2,and SPC25 demonstrated the best performance. Conclusions Three protein-coding genes (UGP2,C3orf80,and SPC25) were screened out to identify breast invasive carcinoma.This study provides a new direction for the treatment and diagnosis of breast invasive carcinoma.
更多查看译文
关键词
breast invasive carcinoma,biomarker,protein-coding genes,UGP2,C3orf80,SPC25
AI 理解论文
溯源树
样例
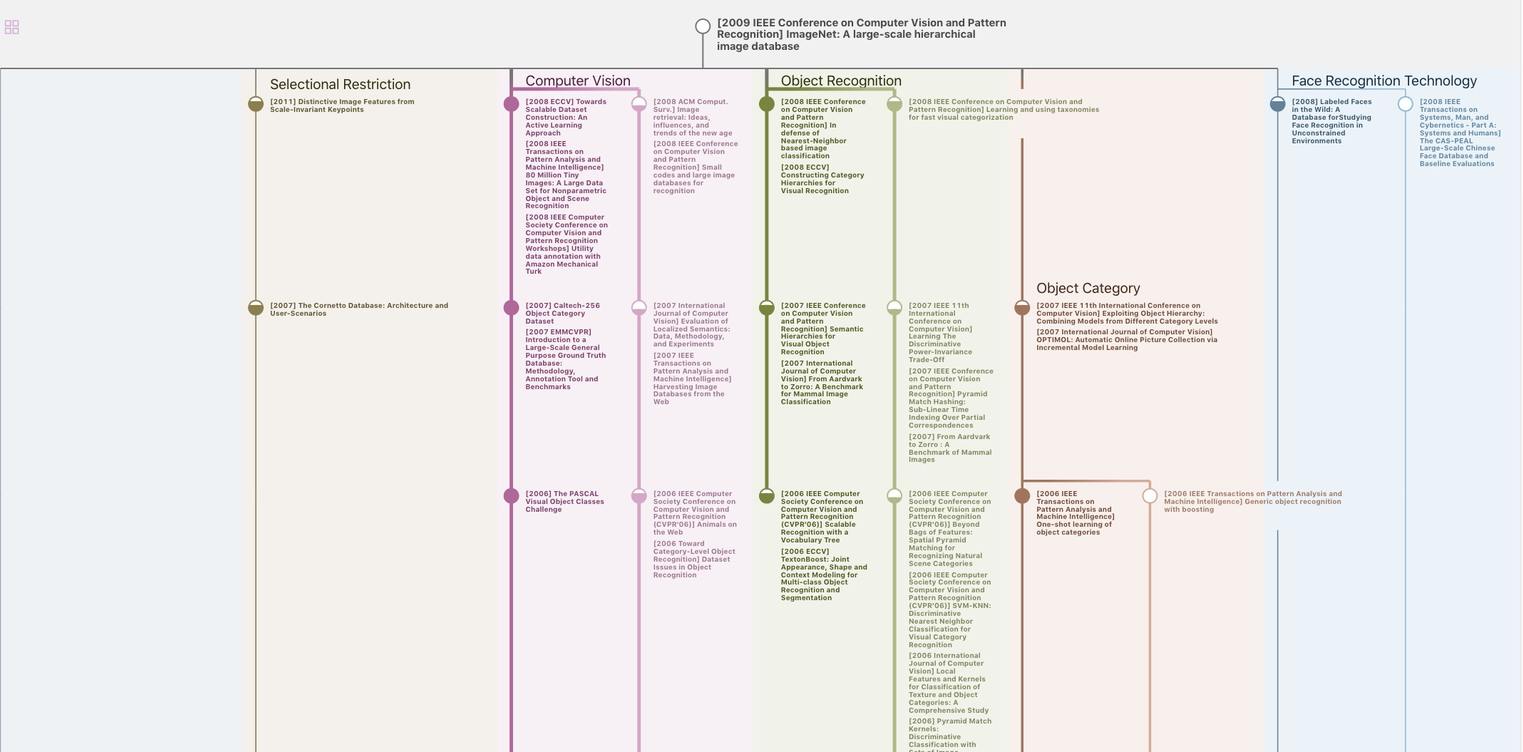
生成溯源树,研究论文发展脉络
Chat Paper
正在生成论文摘要