A Linguistically Interpretable Deep Fuzzy Classification System with Feature Transformation and Reconstruction
IEEE Transactions on Fuzzy Systems(2024)
摘要
Classification tasks involving tabular data often require a balance between exceptional performance and heightened interpretability. To address this challenge, we propose a linguistically interpretable deep fuzzy classification system called FFT-FFR-RBFC. The system employs a Fuzzy Feature Transformation (FFT) unit, formed by employing a stacked architecture of multiple Takagi-Sugeno-Kang (TSK) fuzzy models with non-linear conclusions, to distill high-level fuzzy features from the input data, a Rule-Based Fuzzy Classifier (RBFC) unit to perform classification using these features, while a Fuzzy Feature Reconstruction (FFR) unit in tandem with the FFT to enhance the system's linguistic interpretability by remapping the high-level features back to their original space. The proposed approach is optimized by minimizing a composite loss function that balances classification and reconstruction losses, ensuring a harmonious interplay between performance and interpretability. Comprehensive evaluation across 20 diverse datasets demonstrates that the system's is exceptionally promising, particularly for high-dimensional or large-scale tabular data classification tasks, achieving superior classification performance while maintaining a high degree of interpretability.
更多查看译文
关键词
Deep fuzzy classification system,TSK model,Feature transformation and reconstruction,Linguistic interpretability
AI 理解论文
溯源树
样例
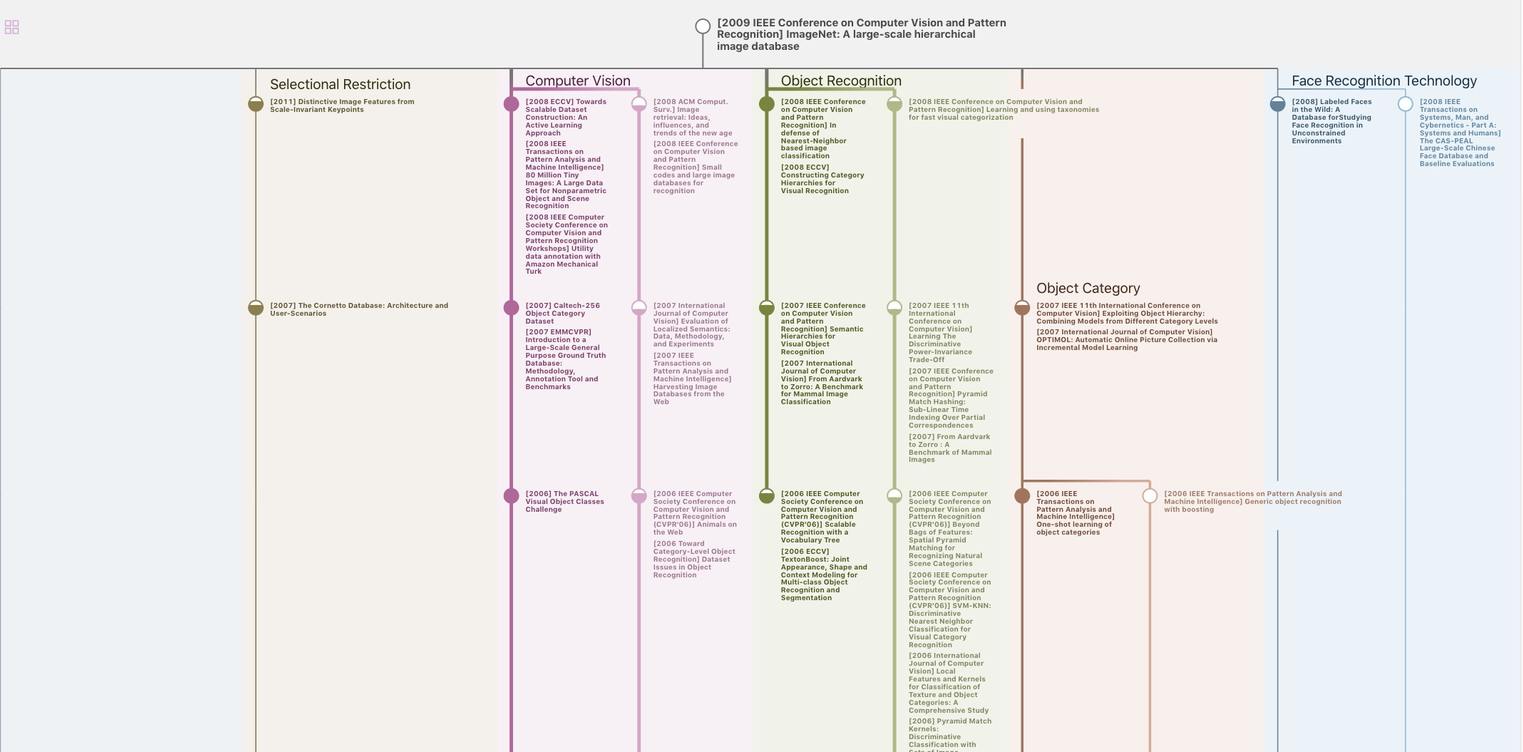
生成溯源树,研究论文发展脉络
Chat Paper
正在生成论文摘要