A Trans-Ptr-Nets Based Transfer Optimization Method for Multi-Objective Flexible Job Shop Scheduling in IIoT
IEEE Internet of Things Journal(2024)
摘要
Industrial Internet-of-things (IIoT) is considered an emerging infrastructure for enhancing manufacturing efficiency by facilitating the sharing of resources across multiple factories. With increasing requirements on customized production in IIoT, the tasks and objectives of the flexible job shop scheduling problem for different orders vary greatly, leading to repetitive algorithm adjustment and time-consuming solver invocation. To accelerate the efficiency for the production orders in different scheduling scenarios, this paper proposed a transfer optimization method based on pointer networks improved by Transformer (Trans-Ptr-Nets). A historical solution selection strategy accompanied with a historical solution dataset to retrieve solutions similar to the current scenario are established. Then, the Trans-Ptr-Nets is designed to transfer the candidate solutions to new solutions that are feasible to the target scheduling scenario. Subsequently, the new solutions are introduced as the additional new population of evolutionary algorithm to accelerate the optimization process. Experimental results conducted on four transfer scenarios show that the proposed method can realize at most 50% reduction in running time while improving the solution quality by at least 10%, compared with six typical evolutionary algorithms and three typical transfer learning networks.
更多查看译文
关键词
Industrial Internet-of-things,flexible job shop scheduling problem,transfer optimization
AI 理解论文
溯源树
样例
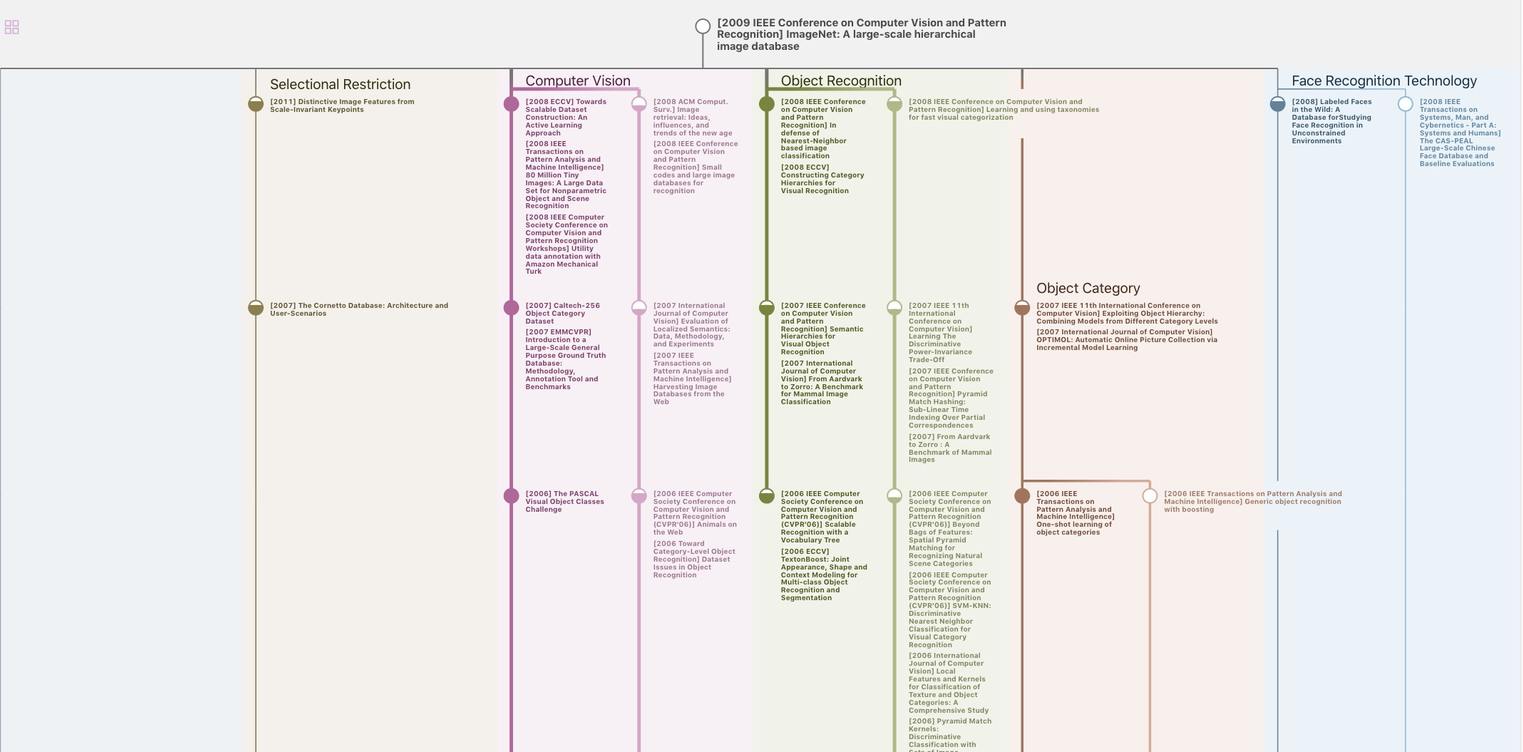
生成溯源树,研究论文发展脉络
Chat Paper
正在生成论文摘要