Addressing Machine Learning Problems in the Non-Negative Orthant
IEEE Transactions on Emerging Topics in Computational Intelligence(2024)
Abstract
Frequently, equality constraints are imposed on the objective function of machine learning algorithms aiming at increasing their robustness and generalization. In addition, non-negativity constraints imposed on the objective function aim to improve interpretability. This paper proposes a framework that solves problems in the non-negative orthant with additional equality constraints. This framework is characterized by an iteration complexity
${\text{\usefont {OMS}{cmsy}{m}{n}O}} {(\lm{\ln}\, \lm{\epsilon} ^{\lm{ -\varrho }})}$
with
$\lm{\epsilon}$
denoting the accuracy and
$\lm{\varrho}$
being the condition number. To avoid “zig-zagging”, a diminishing learning rate is adopted without harming the convergence of the learning procedure. Simple and well-established tools of the theory of Lagrange multipliers for constrained optimization are employed to derive the updating rules and study their convergence properties. To the best of our knowledge, this is the first time these tools are combined in a unified way to derive the proposed optimizer. Its efficiency is demonstrated by conducting classification experiments on well-known datasets, yielding promising results.
MoreTranslated text
Key words
Lagrange multipliers,first-order optimization,convex optimization,constrained optimization,non-negative constraints
AI Read Science
Must-Reading Tree
Example
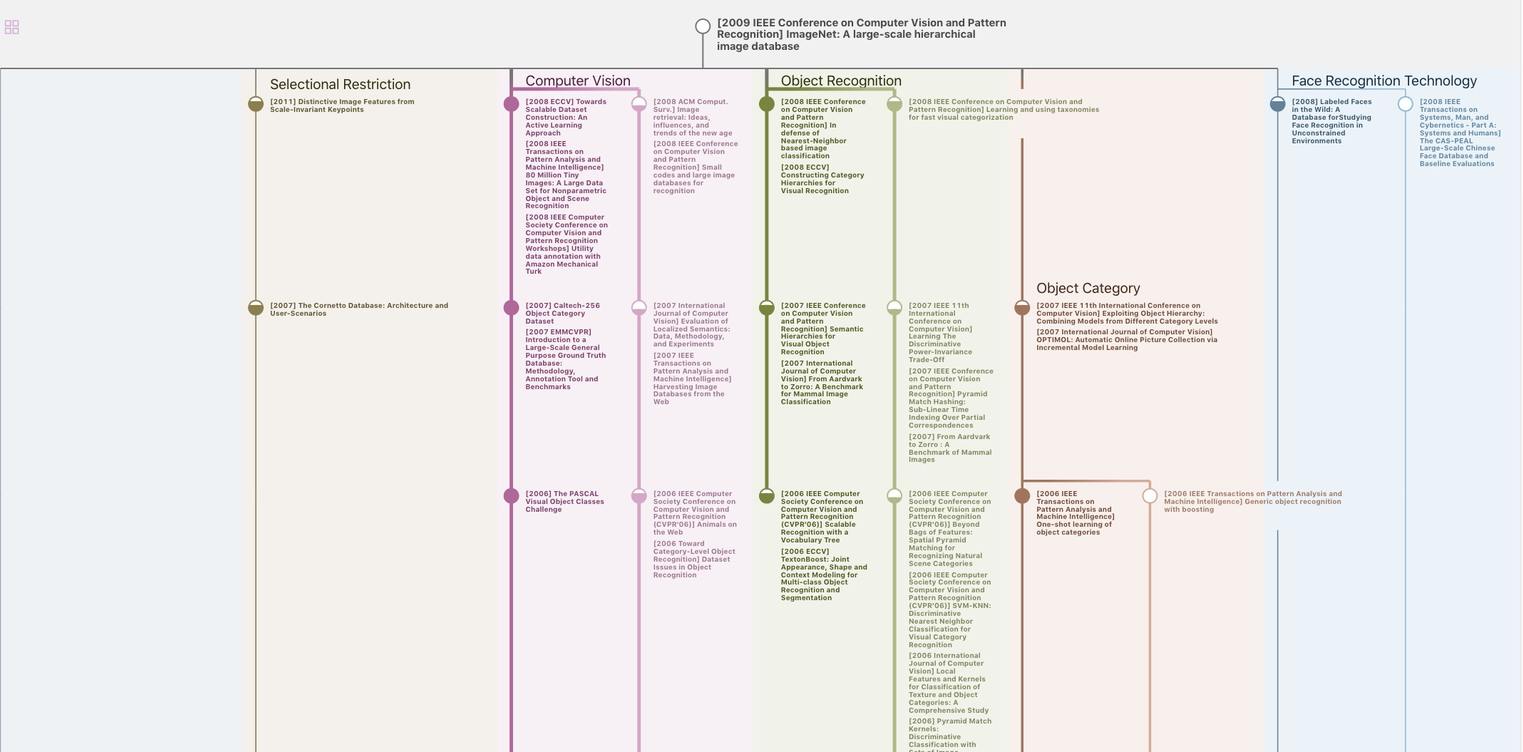
Generate MRT to find the research sequence of this paper
Chat Paper
Summary is being generated by the instructions you defined