Non-convex Pose Graph Optimization in SLAM via Proximal Linearized Riemannian ADMM
CoRR(2024)
Abstract
Pose graph optimization (PGO) is a well-known technique for solving the pose-based simultaneous localization and mapping (SLAM) problem. In this paper, we represent the rotation and translation by a unit quaternion and a three-dimensional vector, and propose a new PGO model based on the von Mises-Fisher distribution. The constraints derived from the unit quaternions are spherical manifolds, and the projection onto the constraints can be calculated by normalization. Then a proximal linearized Riemannian alternating direction method of multipliers (PieADMM) is developed to solve the proposed model, which not only has low memory requirements, but also can update the poses in parallel. Furthermore, we establish the iteration complexity of O(1/ϵ^2) of PieADMM for finding an ϵ-stationary solution of our model. The efficiency of our proposed algorithm is demonstrated by numerical experiments on two synthetic and four 3D SLAM benchmark datasets.
MoreTranslated text
AI Read Science
Must-Reading Tree
Example
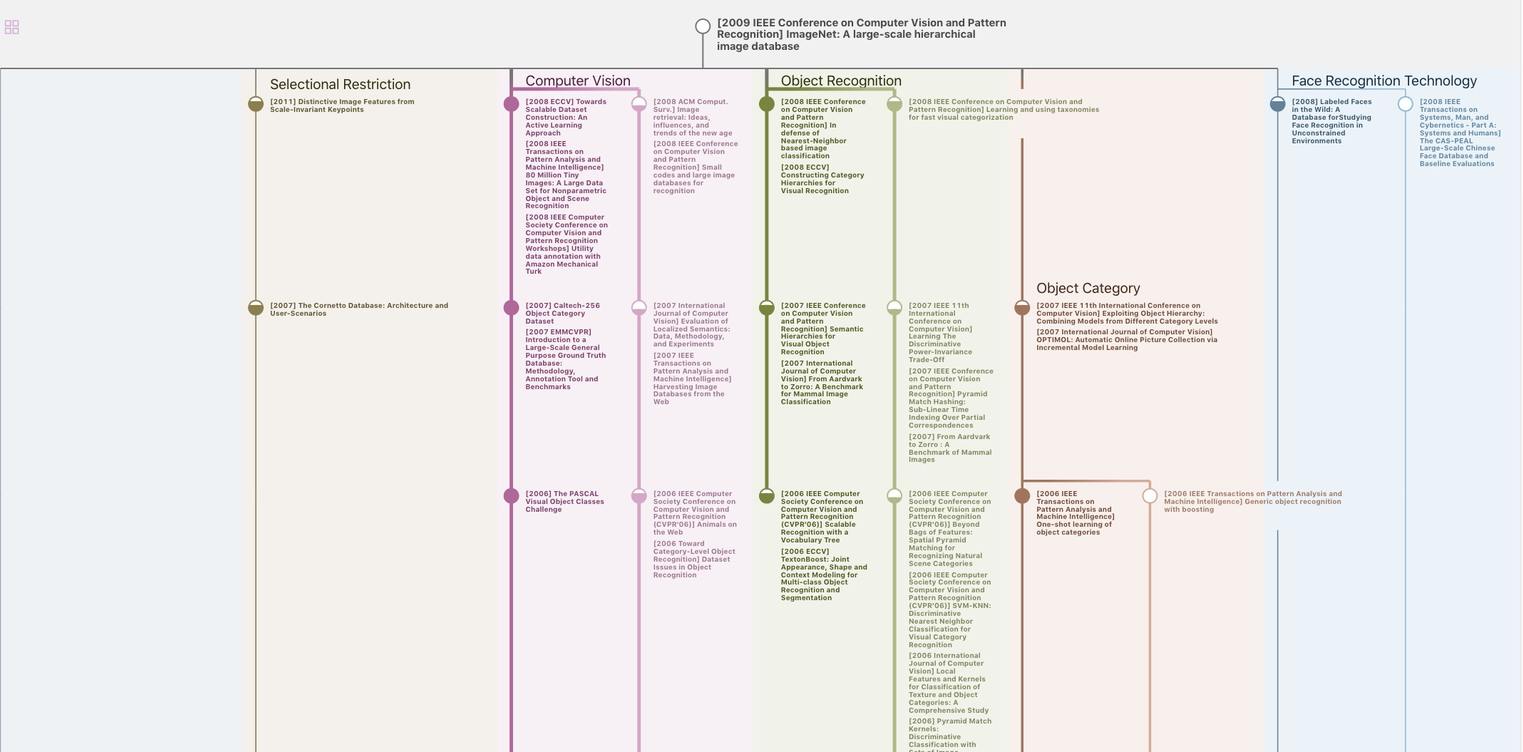
Generate MRT to find the research sequence of this paper
Chat Paper
Summary is being generated by the instructions you defined