Traffic trajectory generation via conditional generative adversarial networks for transportation metaverse
Applied Soft Computing(2024)
摘要
The transportation Metaverse, by integrating real and virtual vehicular networks, brings significant benefits to the development of smart cities. However, the difficulty and high cost of conducting large-scale traffic and driving simulations in the transportation Metaverse via realistic data collection and fusion from the physical world directly result in the lack of spatial–temporal traffic data and privacy concerns, which significantly hinder the development of the transportation Metaverse. Hence, in these situations, it becomes essential to produce high-quality, large-scale trajectory data to support relevant applications. However, the deficiency of data-driven method is the heavy dependence on high-quality historical data. Prior knowledge can help reduce learning difficulties and overfitting problems with small amounts of data. In our study, we use a hybrid framework, the Travel Demand Conditioning Generative Adversarial Network (TD-GAN), which combines data-driven and knowledge-driven approaches to address the issue of traffic trajectory generation. First, we employ a conditional mechanism to incorporate prior knowledge of travel demand to reduce learning difficulties. Second, non-standard convolutional module and multi-headed self-attention module are developed to capture spatial–temporal correlations. The experimental results show that our model outperforms the baseline models.
更多查看译文
关键词
Transportation metaverse,Spatial–temporal data,Traffic trajectory generation,Prior knowledge,Conditional adversarial generative networks
AI 理解论文
溯源树
样例
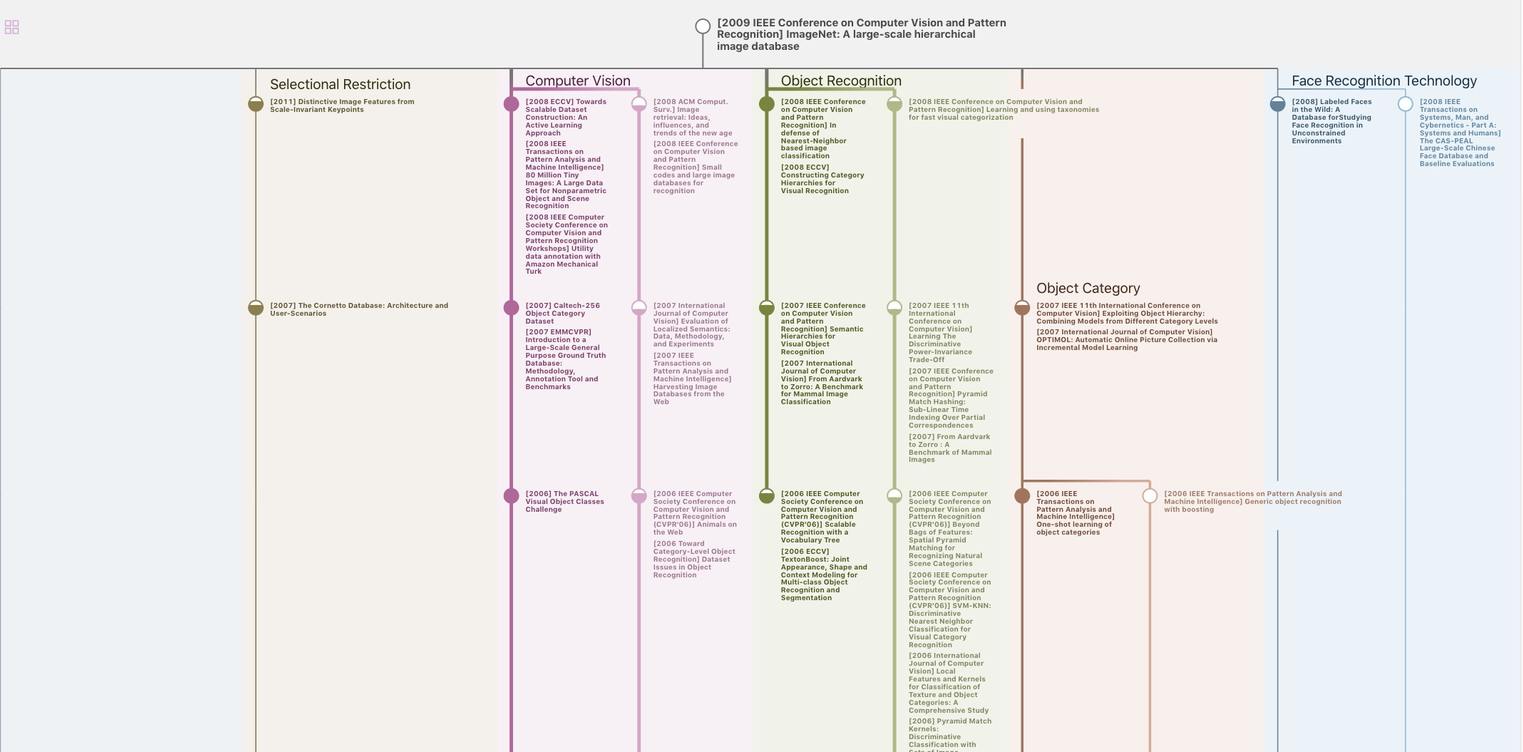
生成溯源树,研究论文发展脉络
Chat Paper
正在生成论文摘要