XGSwap: eXtreme Gradient boosting Swap for Routing in NISQ Devices
arxiv(2024)
摘要
In the current landscape of noisy intermediate-scale quantum (NISQ)
computing, the inherent noise presents significant challenges to achieving
high-fidelity long-range entanglement. Furthermore, this challenge is amplified
by the limited connectivity of current superconducting devices, necessitating
state permutations to establish long-distance entanglement. Traditionally,
graph methods are used to satisfy the coupling constraints of a given
architecture by routing states along the shortest undirected path between
qubits. In this work, we introduce a gradient boosting machine learning model
to predict the fidelity of alternative–potentially longer–routing paths to
improve fidelity. This model was trained on 4050 random CNOT gates ranging in
length from 2 to 100+ qubits. The experiments were all executed on ibm_quebec,
a 127-qubit IBM Quantum System One. Through more than 200+ tests run on actual
hardware, our model successfully identified higher fidelity paths in
approximately 23
更多查看译文
AI 理解论文
溯源树
样例
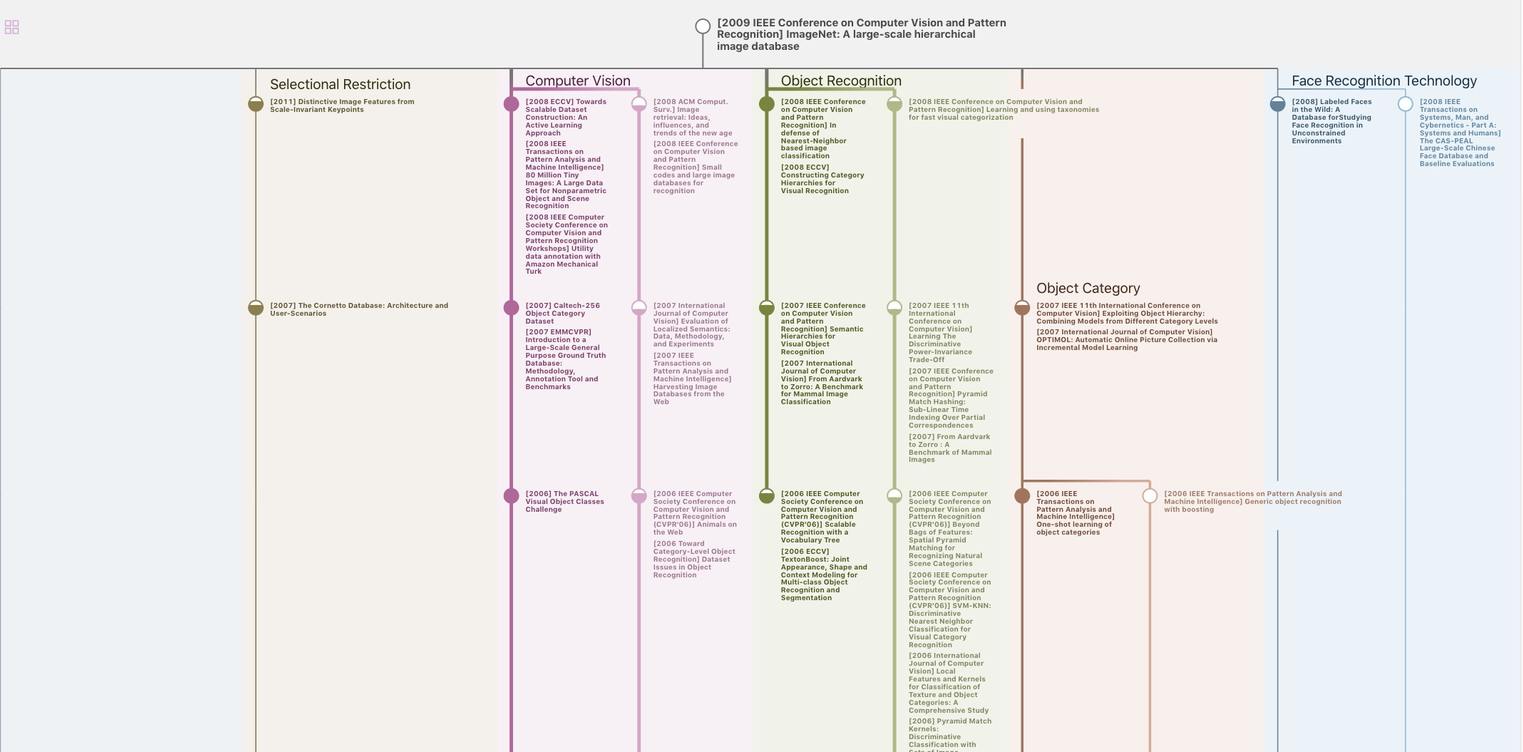
生成溯源树,研究论文发展脉络
Chat Paper
正在生成论文摘要