Channel reflection: Knowledge-driven data augmentation for EEG-based brain-computer interfaces
NEURAL NETWORKS(2024)
Abstract
A brain-computer interface (BCI) enables direct communication between the human brain and external devices. Electroencephalography (EEG) based BCIs are currently the most popular for able-bodied users. To increase user-friendliness, usually a small amount of user-specific EEG data are used for calibration, which may not be enough to develop a pure data -driven decoding model. To cope with this typical calibration data shortage challenge in EEG-based BCIs, this paper proposes a parameter -free channel reflection (CR) data augmentation approach that incorporates prior knowledge on the channel distributions of different BCI paradigms in data augmentation. Experiments on eight public EEG datasets across four different BCI paradigms (motor imagery, steady -state visual evoked potential, P300, and seizure classifications) using different decoding algorithms demonstrated that: (1) CR is effective, i.e., it can noticeably improve the classification accuracy; (2) CR is robust, i.e., it consistently outperforms existing data augmentation approaches in the literature; and, (3) CR is flexible, i.e., it can be combined with other data augmentation approaches to further improve the performance. We suggest that data augmentation approaches like CR should be an essential step in EEG-based BCIs. Our code is available online.
MoreTranslated text
Key words
Brain-computer interface,Electroencephalogram,Informed machine learning,Integration of data and knowledge,Data augmentation
AI Read Science
Must-Reading Tree
Example
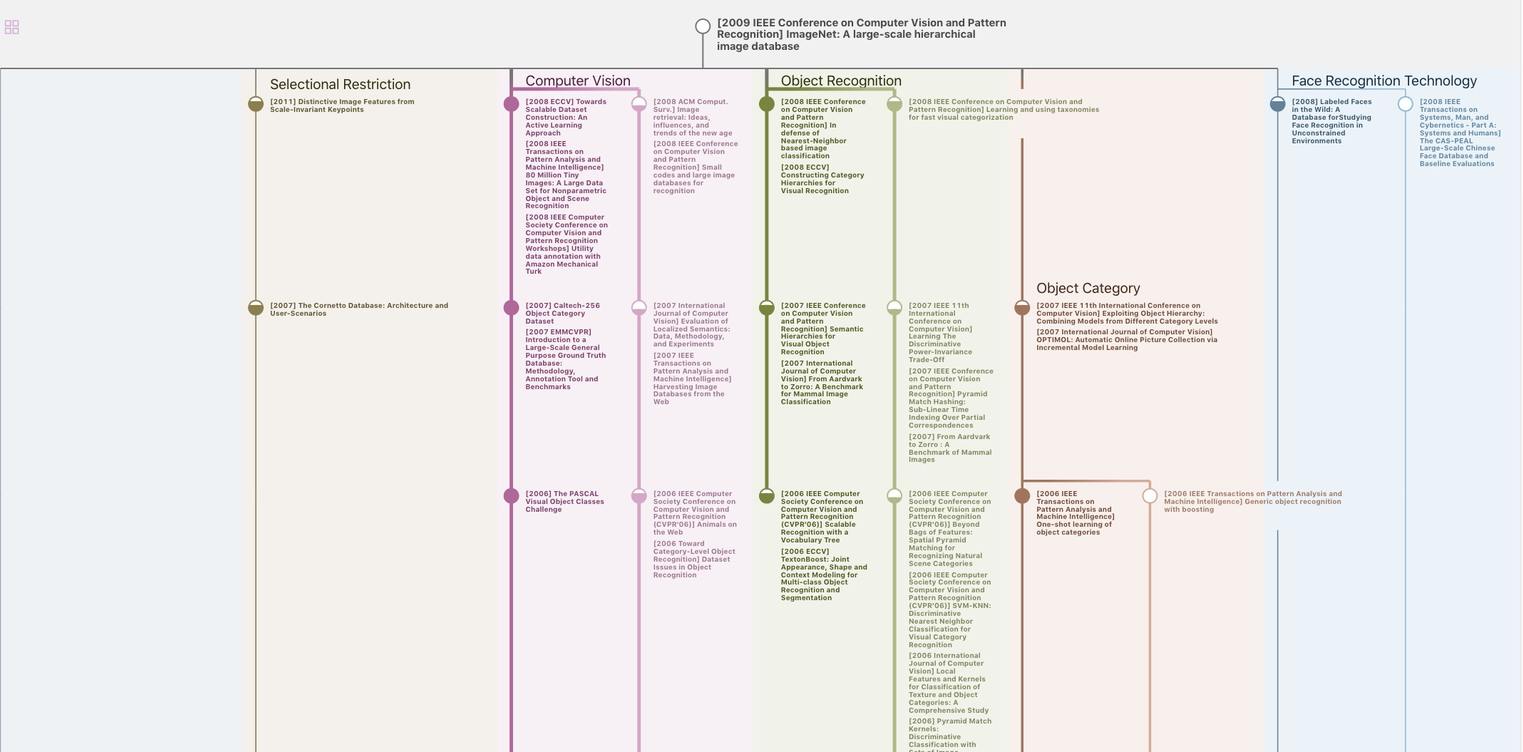
Generate MRT to find the research sequence of this paper
Chat Paper
Summary is being generated by the instructions you defined