Collaborative Control Method of Transit Signal Priority Based on Cooperative Game and Reinforcement Learning
CoRR(2024)
摘要
To address the low efficiency in priority signal control within intelligent
transportation systems, this study introduces a novel eight-phase priority
signal control method, CBQL-TSP, leveraging a hybrid decision-making framework
that integrates cooperative game theory and reinforcement learning. This
approach conceptualizes the allocation of bus signal priorities as a
multi-objective decision-making problem across an eight-phase signal sequence,
differentiating between priority and non-priority phases. It employs a
cooperative game model to facilitate this differentiation. The developed hybrid
decision-making algorithm, CBQL, effectively tackles the multi-objective
decision-making challenges inherent in the eight-phase signal sequence. By
computing the Shapley value function, it quantifies the marginal contributions
of each participant, which in turn inform the construction of a state
transition probability equation based on Shapley value ratios. Compared to
conventional control methods, the CBQL-TSP method not only upholds the fairness
principles of cooperative game theory but also harnesses the adaptive learning
capabilities of Q-Learning. This enables dynamic adjustments to signal timing
in response to real-time traffic conditions, significantly enhancing the
flexibility and efficiency of priority signal control.
更多查看译文
AI 理解论文
溯源树
样例
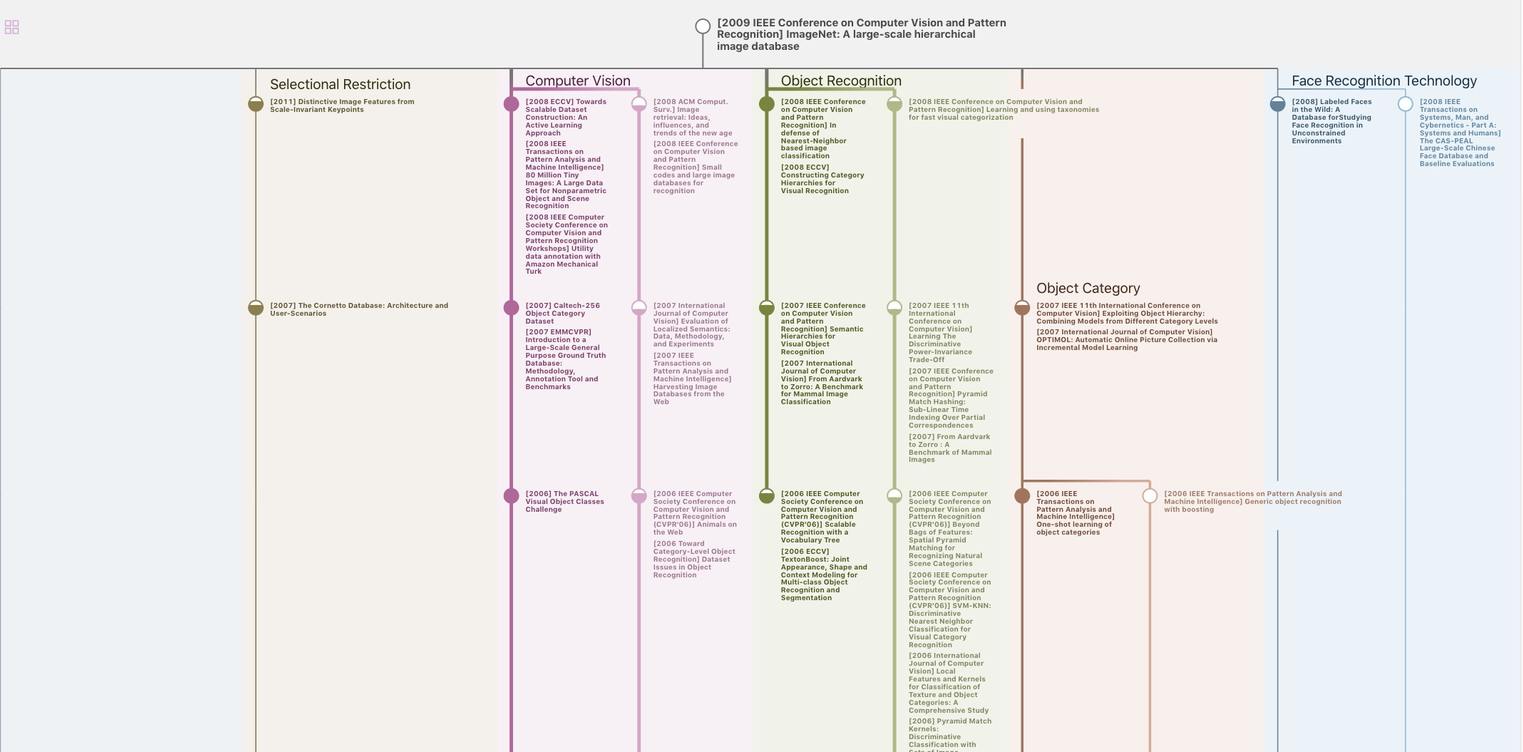
生成溯源树,研究论文发展脉络
Chat Paper
正在生成论文摘要