Hypertension Trends and Disparities Over 12 Years in a Large Health System: Leveraging the Electronic Health Records.
Journal of the American Heart Association(2024)
Abstract
BACKGROUND:The digital transformation of medical data enables health systems to leverage real-world data from electronic health records to gain actionable insights for improving hypertension care.
METHODS AND RESULTS:We performed a serial cross-sectional analysis of outpatients of a large regional health system from 2010 to 2021. Hypertension was defined by systolic blood pressure ≥140 mm Hg, diastolic blood pressure ≥90 mm Hg, or recorded treatment with antihypertension medications. We evaluated 4 methods of using blood pressure measurements in the electronic health record to define hypertension. The primary outcomes were age-adjusted prevalence rates and age-adjusted control rates. Hypertension prevalence varied depending on the definition used, ranging from 36.5% to 50.9% initially and increasing over time by ≈5%, regardless of the definition used. Control rates ranged from 61.2% to 71.3% initially, increased during 2018 to 2019, and decreased during 2020 to 2021. The proportion of patients with a hypertension diagnosis ranged from 45.5% to 60.2% initially and improved during the study period. Non-Hispanic Black patients represented 25% of our regional population and consistently had higher prevalence rates, higher mean systolic and diastolic blood pressure, and lower control rates compared with other racial and ethnic groups.
CONCLUSIONS:In a large regional health system, we leveraged the electronic health record to provide real-world insights. The findings largely reflected national trends but showed distinctive regional demographics and findings, with prevalence increasing, one-quarter of the patients not controlled, and marked disparities. This approach could be emulated by regional health systems seeking to improve hypertension care.
MoreTranslated text
Key words
health systems,hypertension prevalence,racial disparities,real‐world data
AI Read Science
Must-Reading Tree
Example
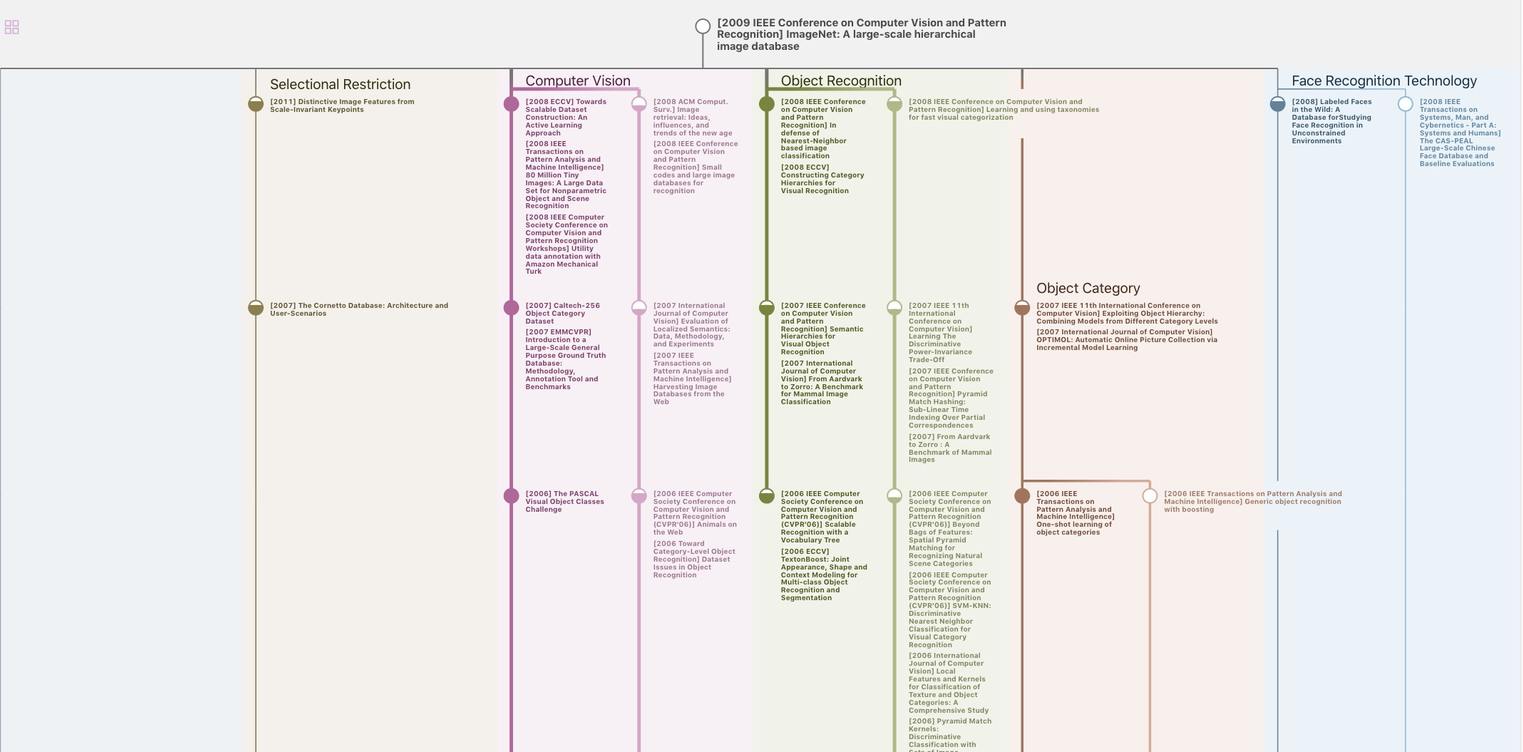
Generate MRT to find the research sequence of this paper
Chat Paper
Summary is being generated by the instructions you defined