Towards eXplainabile Data-Driven Control (XDDC): The Property-Preserving Framework
IEEE Control Systems Letters(2024)
摘要
As Artificial Intelligence (AI) techniques continue to advance, the need for explainability becomes increasingly crucial, especially in sensitive or safety-critical domains. eXplainable AI (XAI) has emerged to address this need, aiming to enhance transparency in complex models. While XAI has gained traction in mainstream machine learning, its application in data-driven control systems remains relatively unexplored. This paper introduces a novel concept of explainability tailored for data-driven control, allowing one to design feedback loops from data incorporating prior knowledge and preserving important system properties. Through two case studies, we demonstrate the efficacy of this property-preserving framework in direct and indirect data-driven control system design. This work lays the foundation for further research at the intersection of AI and data-driven control, offering insights into enhancing transparency in complex control systems.
更多查看译文
关键词
Data driven control,Machine Learning,Identification for control
AI 理解论文
溯源树
样例
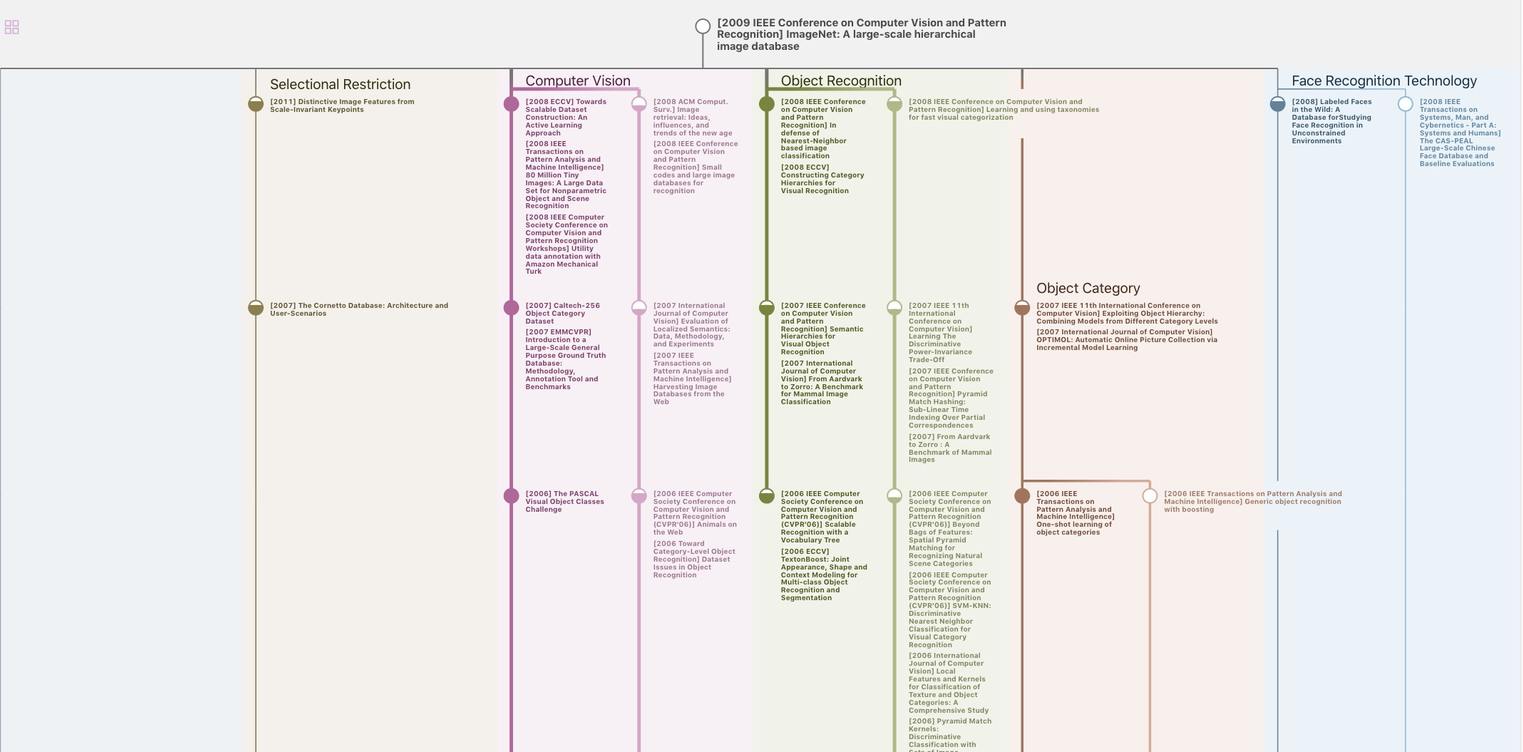
生成溯源树,研究论文发展脉络
Chat Paper
正在生成论文摘要