Hyperspectral Target Detection-Based 2D-3D Parallel Convolutional Neural Networks for Hyperspectral Image Classification
IEEE Journal of Selected Topics in Applied Earth Observations and Remote Sensing(2024)
摘要
This paper presents a novel hyperspectral target detection based 2D-3D parallel convolutional neural network (HTD 2D-3D-PCNN) model, which integrates the hyperspectral target detection (HTD) technique to achieve outstanding performance in hyperspectral image classification (HSIC). The proposed model effectively leverages both spectral and spatial information through a dual-branch architecture in hyperspectral imaging (HSI). In the first branch, HTD is utilized to enhance the spectral features of targets of interest, while suppressing background. The resulting enhanced image is then inputted to a 2D-CNN, augmented with an additional deconvolution layer to highlight spatial characteristics. Concurrently, the second branch employs dimensionality reduction via PCA, and a 3D-CNN is employed to capture both spectral and spatial attributes. Subsequently, the feature maps from both CNNs are combined and processed through fully connected layers for classification. To validate the effectiveness of the proposed HTD-2D-3D-PCNN, extensive experiments are conducted on five widely used hyperspectral public datasets (Indian Pines, Pavia University, Salinas Scene, Kennedy Space Center, and Botswana) with a consistent training sample ratio of either 10% or 5%. The results show that HTD-2D-3D-PCNN can achieve overall accuracy values of 98.41%, 99.85%, 99.92%, 99.82%, and 98.82% for the respective datasets, surpassing the performance of recent methodologies.
更多查看译文
关键词
Convolutional neural networks (CNN),Hyperspectral image classification (HSIC),Hyperspectral target detection (HTD)
AI 理解论文
溯源树
样例
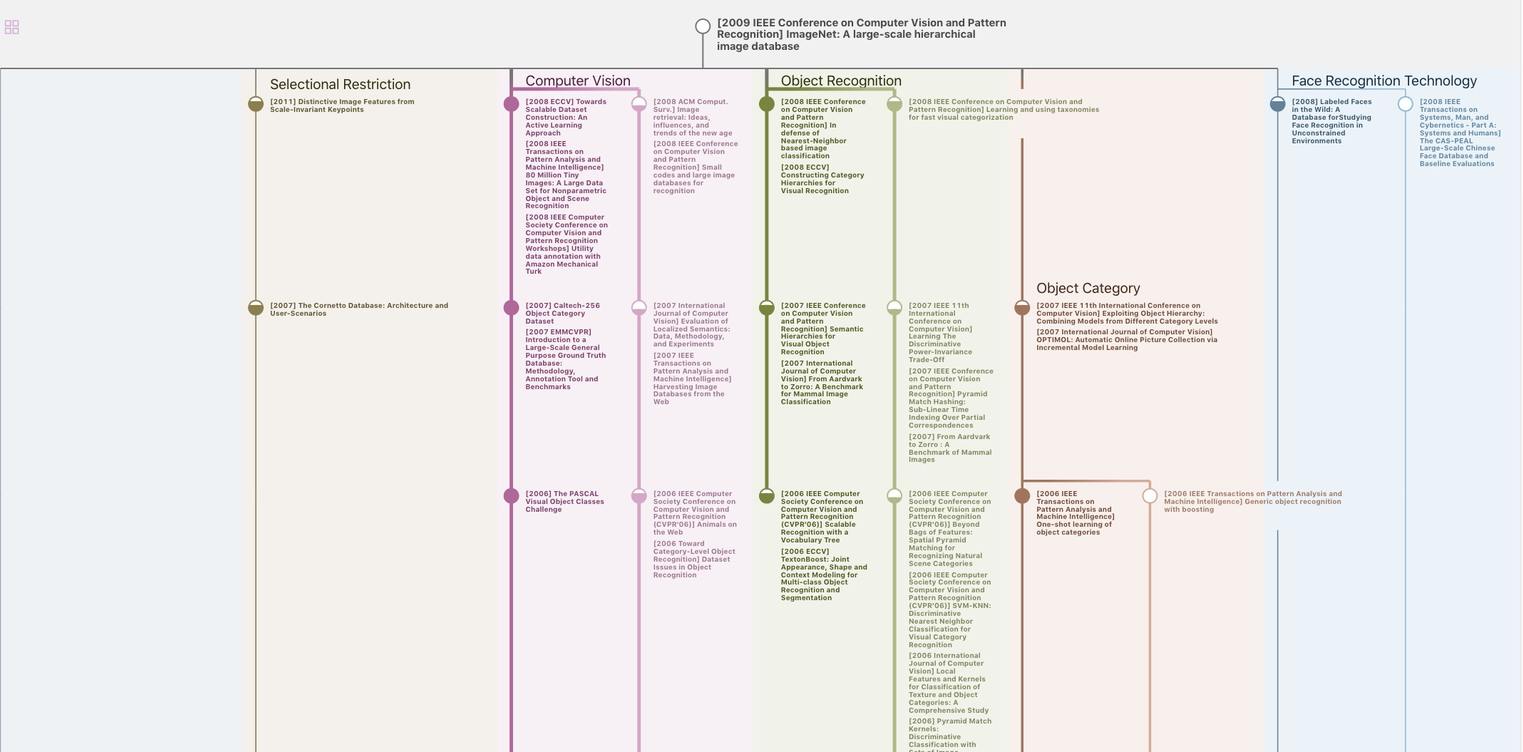
生成溯源树,研究论文发展脉络
Chat Paper
正在生成论文摘要