EM-CNN-BiLSTM: Causality extraction based on Electra and Dual-Stage Attention Mechanism
2023 9th International Conference on Computer and Communications (ICCC)(2023)
Abstract
Event causality extraction (ECE) is the task of extracting event-causal pairs and their structural event information from plain text. However, the accuracy of implicit causal relationship extraction in ECE is low, and how to fully mine the causal semantic relationship within sentences is still a problem in the case of limited samples. Therefore, this paper proposes a causality extraction model (EM-CNN-BiLSTM) based on Electra and dual network fusion. First, the input text sequence is converted into a word embedding vector to obtain the word vector representation of the text. Subsequently, the feature information is generated by a dual-network fusion model, which includes two TextCNN convolutional neural networks for capturing local features, and a bidirectional long-short-term memory network (BiLSTM) for capturing contextual information of sentences. Finally, the context vector is regularized with the dropout technique and mapped to the final classification result using a linear layer. In order to further improve the accuracy of causality extraction, a dual-stage attention mechanism based on features and timing (DAM, dual-stage attention mechanism) is introduced, which are channel attention mechanism and timing attention mechanism respectively, to enhance the model's understanding of important features and the ability to extract historical key information. Through a large number of experimental verifications on the public dataset SemEval-CE, the results show the effectiveness of the model in the task of causality extraction.
MoreTranslated text
AI Read Science
Must-Reading Tree
Example
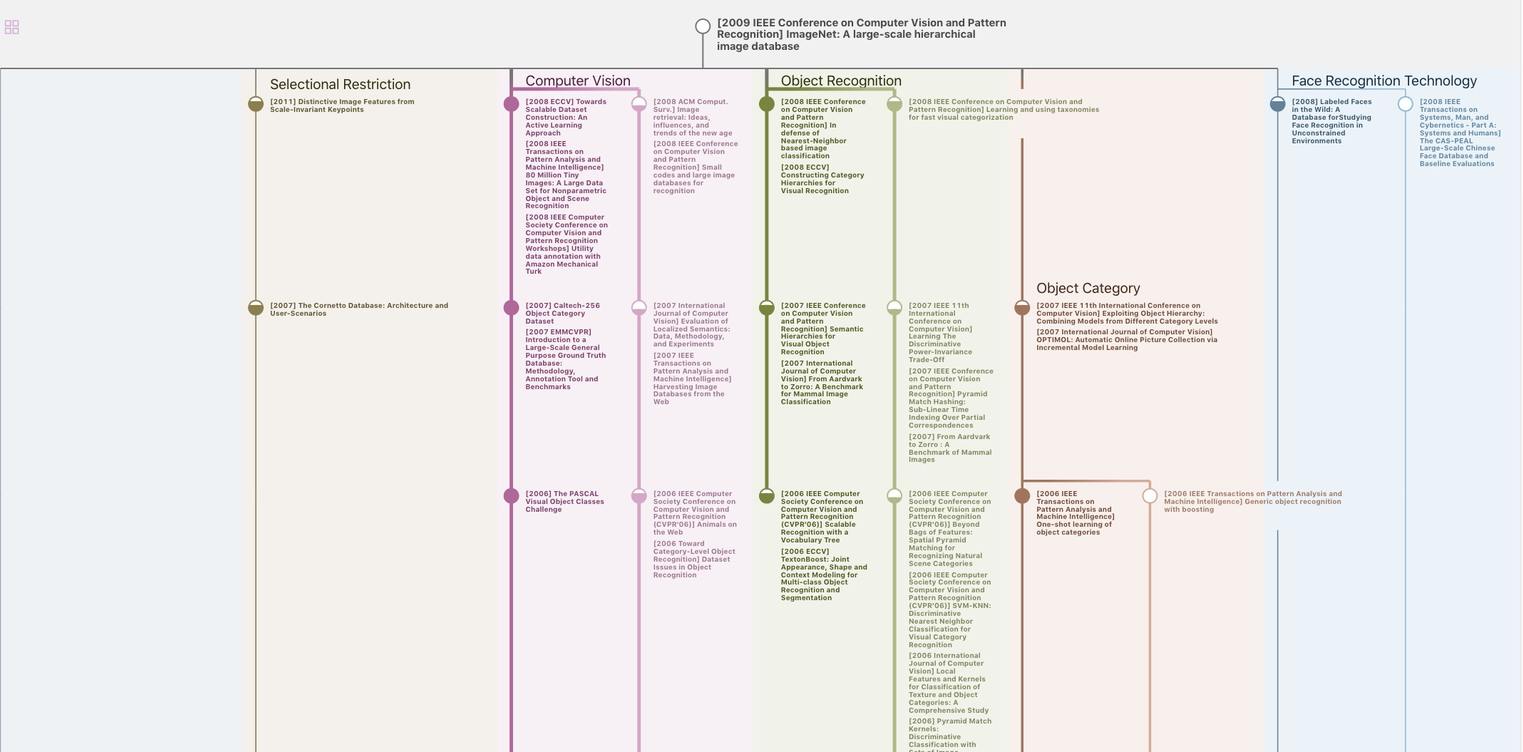
Generate MRT to find the research sequence of this paper
Chat Paper
Summary is being generated by the instructions you defined