Local Co-location Pattern Mining Based on Regional Embedding.
International Conference on Spatial Data and Intelligence(2024)
摘要
Local co-location pattern (LCP) presents the spatial correlation between various categories in local regions. Regional partitioning is a pivotal step in LCP mining. Existing regional partitioning methods may ignore potential LCPs due to subjective elements. Additionally, with the diversity of geographic data increases, previous mining techniques disregarded the semantic information within the data, and limited the interpretability of local regions and LCPs. In response to these issues, this paper introduces an approach for LCP mining based on regional embedding. Initially, the entire study region is finely divided into local regions through natural data like road networks. Next, leveraging regional embedding techniques, local regions are embedded using human trajectory events, resulting in the creation of regional embedding vectors. Subsequently, the k -means method is employed to find functional clusters of local regions, and self-attention mechanisms is used for functional annotation. Then, the semantic LCPs are mined in these annotated local regions. Experiments on real-world datasets comprising urban population trajectories and Points of Interest (POI) confirm the efficiency and interpretability of the proposed framework for LCP mining based on regional embedding.
更多查看译文
AI 理解论文
溯源树
样例
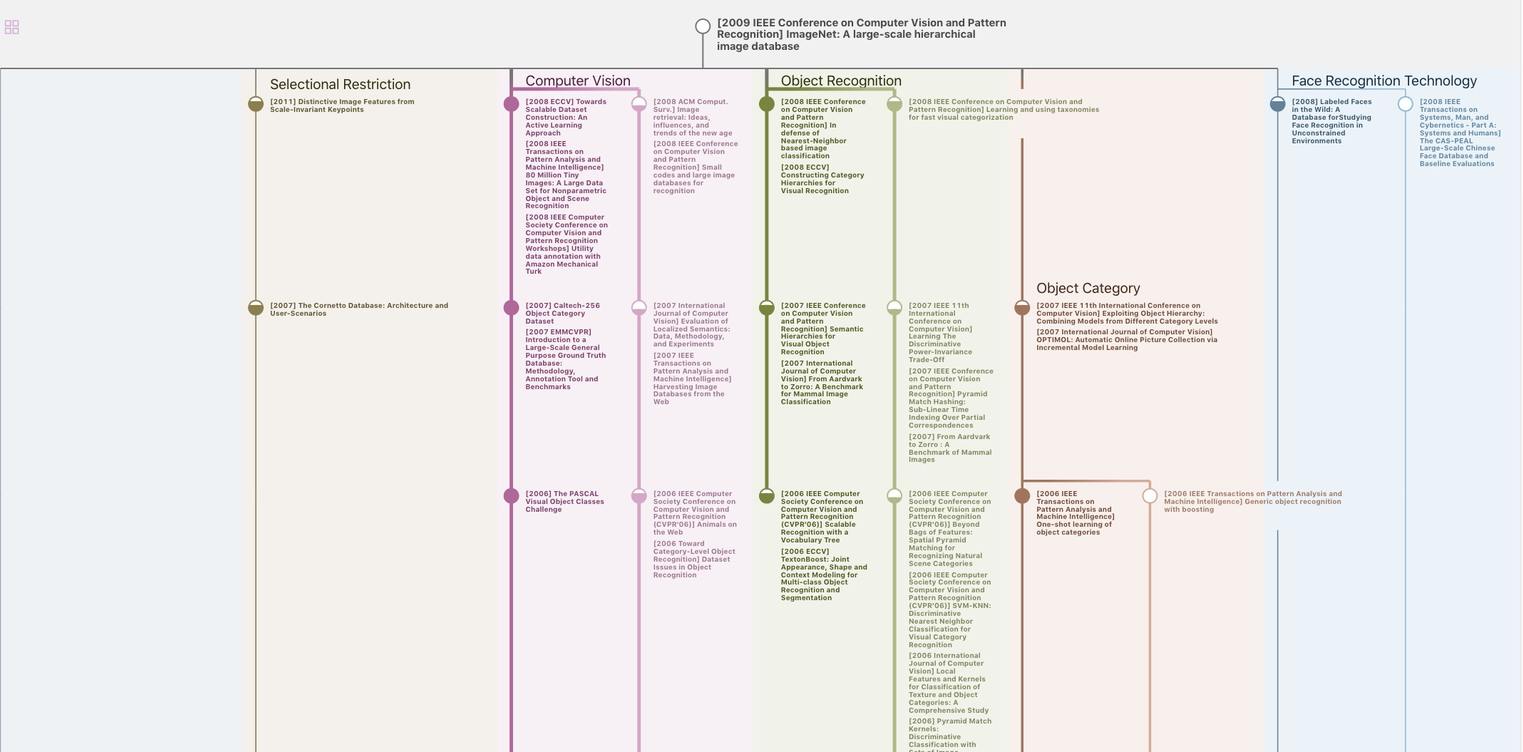
生成溯源树,研究论文发展脉络
Chat Paper
正在生成论文摘要