Learning Approximate Message Passing MIMO Detector for Multi-Scenarios
2023 9th International Conference on Computer and Communications (ICCC)(2023)
摘要
Message passing algorithms (MPAs) have been widely investigated in massive multiple-input multiple-output (MIMO) detectors due to their low complexity and guaranteed performance. Meanwhile, deep learning (DL) has been introduced to further enhance MPAs' performance. In this paper, a model-driven detector called approximate message passing with parameter learning (PL-AMP) is proposed. Specifically, the PL-AMP is obtained by simplifying the Bayesian approximate message passing (BAMP) meanwhile introducing the learnable parameters to compensate for approximation. With only one offline training process, PL-AMP presents superior performance and low complexity across various scenarios as compared to state-of-the-art (SOA) detectors. Also, the robustness of PL-AMP is illustrated, highlighting its ability to adapt to various scenarios.
更多查看译文
AI 理解论文
溯源树
样例
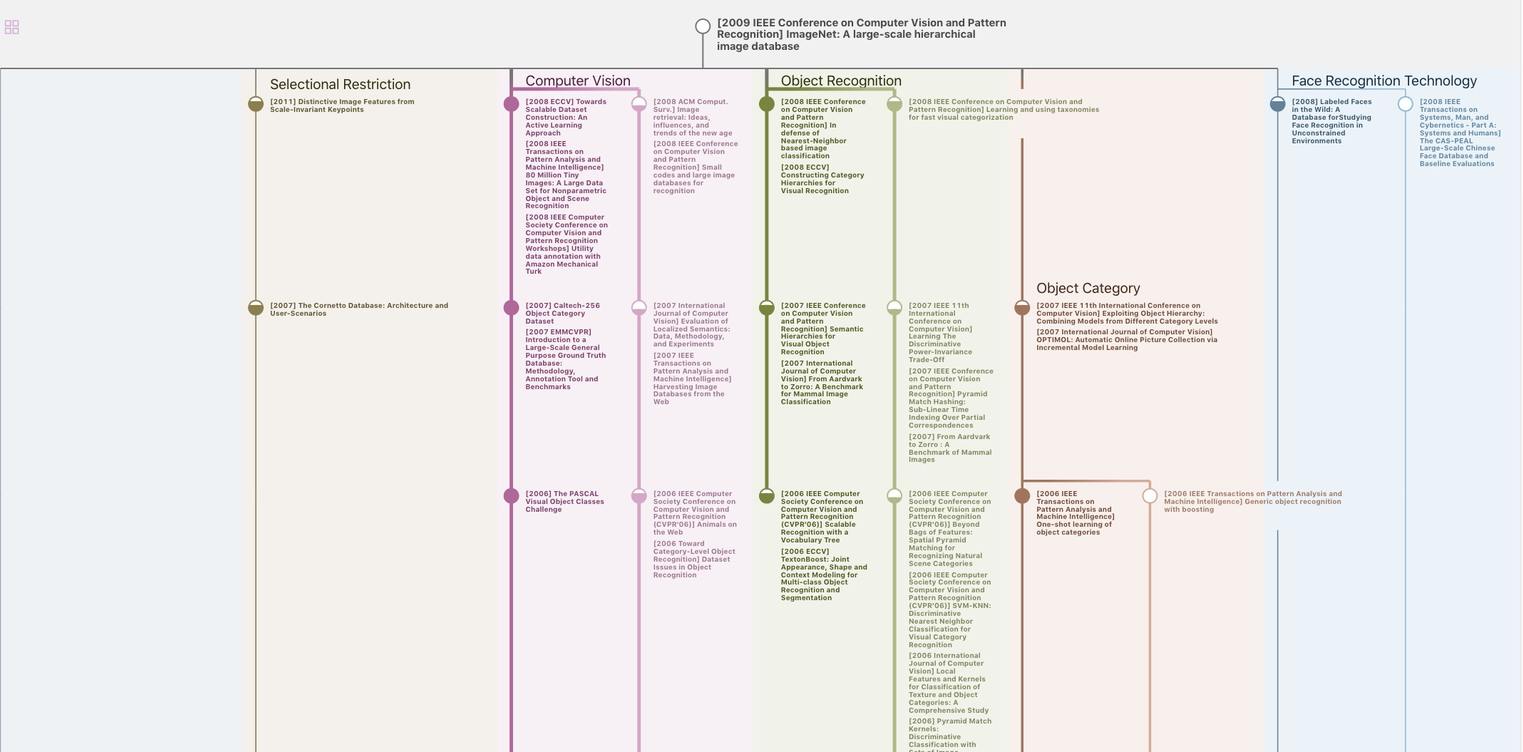
生成溯源树,研究论文发展脉络
Chat Paper
正在生成论文摘要