Unsupervised Homography Estimation with Pixel-level SVDD
IEEE Transactions on Circuits and Systems for Video Technology(2024)
摘要
Homography estimation is a common image alignment method. Unsupervised learning, which uses unlabeled training and exhibits excellent performance, has attracted much attention in this field. When there are multiple planes in the scene, using features over the entire image for matching will lead to compromised results. However, existing methods for learning focused principal plane masks through deep neural networks lack explicit guidance. In this paper, we propose a novel unsupervised method to explicitly model anomaly descriptor removal and mask generation. Specifically, reliable feature descriptors are selected from a novel perspective, and regard the features that are not responsible for alignment as outliers. The pixel-level support vector data description (PL-SVDD) module is designed. This module learns the feature representation of image pixels and fits a hypersphere to exclude the feature redundancy information that is not responsible for alignment from the hypersphere, thereby optimizing the feature descriptor. Based on the optimized image features, a correlation learning (CL) module is designed. This module displays a generated mask through mathematical modeling to select reliable areas for homography estimation. Specifically, the feature descriptor of one unaligned images is modeled as a multivariate Gaussian distribution by Gaussian density estimation (GDE). Then, The Mahalanobis distance is combined with the multivariate Gaussian distribution of the model and the feature descriptor of another image to generate the mask. Experiments show that our method achieves good performance compared with previous methods.
更多查看译文
关键词
Homography estimation,pixel-level SVDD,Gaussian Density Estimation,correlation learning module
AI 理解论文
溯源树
样例
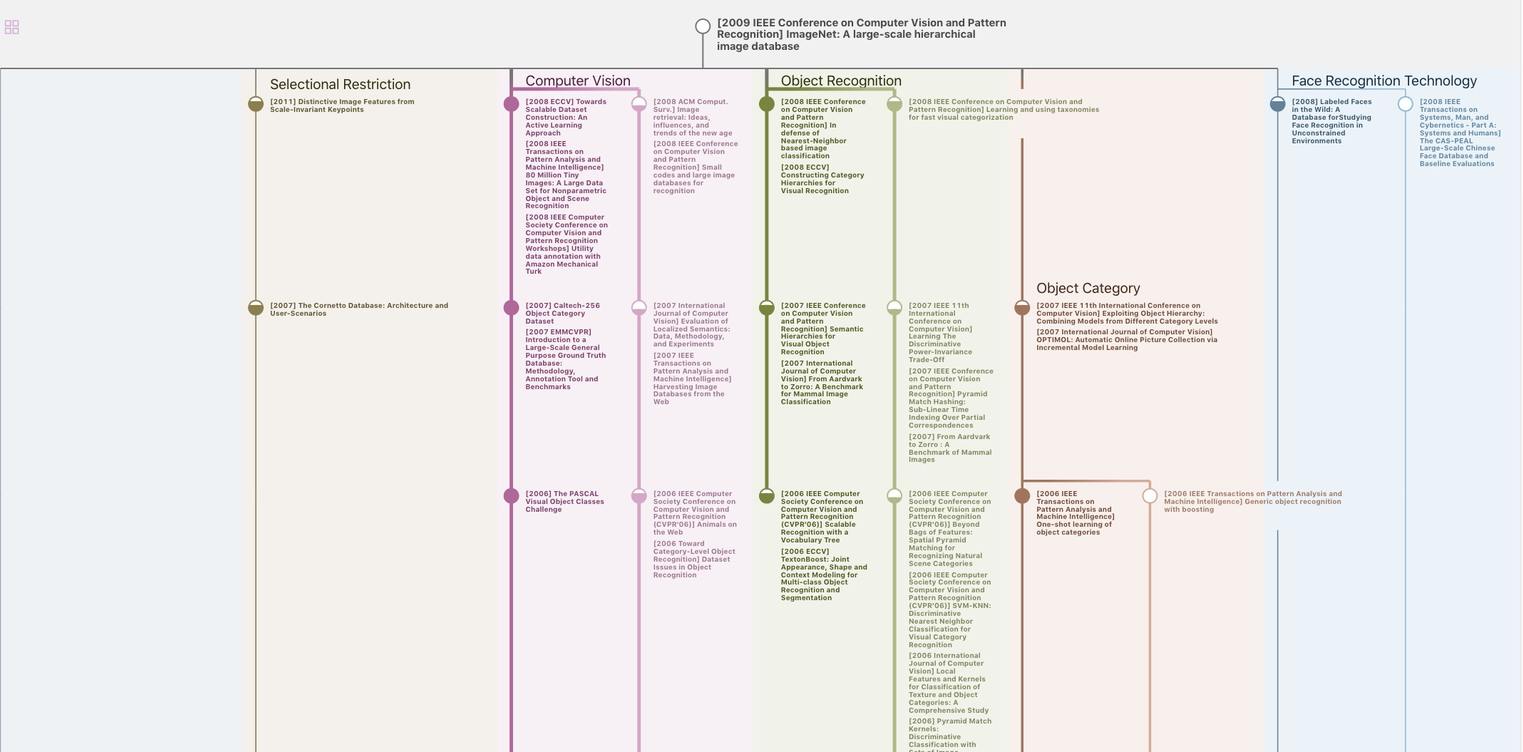
生成溯源树,研究论文发展脉络
Chat Paper
正在生成论文摘要