Optimized model architectures for deep learning on genomic data.
Communications biology(2024)
摘要
The success of deep learning in various applications depends on task-specific architecture design choices, including the types, hyperparameters, and number of layers. In computational biology, there is no consensus on the optimal architecture design, and decisions are often made using insights from more well-established fields such as computer vision. These may not consider the domain-specific characteristics of genome sequences, potentially limiting performance. Here, we present GenomeNet-Architect, a neural architecture design framework that automatically optimizes deep learning models for genome sequence data. It optimizes the overall layout of the architecture, with a search space specifically designed for genomics. Additionally, it optimizes hyperparameters of individual layers and the model training procedure. On a viral classification task, GenomeNet-Architect reduced the read-level misclassification rate by 19%, with 67% faster inference and 83% fewer parameters, and achieved similar contig-level accuracy with ~100 times fewer parameters compared to the best-performing deep learning baselines.
更多查看译文
AI 理解论文
溯源树
样例
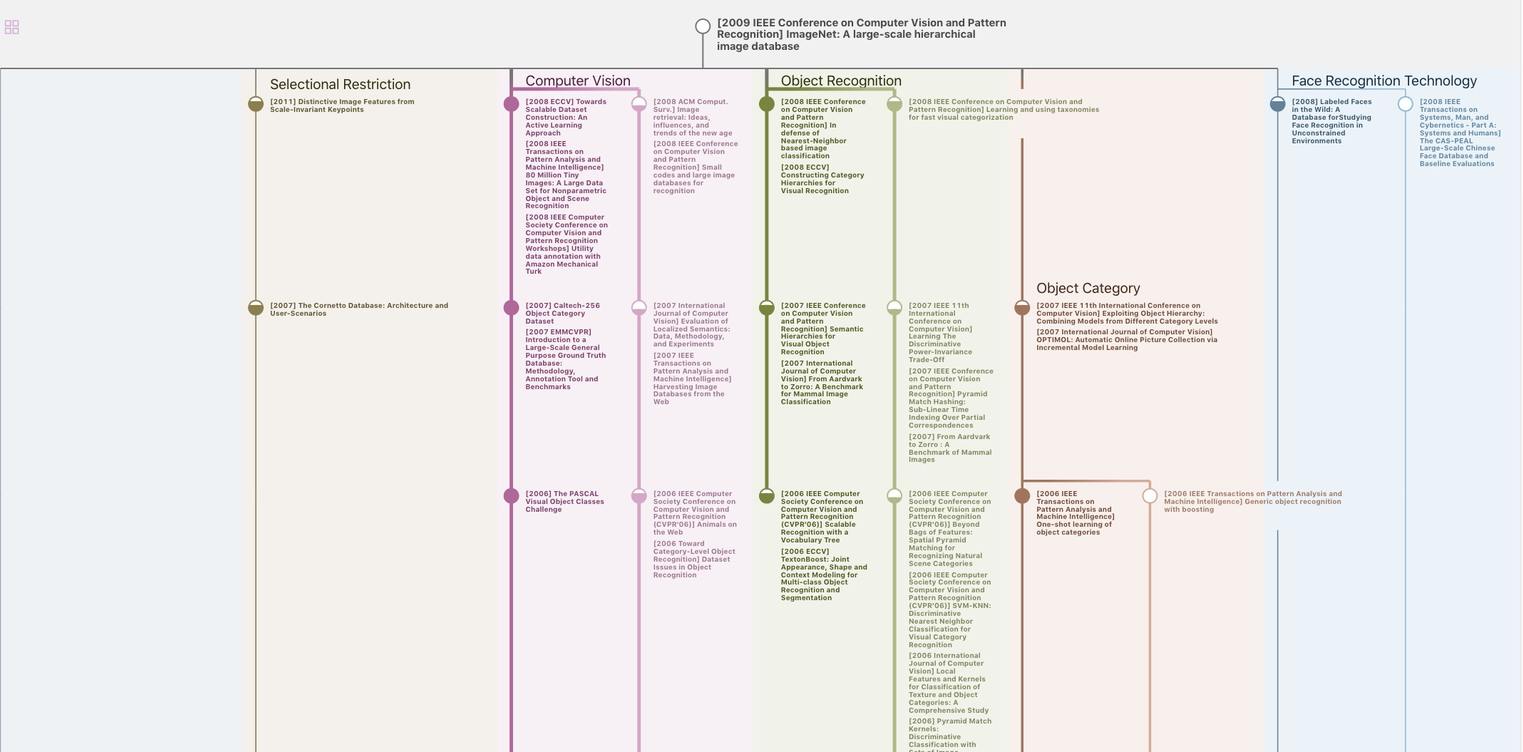
生成溯源树,研究论文发展脉络
Chat Paper
正在生成论文摘要