Prestack Seismic Inversion Driven by Priori Information Neural Network and Statistical Characteristic
IEEE TRANSACTIONS ON GEOSCIENCE AND REMOTE SENSING(2024)
Abstract
Seismic inversion accuracy significantly affects the quality of reservoir modeling. Given the limited samples of well logging, prior information such as geological data and stratigraphic characteristics is crucial for enhancing the accuracy and reliability of inversion results. The inability to fully exploit prior information in the data to compensate for data deficiencies may impair network performance. Moreover, the traditional artificial neural networks (ANNs) cannot fully exploit prior information in the data to compensate for data deficiencies, which may impair network performance and further leads to the network's limited ability to effectively assimilate external prior knowledge. Therefore, a prestack seismic inversion driven by prior information neural network (PINN) and statistical characteristic is proposed to integrate various geological prior information. Initially, lithological analysis is conducted on the well logging data, leading to the classification of the strata into a series of sub-layers based on lithology. After obtaining the statistical characteristics of thickness and elastic parameters for each sub-layer, a geostatistical algorithm is employed to generate numerous pseudo-wells that conform to actual sedimentary laws. Subsequently, the PINN is pre-trained using substantial samples, encompassing both pseudo and real well logging data, to integrate geological structural prior information into its network parameters. Lastly, PINN served as a specially designed prior constraint for the inversion network, allowing its geological information to effectively guide the inversion process via backpropagation. This inversion method is applied to both synthetic and field examples, and in comparison to conventional inversion algorithms, it demonstrated superior accuracy in blind well verification.
MoreTranslated text
Key words
Neural networks,Training,Geology,Reservoirs,Convolutional neural networks,Mathematical models,Synthetic data,Artificial neural networks (ANNs),prior information,statistical distribution
AI Read Science
Must-Reading Tree
Example
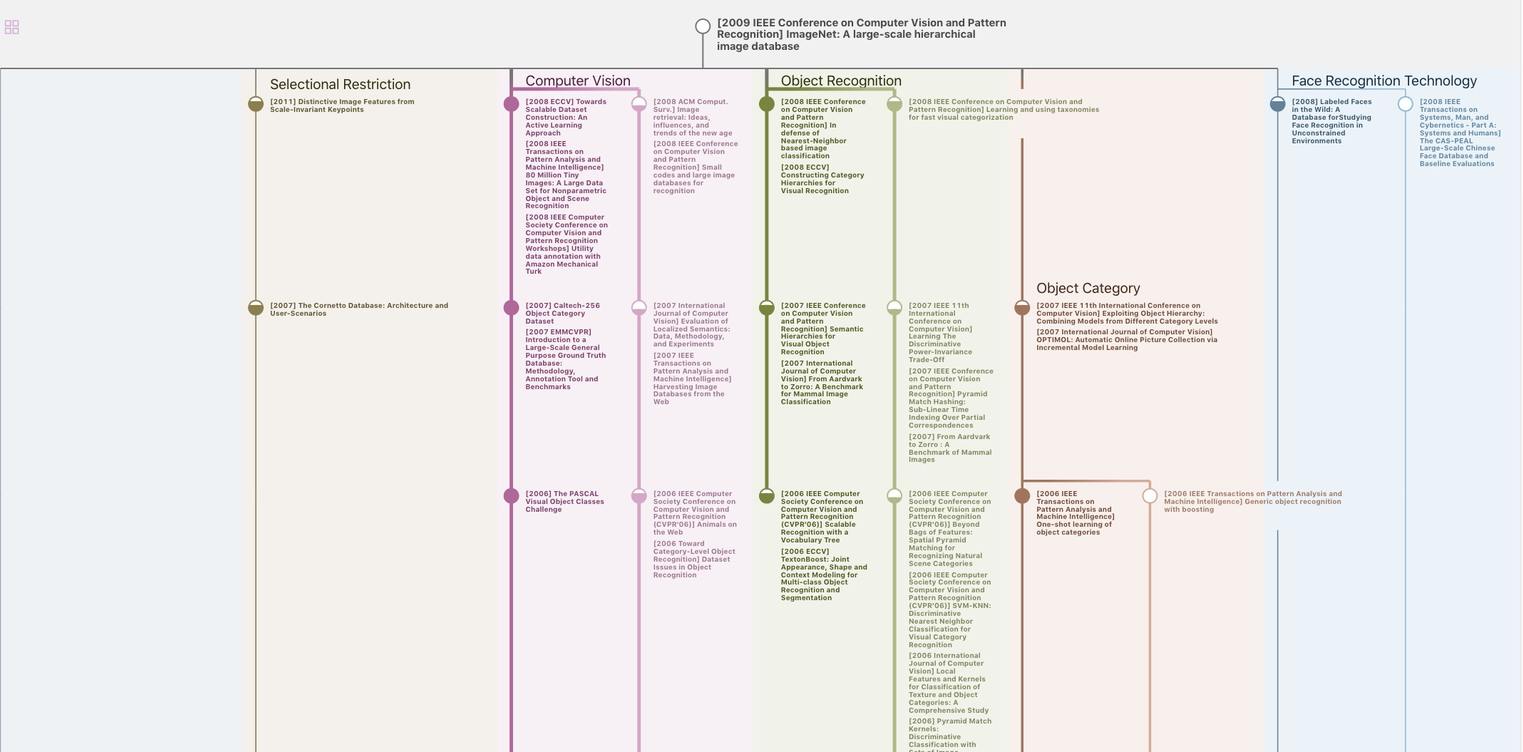
Generate MRT to find the research sequence of this paper
Chat Paper
Summary is being generated by the instructions you defined