Point Cloud Models Improve Visual Robustness in Robotic Learners
CoRR(2024)
摘要
Visual control policies can encounter significant performance degradation
when visual conditions like lighting or camera position differ from those seen
during training – often exhibiting sharp declines in capability even for minor
differences. In this work, we examine robustness to a suite of these types of
visual changes for RGB-D and point cloud based visual control policies. To
perform these experiments on both model-free and model-based reinforcement
learners, we introduce a novel Point Cloud World Model (PCWM) and point cloud
based control policies. Our experiments show that policies that explicitly
encode point clouds are significantly more robust than their RGB-D
counterparts. Further, we find our proposed PCWM significantly outperforms
prior works in terms of sample efficiency during training. Taken together,
these results suggest reasoning about the 3D scene through point clouds can
improve performance, reduce learning time, and increase robustness for robotic
learners. Project Webpage: https://pvskand.github.io/projects/PCWM
更多查看译文
AI 理解论文
溯源树
样例
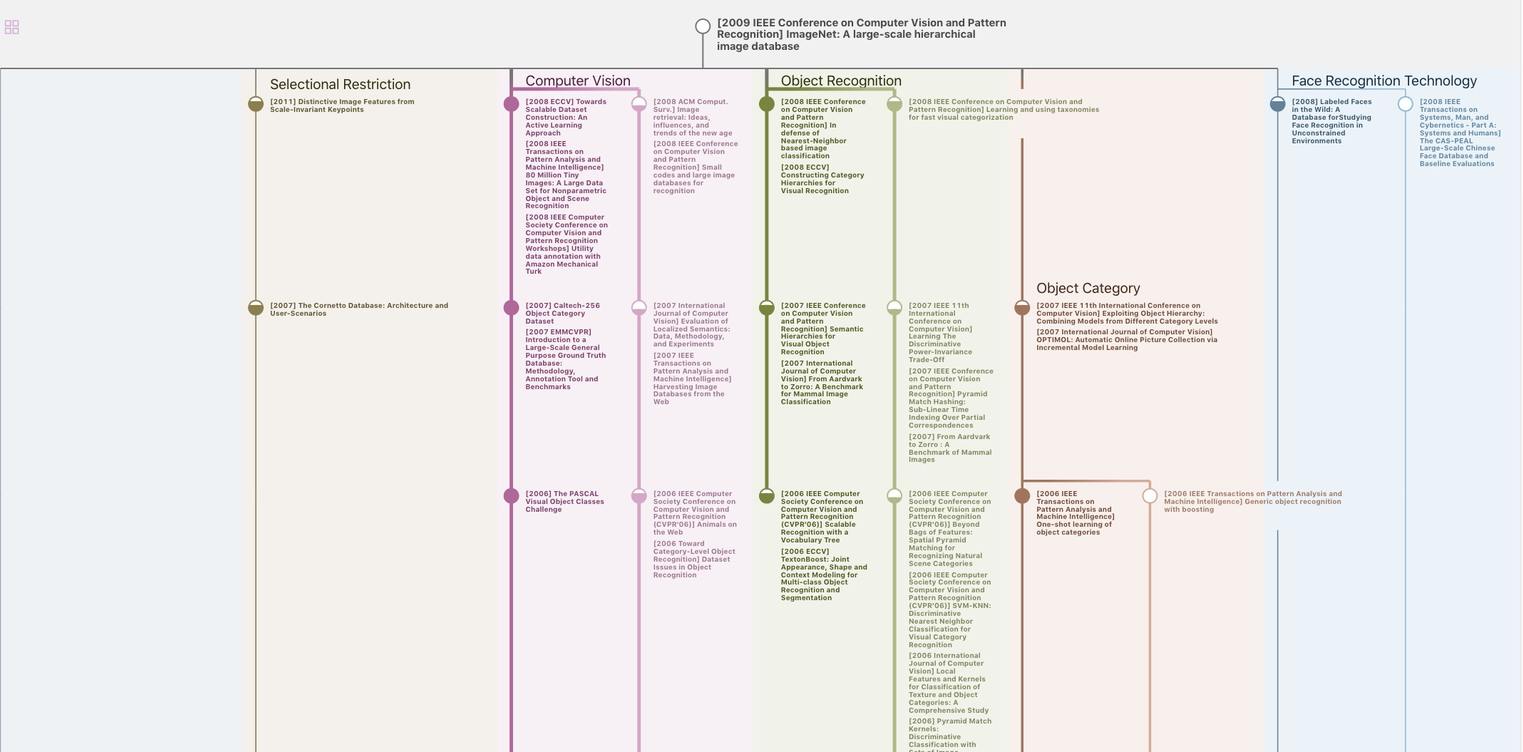
生成溯源树,研究论文发展脉络
Chat Paper
正在生成论文摘要