Road traffic estimation and distribution-based route selection
SSRN Electronic Journal(2022)
Abstract
In route selection problems, the driver's personal preferences will determine whether she prefers a route with a travel time that has a relatively low mean and high variance over one that has relatively high mean and low variance. In practice, however, such risk aversion issues are often ignored, in that a route is selected based on a single-criterion Dijkstra-type algorithm. In addition, the routing decision typically does not take into account the uncertainty in the estimates of the travel time's mean and variance. This paper aims at resolving both issues by setting up a framework for travel time estimation. In our framework, the underlying road network is represented as a graph. Each edge is subdivided into multiple smaller pieces, so as to naturally model the statistical similarity between road pieces that are spatially nearby. Relying on a Bayesian approach, we construct an estimator for the joint per-edge travel time distribution, thus also providing us with an uncertainty quantification of our estimates. Our machinery relies on establishing limit theorems, making the resulting estimation procedure robust in the sense that it effectively does not assume any distributional properties. We present an extensive set of numerical experiments that demonstrate the validity of the estimation procedure and the use of the distributional estimates in the context of data-driven route selection.
MoreTranslated text
Key words
route,estimation,selection,distribution-based
AI Read Science
Must-Reading Tree
Example
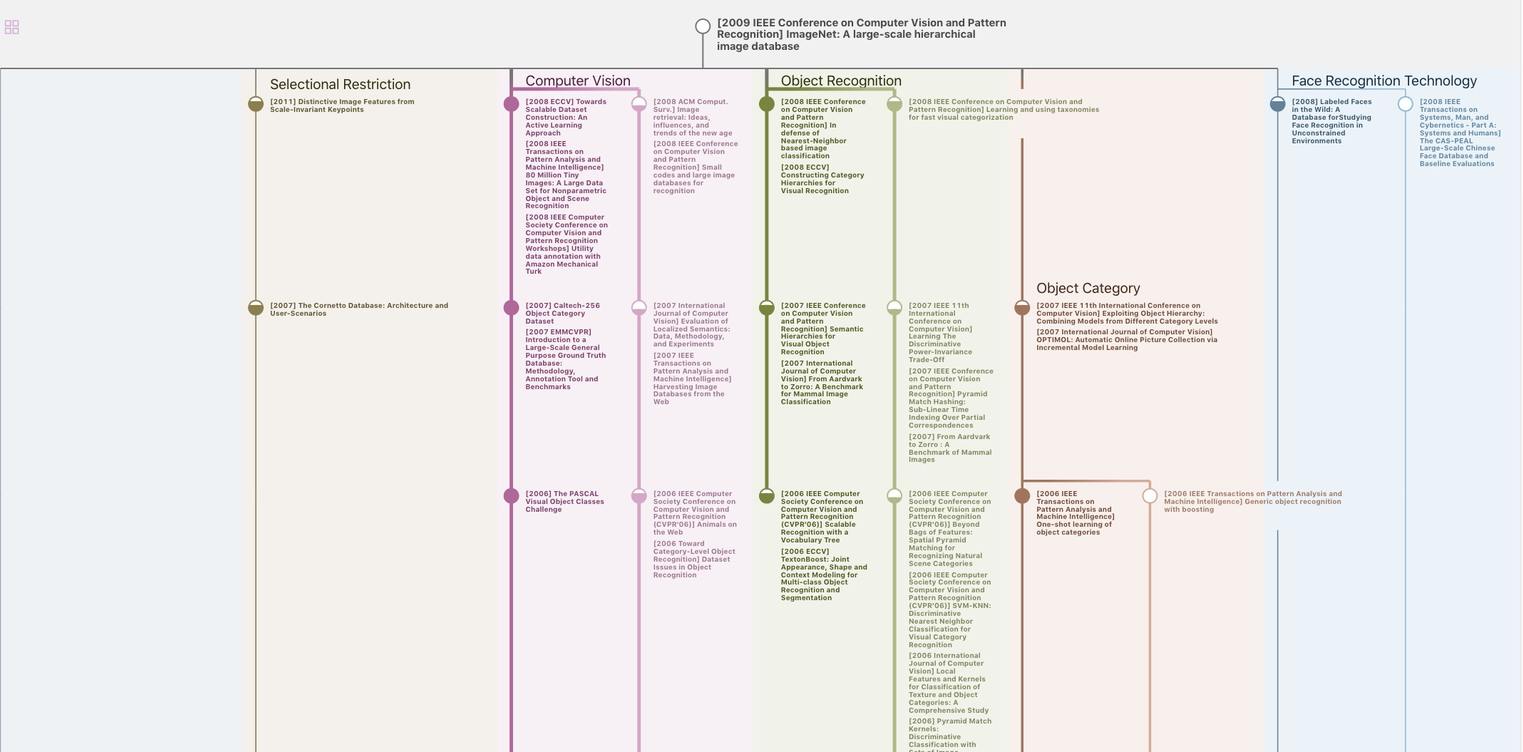
Generate MRT to find the research sequence of this paper
Chat Paper
Summary is being generated by the instructions you defined