Localization Through Particle Filter Powered Neural Network Estimated Monocular Camera Poses
Fourth International Conference on Computer Vision and Pattern Analysis (ICCPA 2024)(2024)
AI 理解论文
溯源树
样例
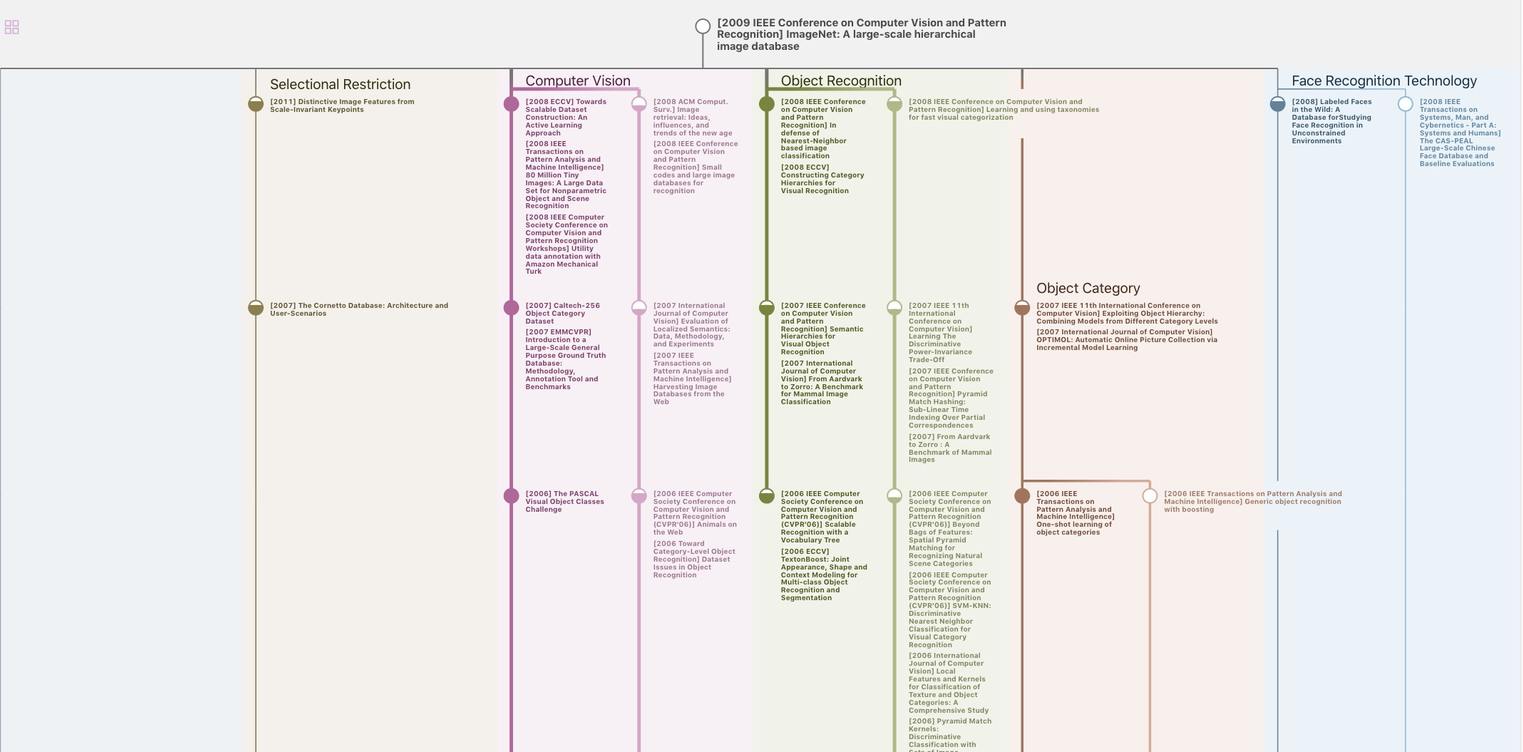
生成溯源树,研究论文发展脉络
Chat Paper
正在生成论文摘要
Fourth International Conference on Computer Vision and Pattern Analysis (ICCPA 2024)(2024)