Label disambiguation-based feature selection for partial label learning via fuzzy dependency and feature discernibility
Applied Soft Computing(2024)
Abstract
Partial label learning is a multi-class classification issue in which each training instance is associated with a set of candidate labels. Feature selection is an effective method to improve the performance of the learning model, and at the same time, feature selection being a challenging problem in partial label learning due to the label ambiguity of partial labels. To tackle this challenge, this paper proposes a label disambiguation-based feature selection for partial label learning via fuzzy dependency and feature discernibility. Specifically, considering the high sensitivity of the fuzzy rough sets model to pseudo labels, a instance distribution-based label disambiguation method is presented to reduce the noise from the candidate labels. Based on this, a weighted fuzzy rough sets model is constructed in accordance with the distribution information of labels, and the function of fuzzy dependency is redefined. Then, the evaluation function of feature significance is obtained by fusing fuzzy dependency and feature discernibility for identifying the critical features. Finally, extensive experiments have confirmed the feasibility and effectiveness of the proposed method. Compared to other feature selection algorithms, the proposed method exhibits superior performance and enhances the generalization of partial label learning models. Furthermore, the feasibility of the proposed label disambiguation method is demonstrated through comparison with state-of-the-art label disambiguation methods.
MoreTranslated text
Key words
Feature selection,Fuzzy rough sets,Granular computing,Label disambiguation,Fuzzy dependency
AI Read Science
Must-Reading Tree
Example
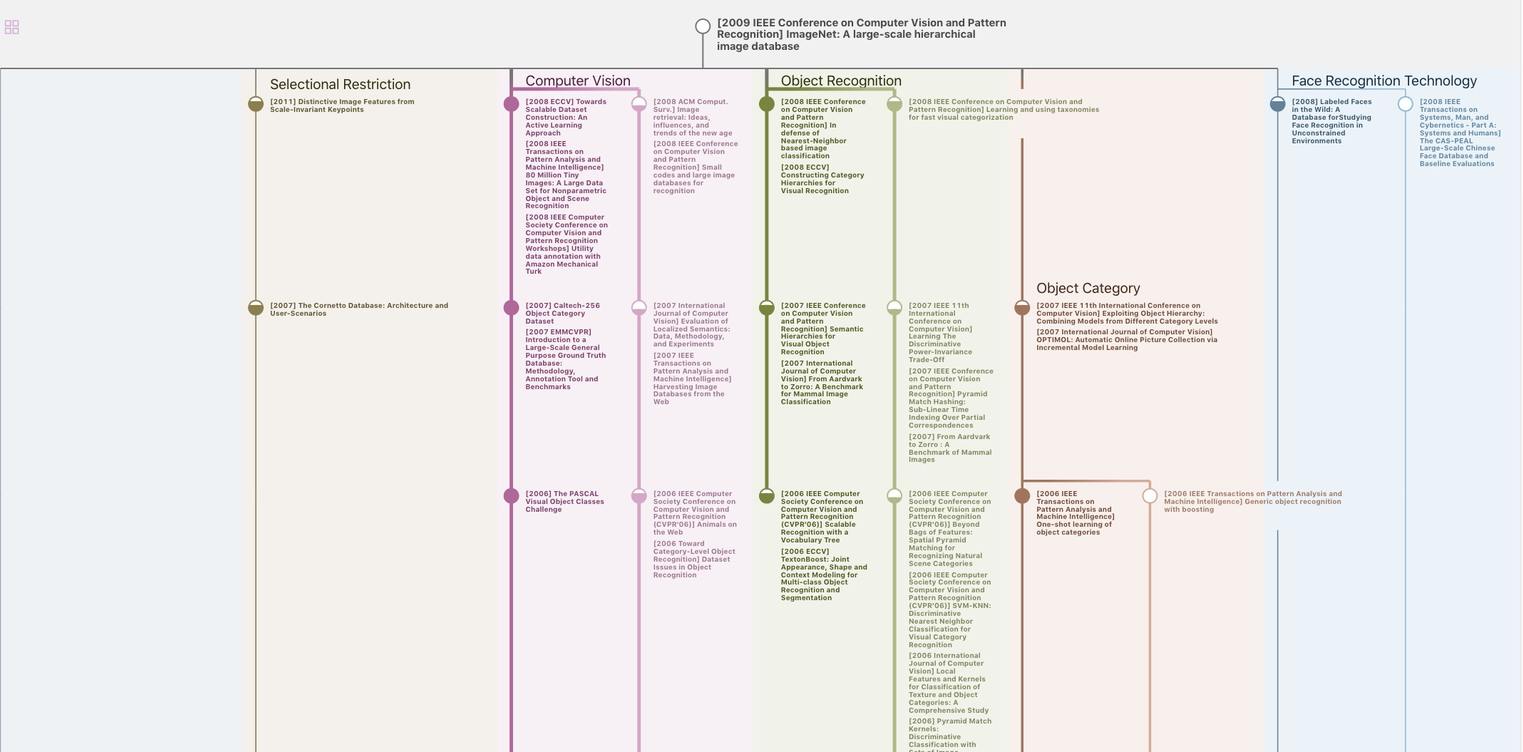
Generate MRT to find the research sequence of this paper
Chat Paper
Summary is being generated by the instructions you defined