S^2Mamba: A Spatial-spectral State Space Model for Hyperspectral Image Classification
arxiv(2024)
摘要
Land cover analysis using hyperspectral images (HSI) remains an open problem
due to their low spatial resolution and complex spectral information. Recent
studies are primarily dedicated to designing Transformer-based architectures
for spatial-spectral long-range dependencies modeling, which is computationally
expensive with quadratic complexity. Selective structured state space model
(Mamba), which is efficient for modeling long-range dependencies with linear
complexity, has recently shown promising progress. However, its potential in
hyperspectral image processing that requires handling numerous spectral bands
has not yet been explored. In this paper, we innovatively propose S^2Mamba, a
spatial-spectral state space model for hyperspectral image classification, to
excavate spatial-spectral contextual features, resulting in more efficient and
accurate land cover analysis. In S^2Mamba, two selective structured state
space models through different dimensions are designed for feature extraction,
one for spatial, and the other for spectral, along with a spatial-spectral
mixture gate for optimal fusion. More specifically, S^2Mamba first captures
spatial contextual relations by interacting each pixel with its adjacent
through a Patch Cross Scanning module and then explores semantic information
from continuous spectral bands through a Bi-directional Spectral Scanning
module. Considering the distinct expertise of the two attributes in homogenous
and complicated texture scenes, we realize the Spatial-spectral Mixture Gate by
a group of learnable matrices, allowing for the adaptive incorporation of
representations learned across different dimensions. Extensive experiments
conducted on HSI classification benchmarks demonstrate the superiority and
prospect of S^2Mamba. The code will be available at:
https://github.com/PURE-melo/S2Mamba.
更多查看译文
AI 理解论文
溯源树
样例
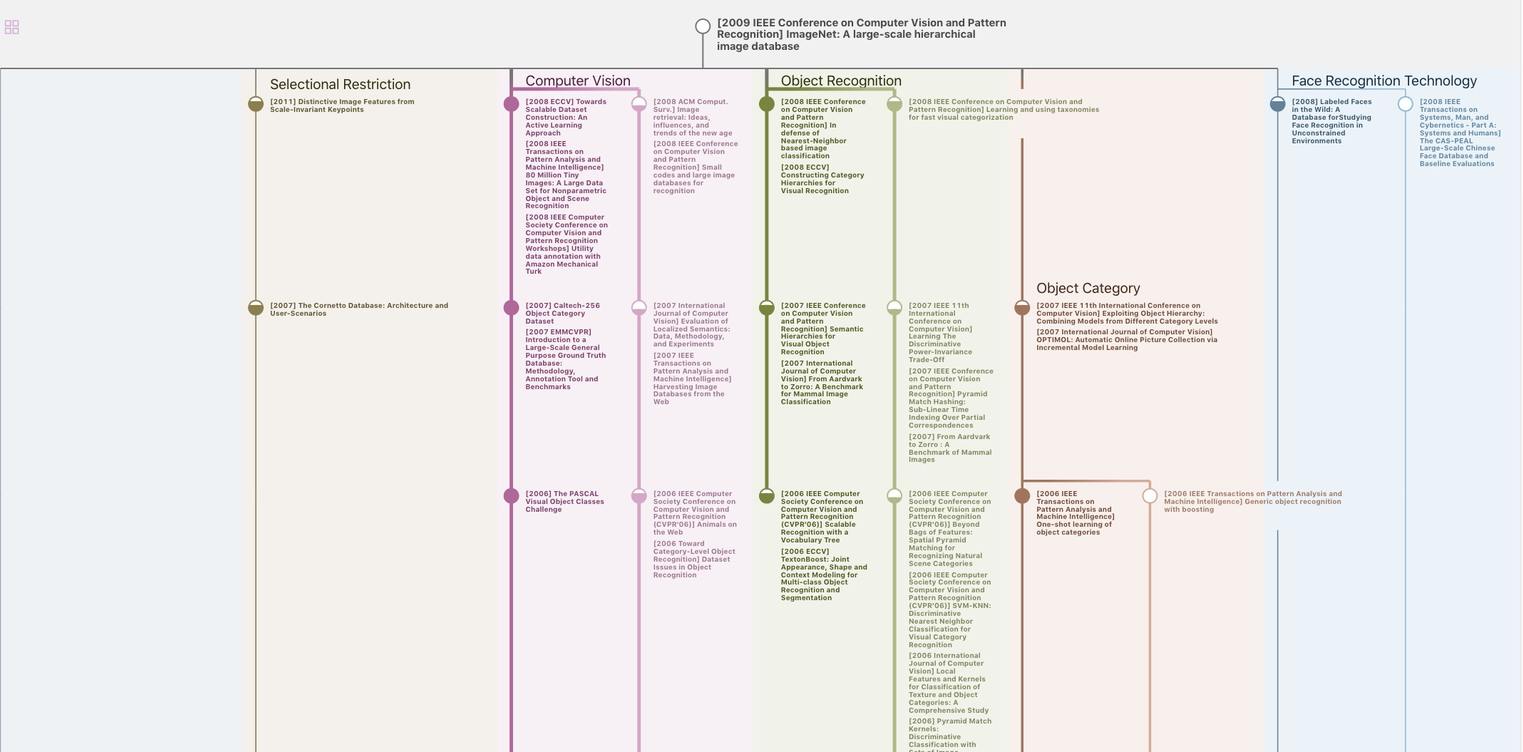
生成溯源树,研究论文发展脉络
Chat Paper
正在生成论文摘要