Variational approximations of possibilistic inferential models
arxiv(2024)
摘要
Inferential models (IMs) offer reliable, data-driven, possibilistic
statistical inference. But despite IMs' theoretical/foundational advantages,
efficient computation in applications is a major challenge. This paper presents
a simple and apparently powerful Monte Carlo-driven strategy for approximating
the IM's possibility contour, or at least its α-level set for a
specified α. Our proposal utilizes a parametric family that, in a
certain sense, approximately covers the credal set associated with the IM's
possibility measure, which is reminiscent of variational approximations now
widely used in Bayesian statistics.
更多查看译文
AI 理解论文
溯源树
样例
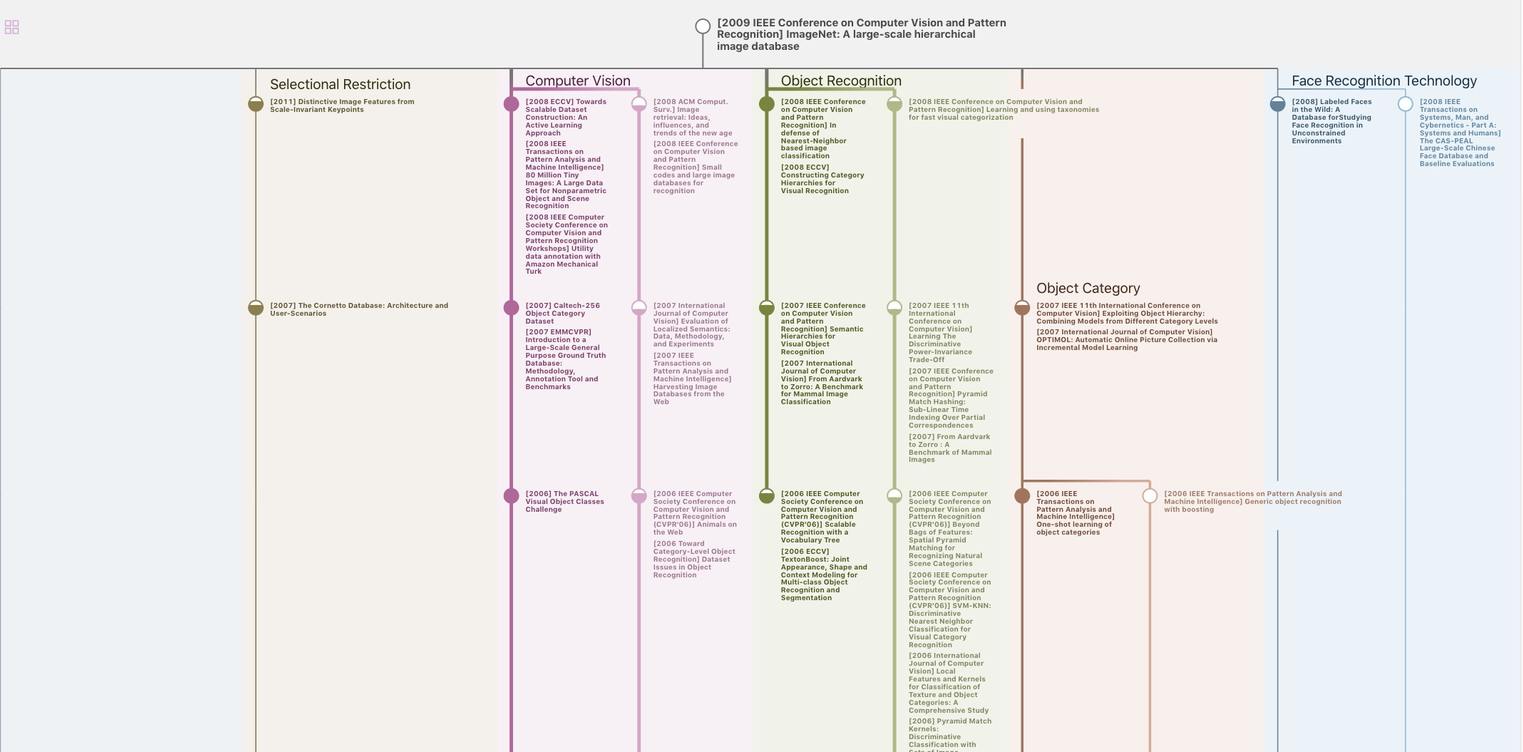
生成溯源树,研究论文发展脉络
Chat Paper
正在生成论文摘要