Inexact subgradient methods for semialgebraic functions
arxiv(2024)
Abstract
Motivated by the widespread use of approximate derivatives in machine
learning and optimization, we study inexact subgradient methods with
non-vanishing additive errors and step sizes. In the nonconvex semialgebraic
setting, under boundedness assumptions, we prove that the method provides
points that eventually fluctuate close to the critical set at a distance
proportional to ϵ^ρ where ϵ is the error in subgradient
evaluation and ρ relates to the geometry of the problem. In the convex
setting, we provide complexity results for the averaged values. We also obtain
byproducts of independent interest, such as descent-like lemmas for nonsmooth
nonconvex problems and some results on the limit of affine interpolants of
differential inclusions.
MoreTranslated text
AI Read Science
Must-Reading Tree
Example
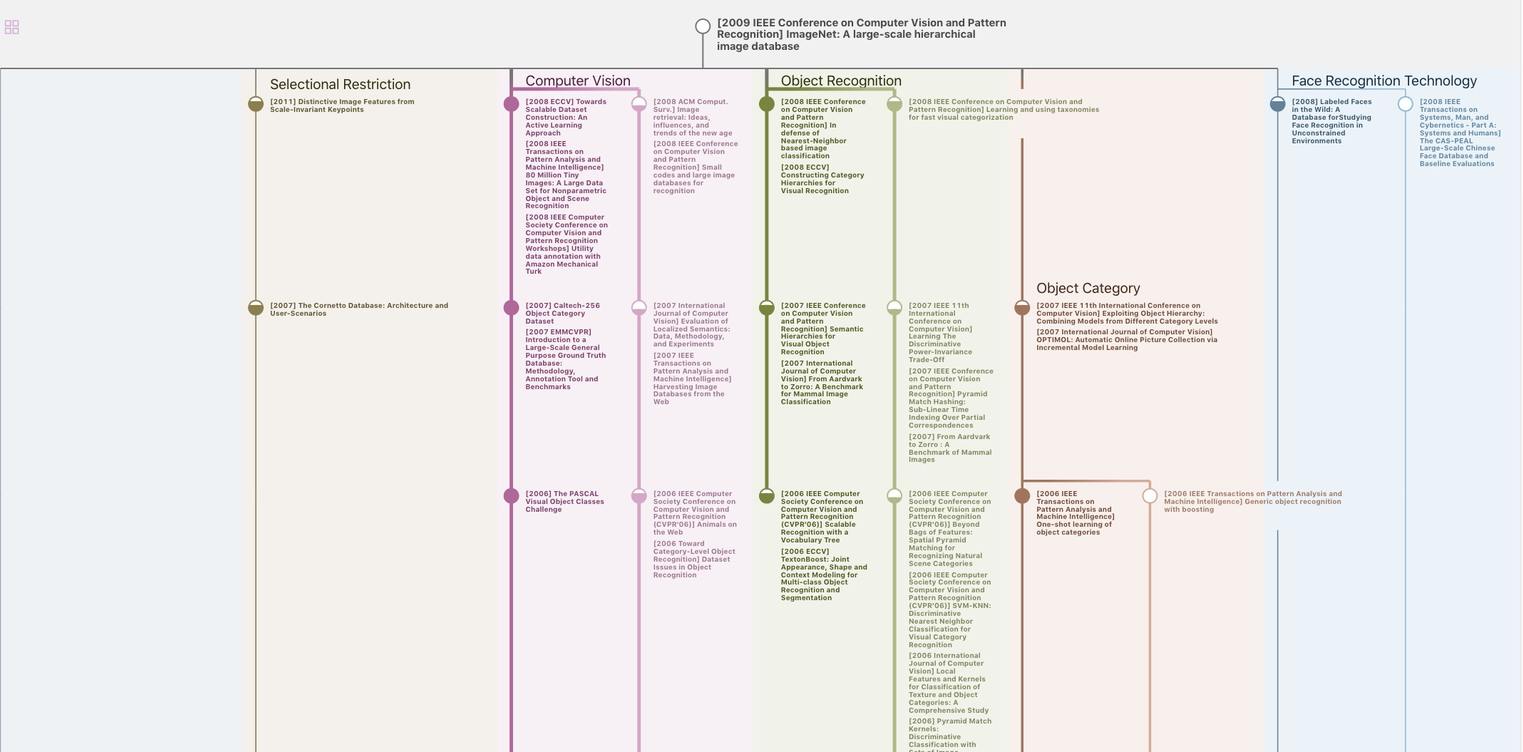
Generate MRT to find the research sequence of this paper
Chat Paper
Summary is being generated by the instructions you defined