Bayesian Direction of Arrival Estimation using Atomic Norm Minimization with Prior Knowledge
IEEE Transactions on Aerospace and Electronic Systems(2024)
摘要
This paper concerns the direction of arrival (DOA) estimation problem in Bayesian framework by using the sparse methods that incorporate the prior knowledge within the array observation data. The obtained prior of knowledge of DOAs is assumed to follow a prior distribution. Considering the unknown DOAs are random variables, we propose two sparse methods by effectively and efficiently exploiting the information from the observation data and prior knowledge. One is a grid-based sparse method using the second-order cone programming (SOCP) by discretizing the grids in the prescribed prior region where the targets occur with high probability. The other is a gridless sparse method using the atomic norm minimization by transforming the prior knowledge into semidefinite constraint. The first is computationally efficient, but it suffers from grid mismatch problem in high SNR. The second further improves the estimation performance with high computational complexity. Simulation results demonstrate the superiority of the proposed methods when comparing with the traditional DOA estimation methods together with the maximum a posterior (MAP) estimator and the Bayesian Cramer- ´ Rao lower bounds (BCRLB)
更多查看译文
关键词
Bayesian DOA estimation,prior knowledge,sparse methods,atomic norm minimization,Toeplitz matrix
AI 理解论文
溯源树
样例
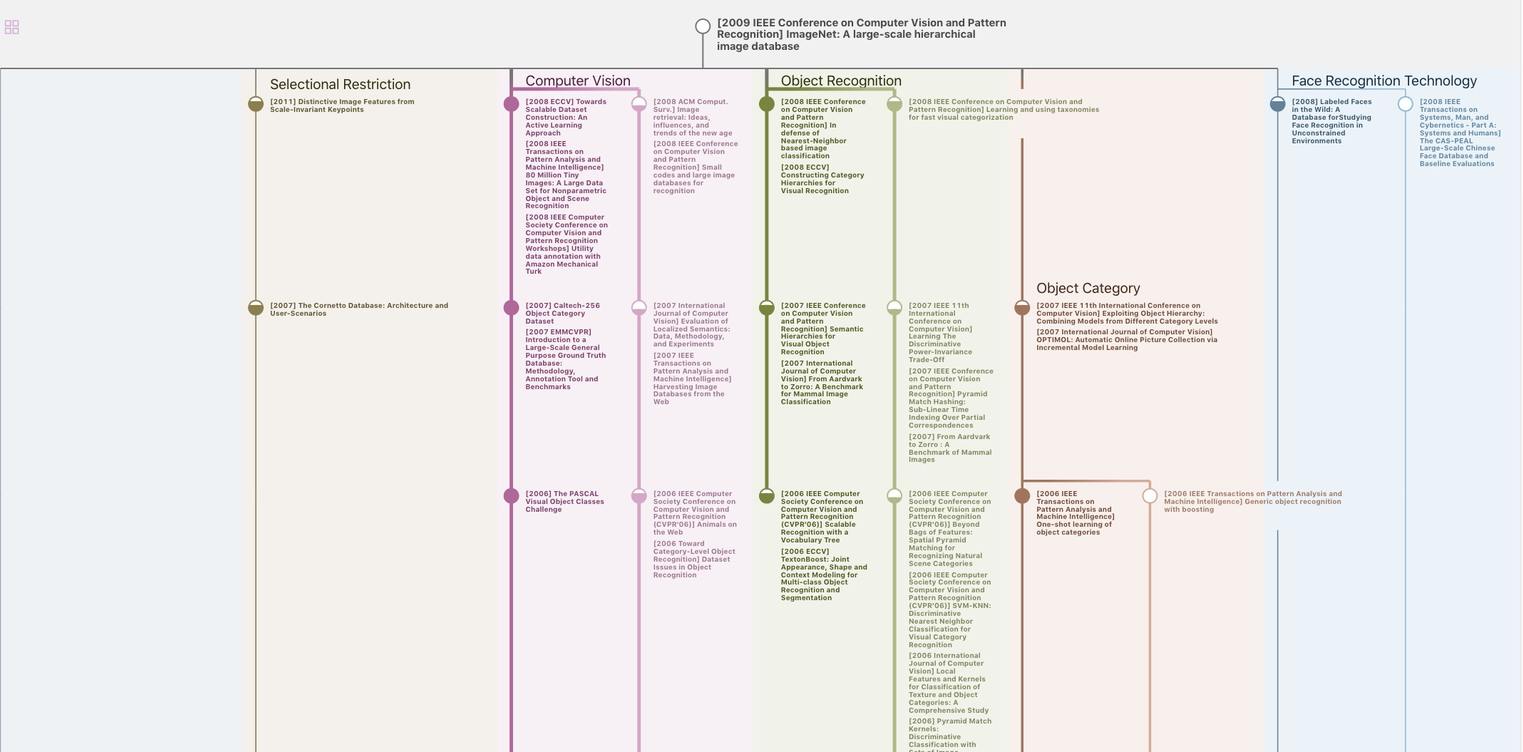
生成溯源树,研究论文发展脉络
Chat Paper
正在生成论文摘要